Evaluating the skill of correlative species distribution models trained with mechanistic model output
ECOLOGICAL MODELLING(2024)
摘要
Predicting the change in the distribution pattern of organisms is critical for assessing and mitigating risks associated with climate change and environmental variability. Correlative species distribution models (SDMs), which relate species' abundances to environmental data, are particularly useful for generating such predictions as they do not require a priori insight into the complex species' dynamics. Although correlative SDMs are typically developed using in situ environmental observations, their predictions are commonly created by applying SDMs with environmental information generated by mechanistic models. This can expand the temporal and spatial domain of the projections; however, this may also decrease the SDM prediction skill because of biases associated with the mechanistic model output. We test the hypothesis that training SDMs using environmental mechanistic model output may enhance model prediction skill by compensating for biases in the mechanistic model. We train SDMs for seven estuarine algal taxa observed in the Chesapeake Bay (U.S.A.) using both multi-decadal in situ environmental observations and mechanistic environmental output provided by a 3D coupled hydrodynamic-biogeochemical model. Training the SDMs using mechanistic model output, rather than in situ data, improves the model prediction skill by more than 10%. This demonstrates that although errors in SDM predictions can be caused by using imperfect environmental fields derived from mechanistic models, these errors may be diminished by training SDMs using these same environmental fields.
更多查看译文
关键词
Species distribution modeling,Model prediction skill,Forecasting,Statistical training,Mechanistic models,Data assimilation,Generalized linear models,Harmful algal bloom,Chesapeake Bay
AI 理解论文
溯源树
样例
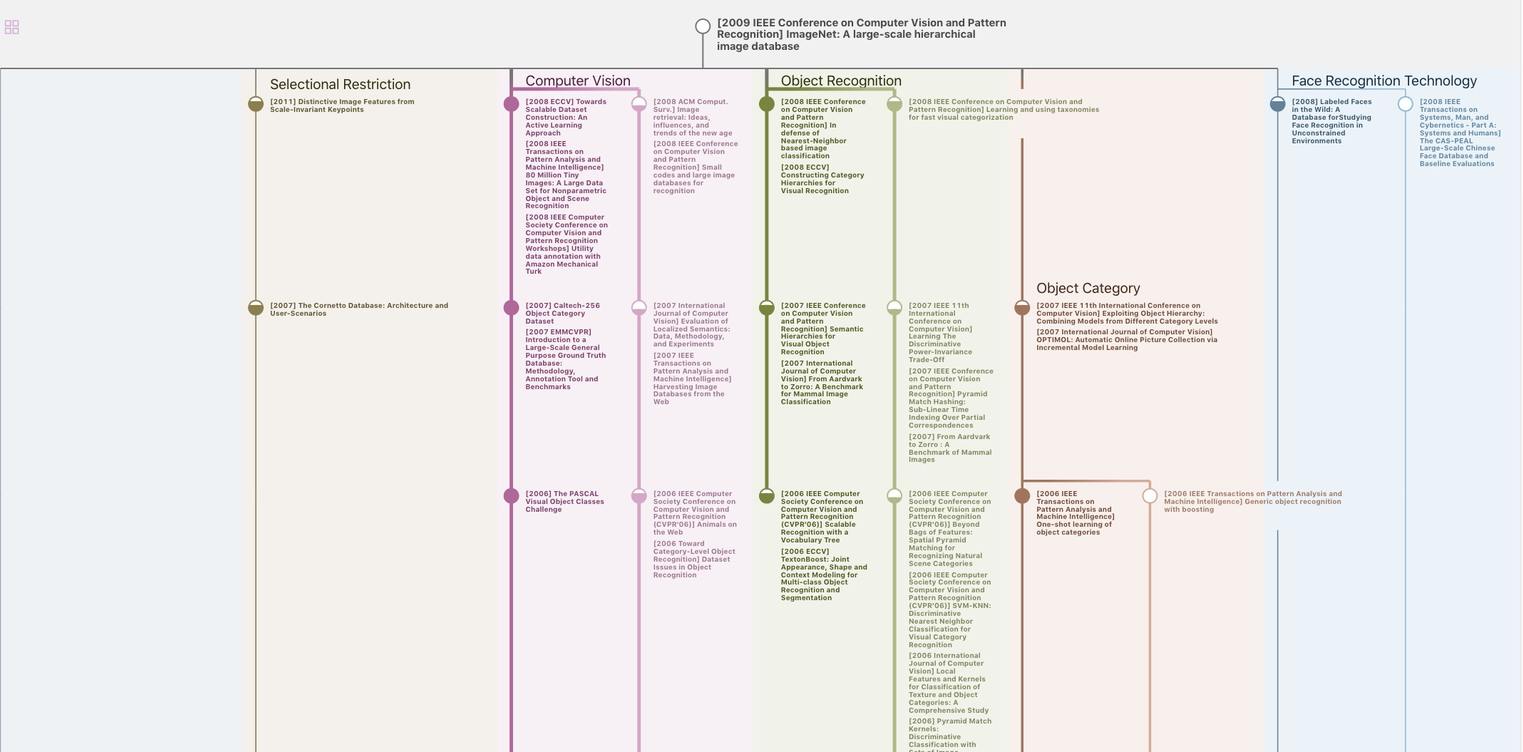
生成溯源树,研究论文发展脉络
Chat Paper
正在生成论文摘要