Modeling interpretable correspondence between cell state and perturbation response with CellCap.
bioRxiv : the preprint server for biology(2024)
摘要
Single-cell transcriptomics, in conjunction with genetic and compound perturbations, offers a robust approach for exploring cellular behaviors in diverse contexts. Such experiments allow un-covering cell-state-specific responses to perturbations, a crucial aspect in unraveling the intricate molecular mechanisms governing cellular behavior and potentially discovering novel regulatory pathways and therapeutic targets. However, prevailing computational methods predominantly focus on predicting average cellular responses, disregarding the inherent response heterogeneity associated with cell state diversity. In this study, we present CellCap, a deep generative model designed for the end-to-end analysis of single-cell perturbation experiments. CellCap employs sparse dictionary learning in a latent space to deconstruct cell-state-specific perturbation responses into a set of transcriptional response programs. These programs are then utilized by each perturbation condition and each cell at varying degrees. The incorporation of specific model design choices, such as dot-product cross-attention between cell states and response programs, along with a linearly-decoded latent space, underlay the interpretation power of CellCap. We evaluate CellCap's model interpretability through multiple simulated scenarios and apply it to two real single-cell perturbation datasets. These datasets feature either heterogeneous cellular populations or a complex experimental setup. Our results demonstrate that CellCap successfully uncovers the relationship between cell state and perturbation response, unveiling novel insights overlooked in previous analyses. The model's interpretability, coupled with its effectiveness in capturing heterogeneous responses, positions CellCap as a valuable tool for advancing our understanding of cellular behaviors in the context of perturbation experiments.
更多查看译文
AI 理解论文
溯源树
样例
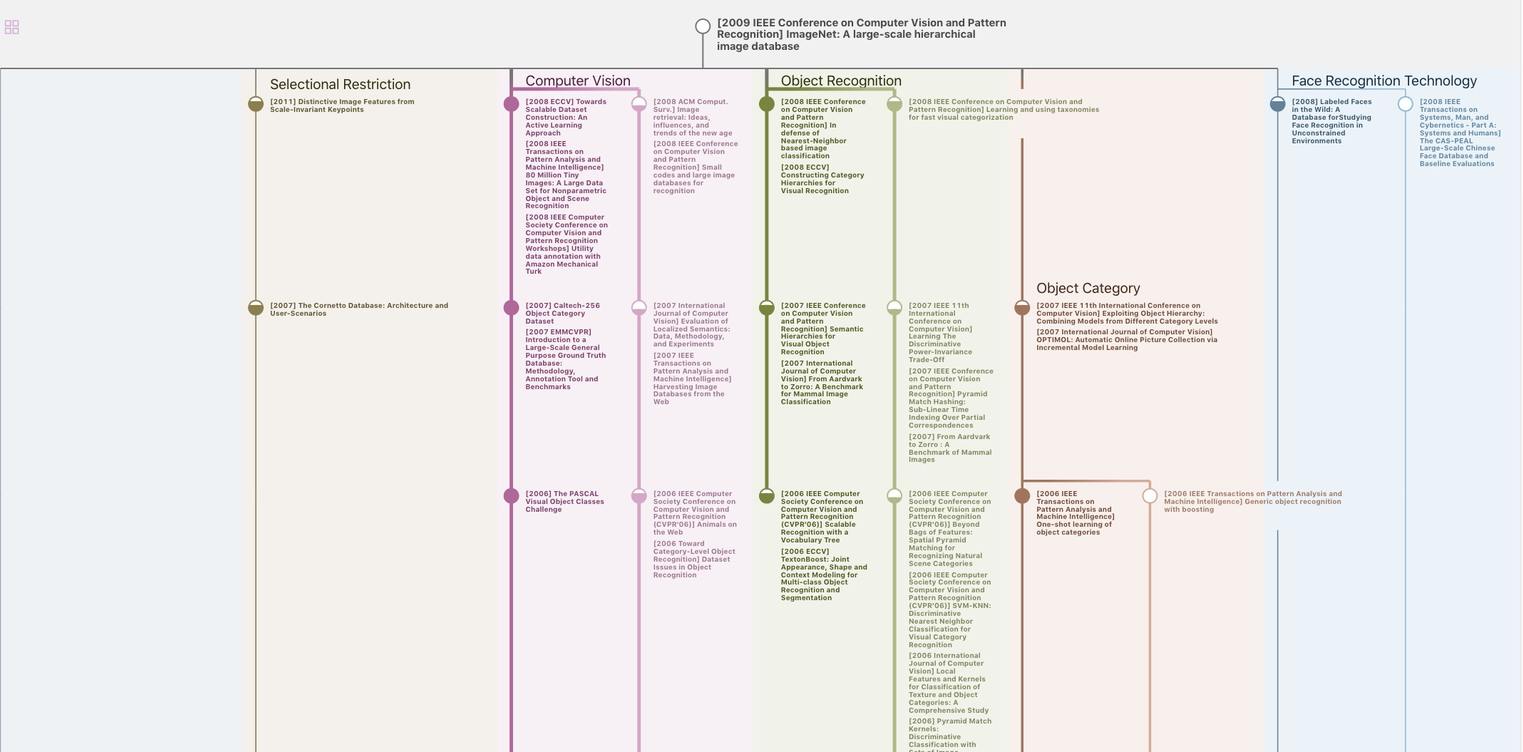
生成溯源树,研究论文发展脉络
Chat Paper
正在生成论文摘要