Physics-constrained neural networks as multi-material Riemann solvers for compressible two-gas simulations
Journal of Computational Science(2024)
摘要
The Riemann solver is crucial in compressible multi-material simulations as it helps predict the behavior of interfacial waves and the interaction between materials. However, the difference of material parameters and the complexity of interface discontinuity mechanisms may lead to difficulties in modeling the coupling effects of multi-material flows under extreme conditions. In this work, we focus on the solution strategy that combines the machine learning with physical constraints for multi-material Riemann problem, as well as its applications in compressible two-gas flows. A neural network architecture is designed to make it suitable for solving the interfacial velocity of multi-material Riemann problem. By means of the construction of physical constraints, the output of neural networks faithfully represents the physical behavior, and the advantages in training convergence and accuracy are obvious for networks with fewer neurons. In addition, a transformation of input data from unbounded domain to bounded domain is proposed, which is capable of improving the applicability of neural networks. Such neural network surrogate model is relatively simple and conveniently applied to the practical ghost fluid method (PGFM), in which only the interfacial velocity solution of Riemann problem is required to define interface conditions. Several typical problems are selected to test the performance of the physics-constrained neural network model. If the offline surrogate model is embedded in the PGFM, the results indicate that it enables an effective implementation for simulating various two-gas flows with a wide range of applications.
更多查看译文
关键词
Compressible two-gas flows,Multi-material Riemann problem,Riemann solver,Neural networks,Physical constraints,Practical ghost fluid method
AI 理解论文
溯源树
样例
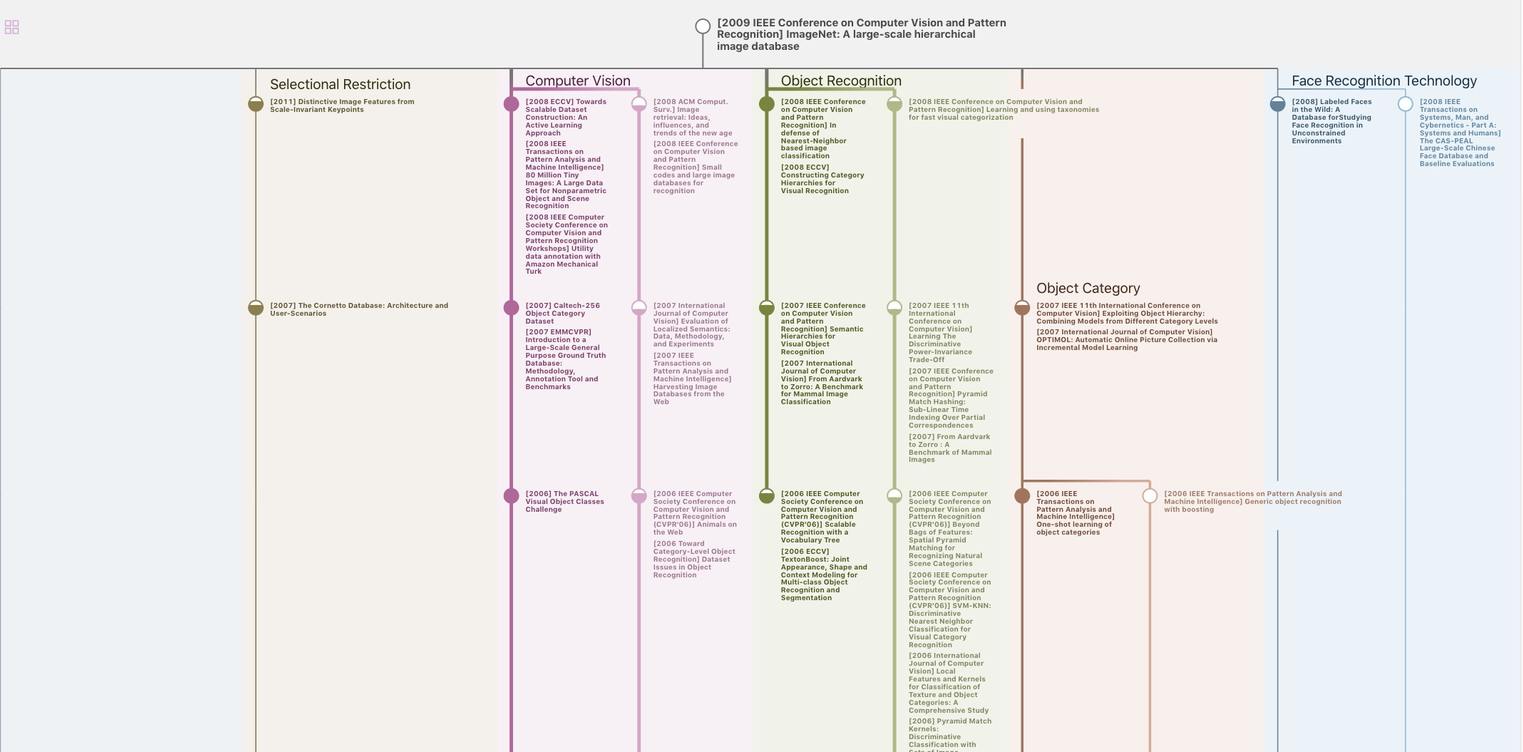
生成溯源树,研究论文发展脉络
Chat Paper
正在生成论文摘要