Accurate and Data-Efficient Micro-XRD Phase Identification Using Multi-Task Learning: Application to Hydrothermal Fluids
CoRR(2024)
Abstract
Traditional analysis of highly distorted micro-X-ray diffraction (μ-XRD)
patterns from hydrothermal fluid environments is a time-consuming process,
often requiring substantial data preprocessing and labeled experimental data.
This study demonstrates the potential of deep learning with a multitask
learning (MTL) architecture to overcome these limitations. We trained MTL
models to identify phase information in μ-XRD patterns, minimizing the need
for labeled experimental data and masking preprocessing steps. Notably, MTL
models showed superior accuracy compared to binary classification CNNs.
Additionally, introducing a tailored cross-entropy loss function improved MTL
model performance. Most significantly, MTL models tuned to analyze raw and
unmasked XRD patterns achieved close performance to models analyzing
preprocessed data, with minimal accuracy differences. This work indicates that
advanced deep learning architectures like MTL can automate arduous data
handling tasks, streamline the analysis of distorted XRD patterns, and reduce
the reliance on labor-intensive experimental datasets.
MoreTranslated text
AI Read Science
Must-Reading Tree
Example
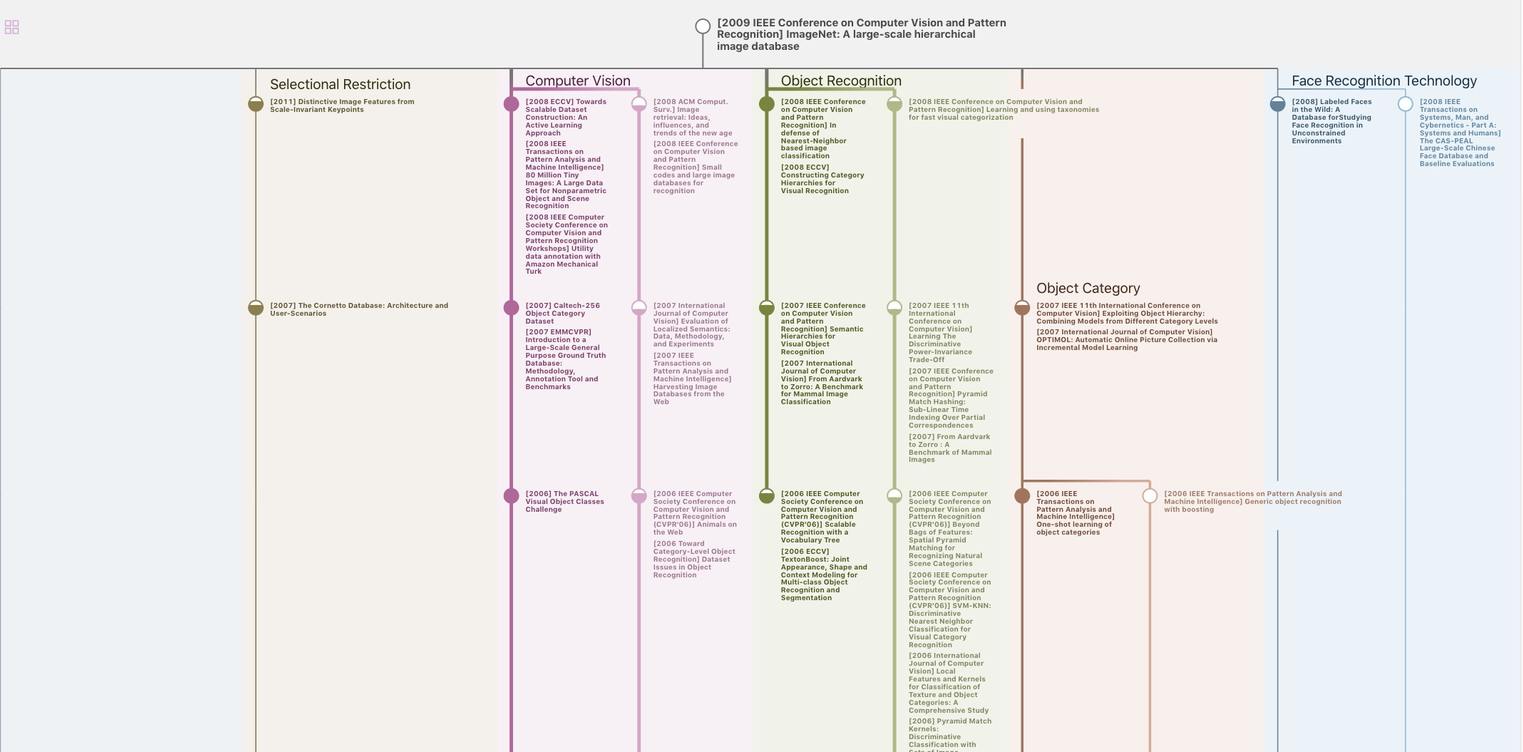
Generate MRT to find the research sequence of this paper
Chat Paper
Summary is being generated by the instructions you defined