Mapping Urban Floods via Spectral Indices and Machine Learning Algorithms
SUSTAINABILITY(2024)
Abstract
Throughout history, natural disasters have caused severe damage to people and properties worldwide. Flooding is one of the most disastrous types of natural disasters. A key feature of flood assessment has been making use of the information derived from remote-sensing imagery from optical sensors on satellites using spectral indices. Here, a study was conducted about a recent spectral index, the Normalised Difference Inundation Index, and a new ensemble spectral index, the Concatenated Normalised Difference Water Index, and two mature spectral indices: Normalised Difference Water Index and the differential Normalised Difference Water Index with four different machine learning algorithms: Decision Tree, Random Forest, Naive Bayes, and K-Nearest Neighbours applied to the PlanetScope satellite imagery about the Brisbane February 2022 flood which is in urban environment. Statistical analysis was applied to evaluate the results. Overall, the four algorithms provided no significant difference in terms of accuracy and F1 score. However, there were significant differences when some variations in the indices and the algorithms were combined. This research provides a validation of existing measures to identify floods in an urban environment that can help to improve sustainable development.
MoreTranslated text
Key words
urban floods,flood mapping,disaster management,machine learning,NDWI,NDII,dNDWI,CNDWI,PlanetScope,decision tree,random forest,naive bayes,k-nearest neighbours
AI Read Science
Must-Reading Tree
Example
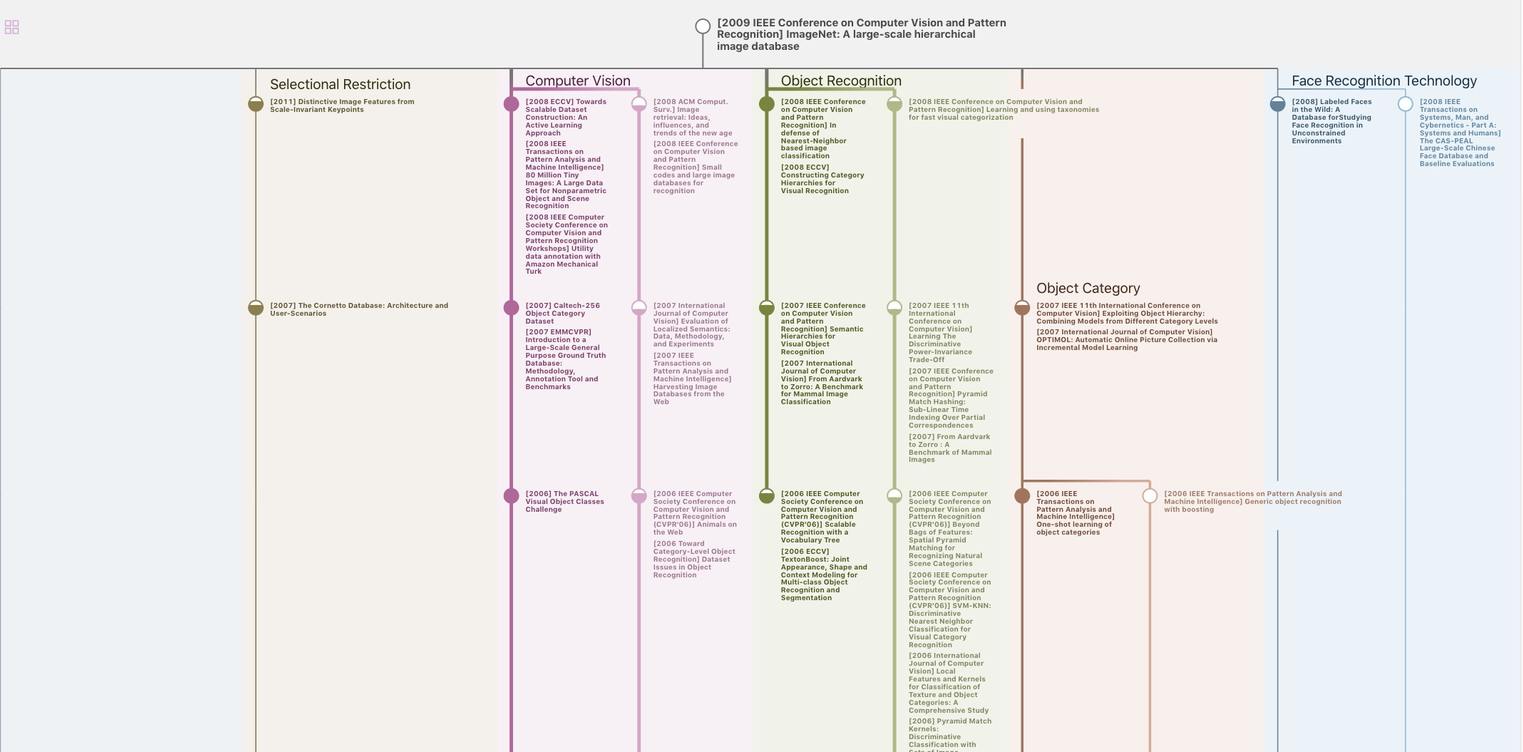
Generate MRT to find the research sequence of this paper
Chat Paper
Summary is being generated by the instructions you defined