Frozen Feature Augmentation for Few-Shot Image Classification
CVPR 2024(2024)
Abstract
Training a linear classifier or lightweight model on top of pretrained vision
model outputs, so-called 'frozen features', leads to impressive performance on
a number of downstream few-shot tasks. Currently, frozen features are not
modified during training. On the other hand, when networks are trained directly
on images, data augmentation is a standard recipe that improves performance
with no substantial overhead. In this paper, we conduct an extensive pilot
study on few-shot image classification that explores applying data
augmentations in the frozen feature space, dubbed 'frozen feature augmentation
(FroFA)', covering twenty augmentations in total. Our study demonstrates that
adopting a deceptively simple pointwise FroFA, such as brightness, can improve
few-shot performance consistently across three network architectures, three
large pretraining datasets, and eight transfer datasets.
MoreTranslated text
AI Read Science
Must-Reading Tree
Example
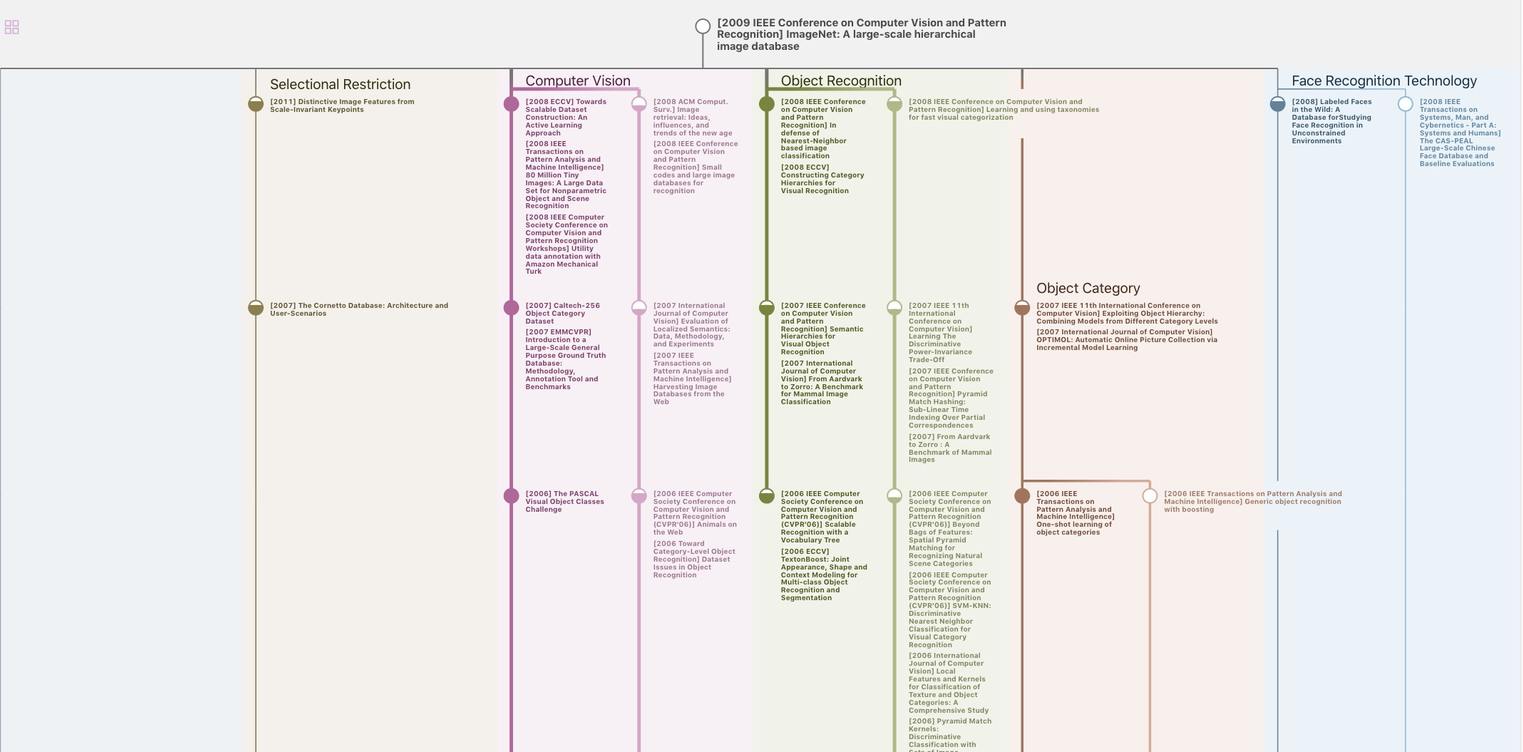
Generate MRT to find the research sequence of this paper
Chat Paper
Summary is being generated by the instructions you defined