A Structure-Preserving Kernel Method for Learning Hamiltonian Systems
CoRR(2024)
Abstract
A structure-preserving kernel ridge regression method is presented that
allows the recovery of potentially high-dimensional and nonlinear Hamiltonian
functions out of datasets made of noisy observations of Hamiltonian vector
fields. The method proposes a closed-form solution that yields excellent
numerical performances that surpass other techniques proposed in the literature
in this setup. From the methodological point of view, the paper extends kernel
regression methods to problems in which loss functions involving linear
functions of gradients are required and, in particular, a differential
reproducing property and a Representer Theorem are proved in this context. The
relation between the structure-preserving kernel estimator and the Gaussian
posterior mean estimator is analyzed. A full error analysis is conducted that
provides convergence rates using fixed and adaptive regularization parameters.
The good performance of the proposed estimator is illustrated with various
numerical experiments.
MoreTranslated text
AI Read Science
Must-Reading Tree
Example
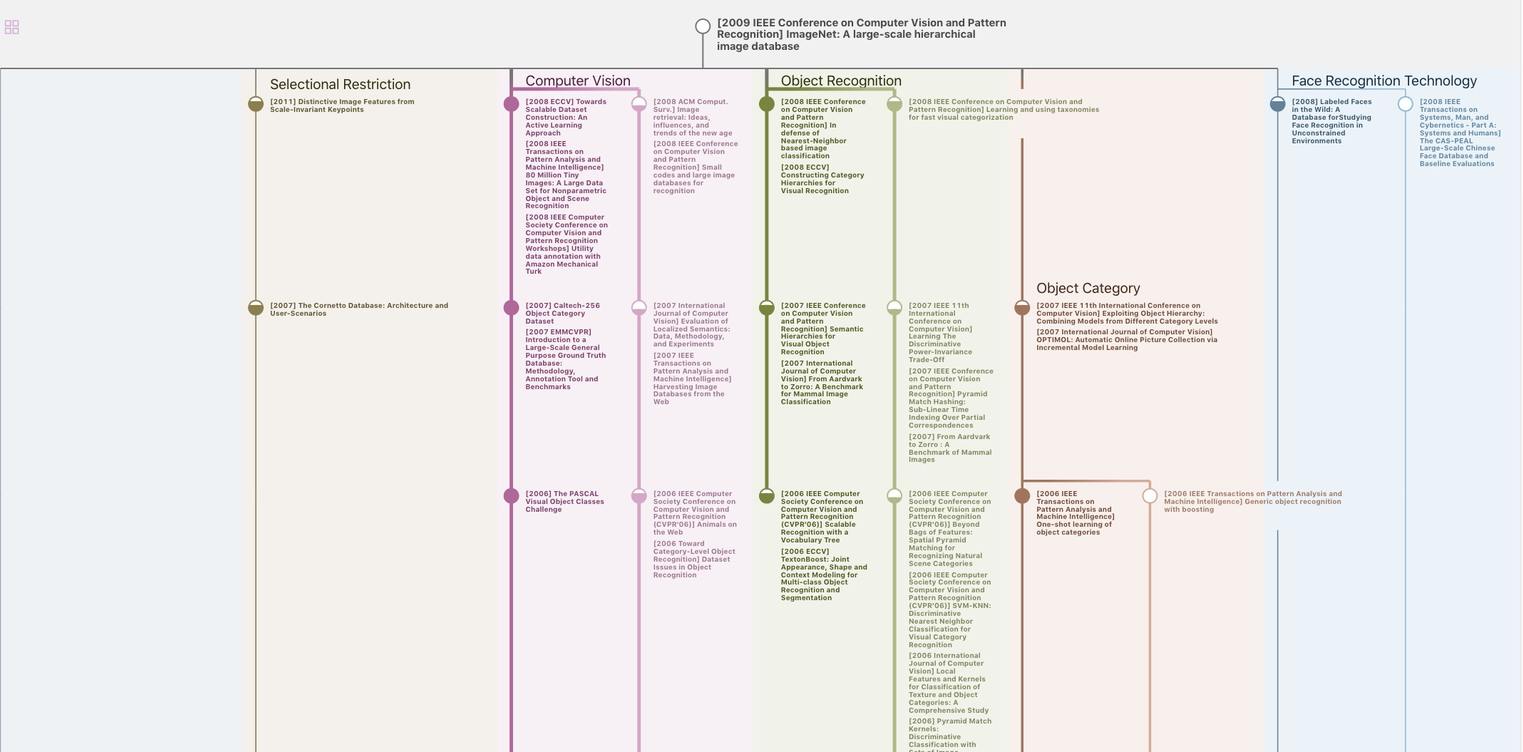
Generate MRT to find the research sequence of this paper
Chat Paper
Summary is being generated by the instructions you defined