LabelAId: Just-in-time AI Interventions for Improving Human Labeling Quality and Domain Knowledge in Crowdsourcing Systems
arxiv(2024)
摘要
Crowdsourcing platforms have transformed distributed problem-solving, yet
quality control remains a persistent challenge. Traditional quality control
measures, such as prescreening workers and refining instructions, often focus
solely on optimizing economic output. This paper explores just-in-time AI
interventions to enhance both labeling quality and domain-specific knowledge
among crowdworkers. We introduce LabelAId, an advanced inference model
combining Programmatic Weak Supervision (PWS) with FT-Transformers to infer
label correctness based on user behavior and domain knowledge. Our technical
evaluation shows that our LabelAId pipeline consistently outperforms
state-of-the-art ML baselines, improving mistake inference accuracy by 36.7
with 50 downstream samples. We then implemented LabelAId into Project Sidewalk,
an open-source crowdsourcing platform for urban accessibility. A
between-subjects study with 34 participants demonstrates that LabelAId
significantly enhances label precision without compromising efficiency while
also increasing labeler confidence. We discuss LabelAId's success factors,
limitations, and its generalizability to other crowdsourced science domains.
更多查看译文
AI 理解论文
溯源树
样例
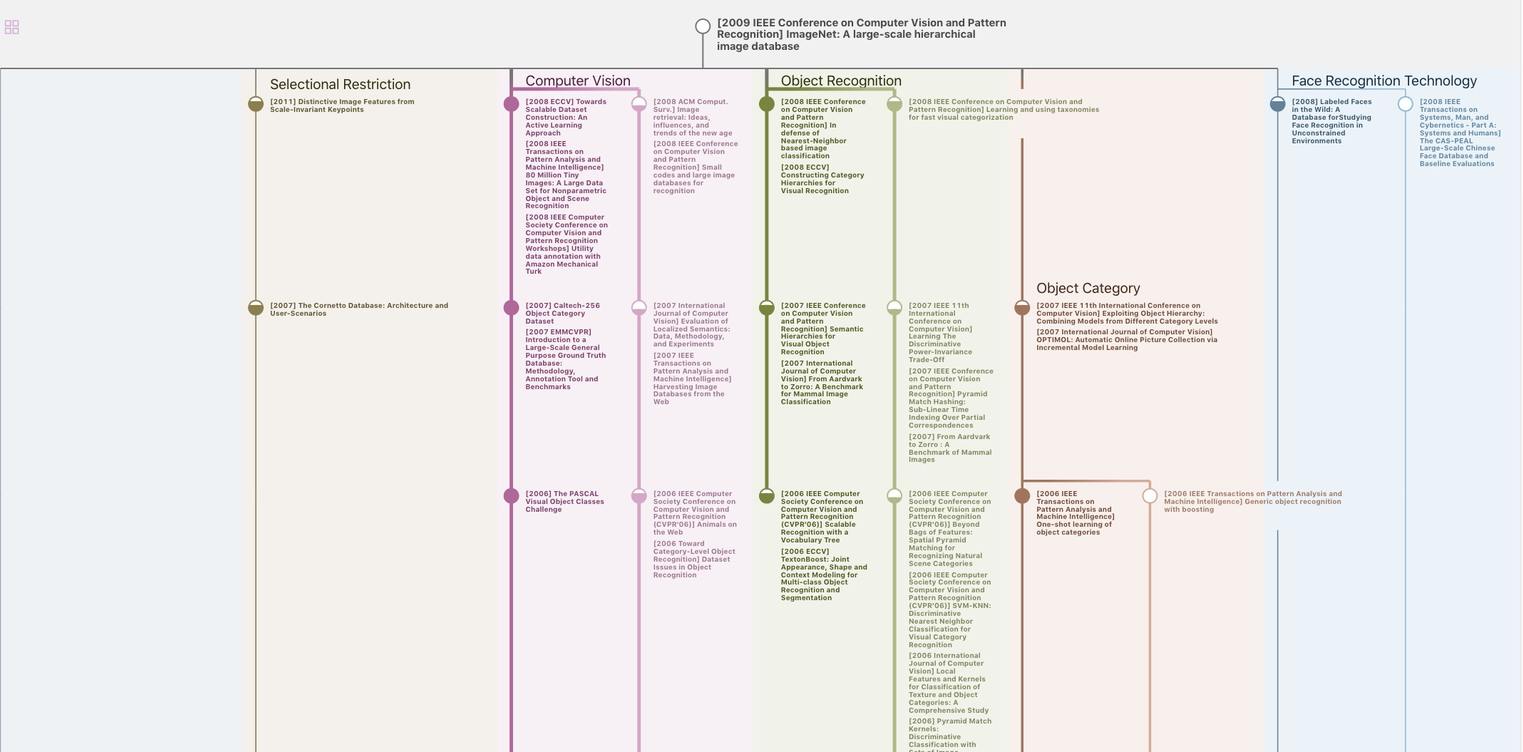
生成溯源树,研究论文发展脉络
Chat Paper
正在生成论文摘要