Do Visual-Language Maps Capture Latent Semantics?
CoRR(2024)
Abstract
Visual-language models (VLMs) have recently been introduced in robotic
mapping by using the latent representations, i.e., embeddings, of the VLMs to
represent the natural language semantics in the map. The main benefit is moving
beyond a small set of human-created labels toward open-vocabulary scene
understanding. While there is anecdotal evidence that maps built this way
support downstream tasks, such as navigation, rigorous analysis of the quality
of the maps using these embeddings is lacking. We investigate two critical
properties of map quality: queryability and consistency. The evaluation of
queryability addresses the ability to retrieve information from the embeddings.
We investigate two aspects of consistency: intra-map consistency and inter-map
consistency. Intra-map consistency captures the ability of the embeddings to
represent abstract semantic classes, and inter-map consistency captures the
generalization properties of the representation. In this paper, we propose a
way to analyze the quality of maps created using VLMs, which forms an
open-source benchmark to be used when proposing new open-vocabulary map
representations. We demonstrate the benchmark by evaluating the maps created by
two state-of-the-art methods, VLMaps and OpenScene, using two encoders, LSeg
and OpenSeg, using real-world data from the Matterport3D data set. We find that
OpenScene outperforms VLMaps with both encoders, and LSeg outperforms OpenSeg
with both methods.
MoreTranslated text
AI Read Science
Must-Reading Tree
Example
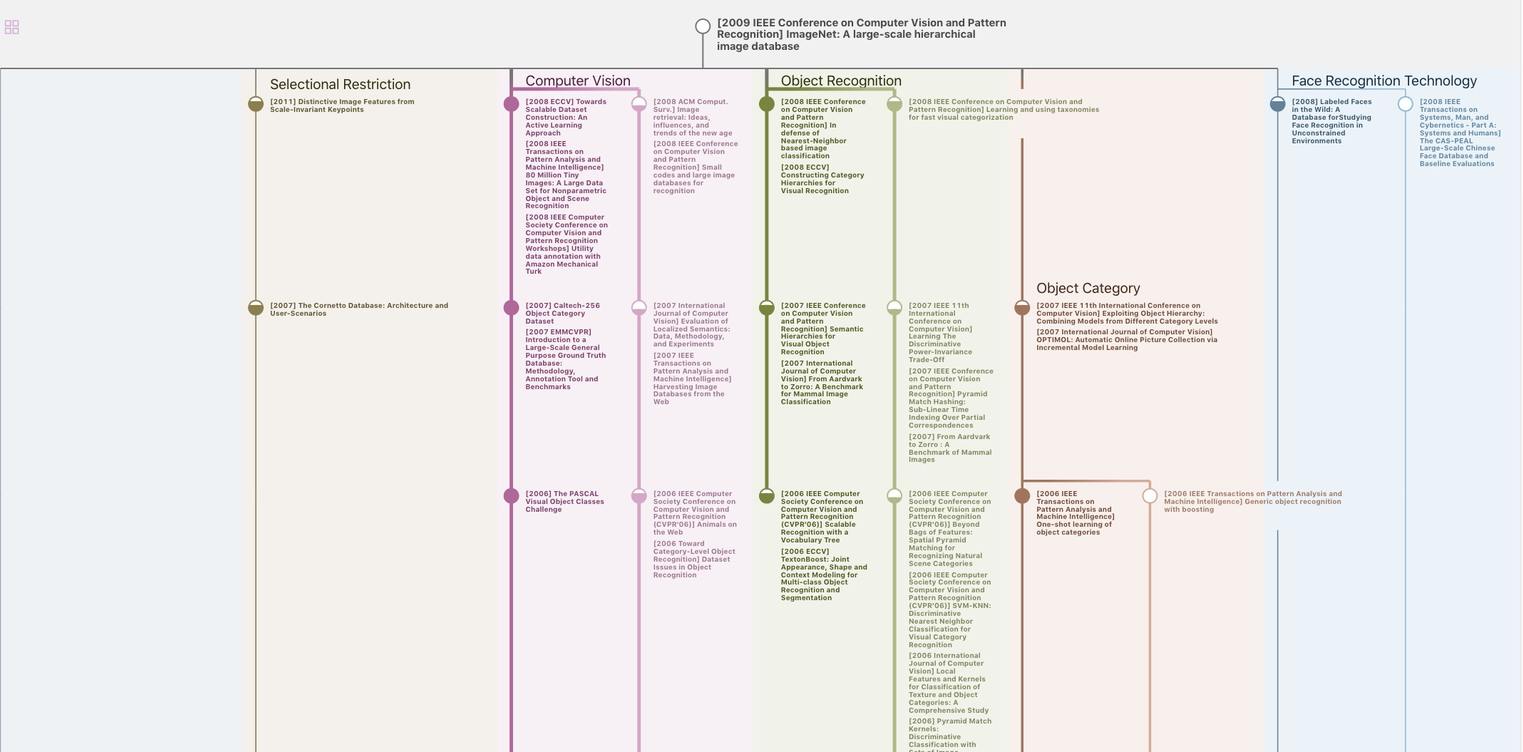
Generate MRT to find the research sequence of this paper
Chat Paper
Summary is being generated by the instructions you defined