A Multi-source Domain Adaption Approach to Minority Disk Failure Prediction
ALGORITHMS AND ARCHITECTURES FOR PARALLEL PROCESSING, ICA3PP 2023, PT II(2024)
摘要
Frequent happening of disk failures affects the reliability of the storage system, which can cause jittering of performance or even data loss of services and thus seriously threaten the quality of service. Although a host of machine (deep) learning-based disk failure prediction approaches have been proposed to prevent system breakdown due to unexpected disk failure, they are able to achieve high performance based on the assumption that the disk model has plenty of samples (especially failure samples). However, new disk models continuously appear in data centers with the evolution of disk manufacturing technology and the expansion of storage system capacity. Limited by the deploying time, these disk models have few failure samples and are called minority disks. The minority disks are widespread in large-scale data centers and contain amounts of disks while existing approaches cannot reach satisfying performance on such disks due to the lack of failure samples. What's worse, failure prediction models trained on other disk models cannot be directly applied to these minority disks either due to the commonly existing distribution shift among disk models. In this work, we propose DiskDA, a novel multi-source domain adaption-based solution that can fully utilize knowledge from other disk models to predict failures for minority disks having no failure samples. Our experimental results on real-world datasets show the superiority of DiskDA against previous approaches on minority disks with a few failure samples. What's more, DiskDA also shows its good adaptivity on minority disks having no failure samples, whereas previous works are unusable.
更多查看译文
关键词
Fault tolerance,Disk failure prediction,Domain adaption,Cloud computing
AI 理解论文
溯源树
样例
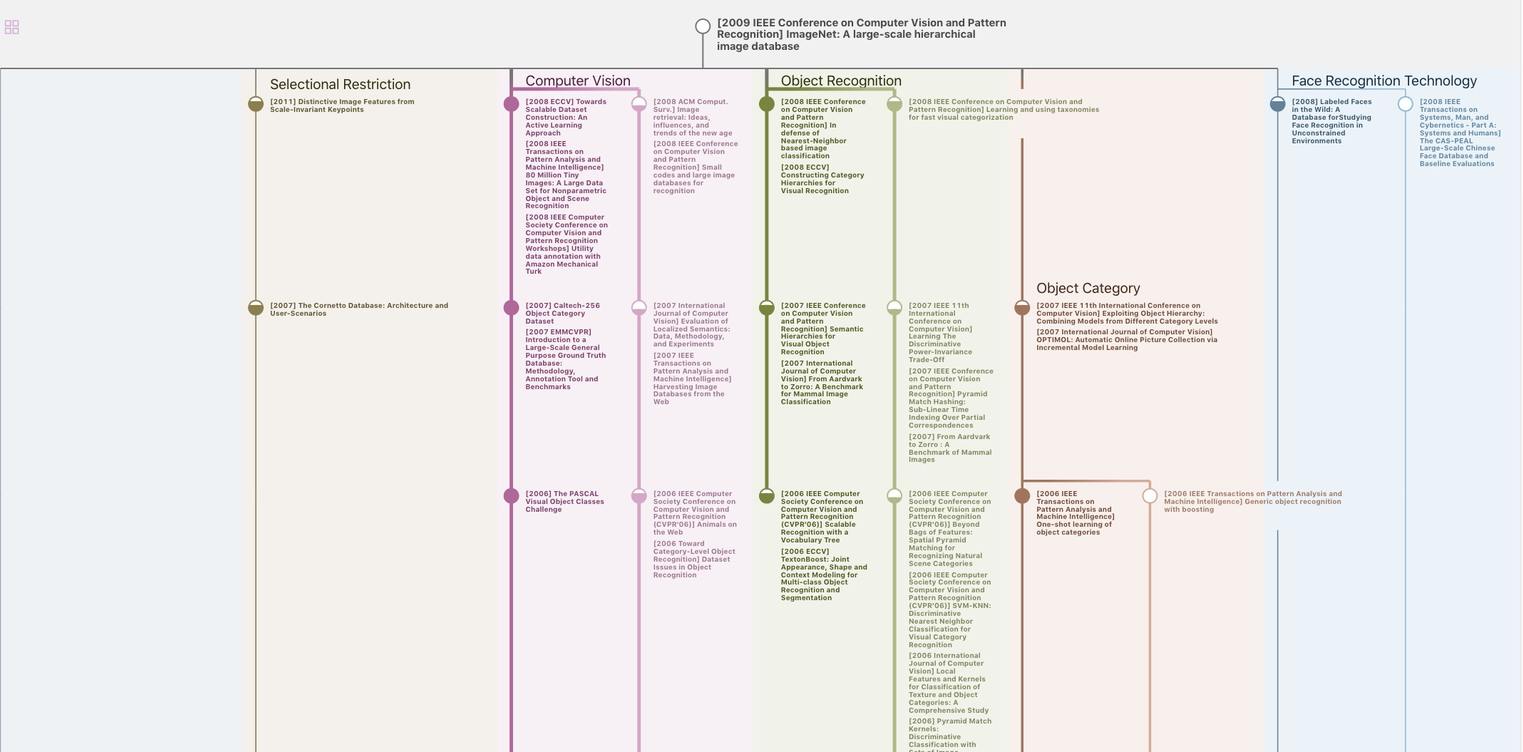
生成溯源树,研究论文发展脉络
Chat Paper
正在生成论文摘要