Reconfigurable Robot Identification from Motion Data
CoRR(2024)
Abstract
Integrating Large Language Models (VLMs) and Vision-Language Models (VLMs)
with robotic systems enables robots to process and understand complex natural
language instructions and visual information. However, a fundamental challenge
remains: for robots to fully capitalize on these advancements, they must have a
deep understanding of their physical embodiment. The gap between AI models
cognitive capabilities and the understanding of physical embodiment leads to
the following question: Can a robot autonomously understand and adapt to its
physical form and functionalities through interaction with its environment?
This question underscores the transition towards developing self-modeling
robots without reliance on external sensory or pre-programmed knowledge about
their structure. Here, we propose a meta self modeling that can deduce robot
morphology through proprioception (the internal sense of position and
movement). Our study introduces a 12 DoF reconfigurable legged robot,
accompanied by a diverse dataset of 200k unique configurations, to
systematically investigate the relationship between robotic motion and robot
morphology. Utilizing a deep neural network model comprising a robot signature
encoder and a configuration decoder, we demonstrate the capability of our
system to accurately predict robot configurations from proprioceptive signals.
This research contributes to the field of robotic self-modeling, aiming to
enhance understanding of their physical embodiment and adaptability in real
world scenarios.
MoreTranslated text
AI Read Science
Must-Reading Tree
Example
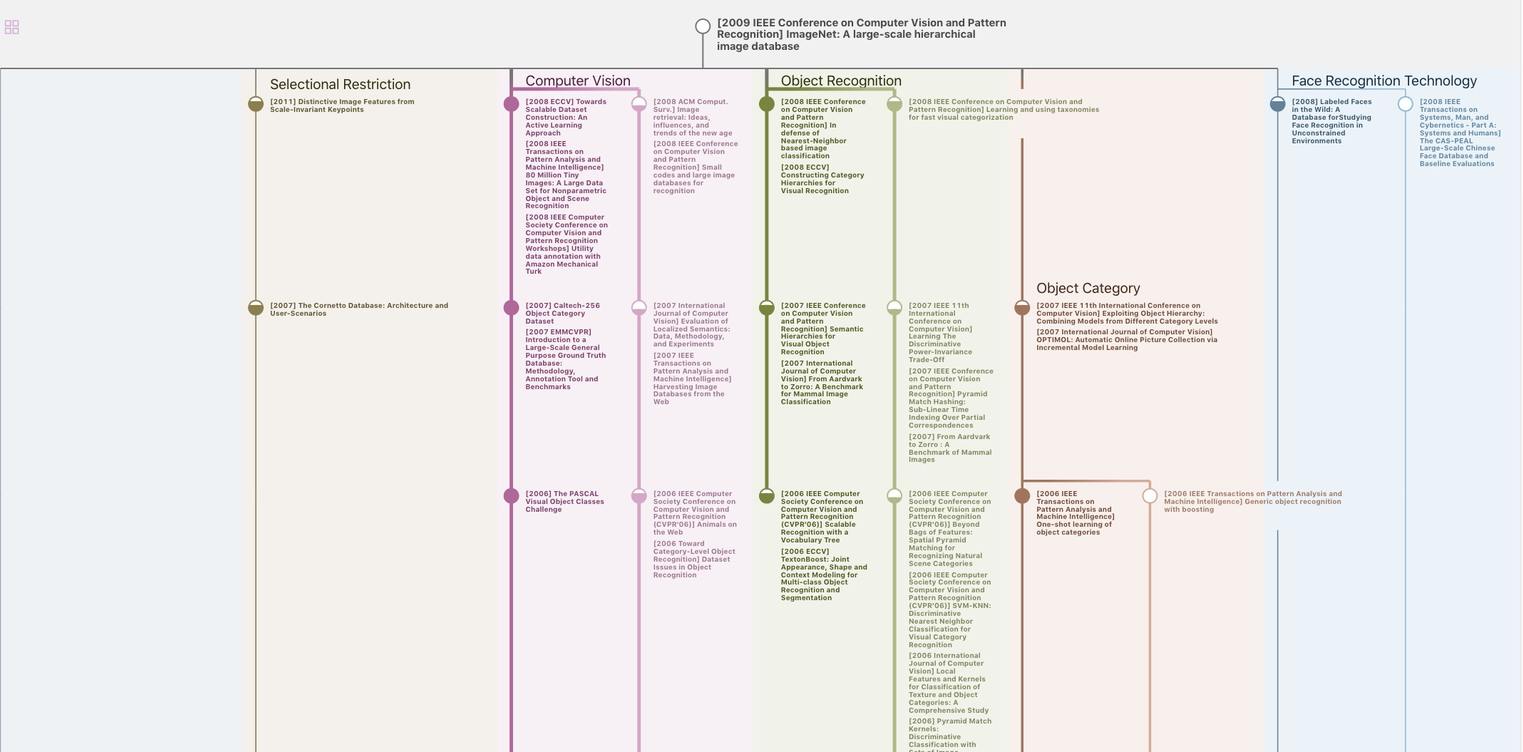
Generate MRT to find the research sequence of this paper
Chat Paper
Summary is being generated by the instructions you defined