Multi-Task Residential Short-Term Load Prediction Based on Contrastive Learning
IEEJ TRANSACTIONS ON ELECTRICAL AND ELECTRONIC ENGINEERING(2024)
摘要
Load forecasting is crucial for the operation and planning of electricity generation, transmission, and distribution. In the context of short-term electricity load prediction for residential users, single-task learning methods fail to consider the relationship among multiple residential users and have limited feature extraction capabilities for residential load data. It is challenging to obtain sufficient information from individual residential user load predictions, resulting in poor prediction performance. To address these issues, we propose a framework for multi-task residential short-term load prediction based on contrastive learning. Firstly, clustering techniques are used to select residential users with similar electricity consumption patterns. Secondly, contrastive learning is employed for pre-training, extracting trend and seasonal feature representations of load sequences, thereby enhancing the feature extraction capability for residential load Feature. Lastly, a multi-task learning prediction framework is utilized to learn shared information among multiple residential users' loads, enabling short-term load prediction for multiple residences. The proposed load prediction framework has been implemented on two real-world load data sets, and the experimental results demonstrate that it effectively reduces the prediction errors for residential load prediction. (c) 2024 Institute of Electrical Engineer of Japan and Wiley Periodicals LLC.
更多查看译文
关键词
load prediction,contrastive learning,multi-task learning,deep learning
AI 理解论文
溯源树
样例
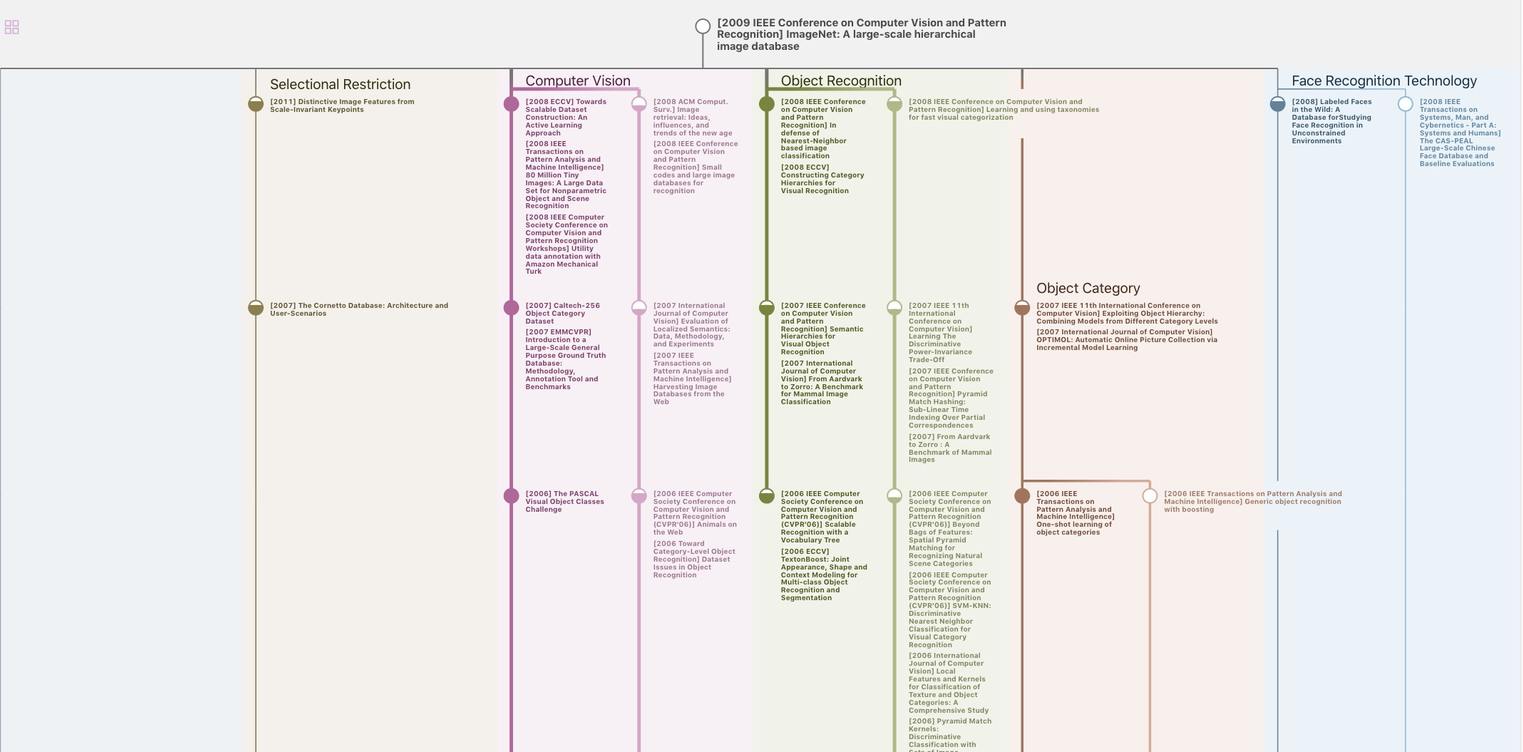
生成溯源树,研究论文发展脉络
Chat Paper
正在生成论文摘要