Health Care Cost Reductions with Machine Learning-Directed Evaluations during Radiation Therapy - An Economic Analysis of a Randomized Controlled Study.
NEJM AI(2024)
摘要
BACKGROUND:Machine learning (ML) may cost-effectively direct health care by identifying patients most likely to benefit from preventative interventions to avoid negative and expensive outcomes. System for High-Intensity Evaluation During Radiation Therapy (SHIELD-RT; NCT04277650) was a single-institution, randomized controlled study in which electronic health record-based ML accurately identified patients at high risk for acute care (emergency visit or hospitalization) during radiotherapy (RT) and targeted them for supplemental clinical evaluations. This ML-directed intervention resulted in decreased acute care utilization. Given the limited prospective data showing the ability of ML to direct interventions cost-efficiently, an economic analysis was performed.
METHODS:A post hoc economic analysis was conducted of SHIELD-RT that included RT courses from January 7, 2019, to June 30, 2019. ML-identified high-risk courses (≥10% risk of acute care during RT) were randomized to receive standard of care weekly clinical evaluations with ad hoc supplemental evaluations per clinician discretion versus mandatory twice-weekly evaluations. The primary outcome was difference in mean total medical costs during and 15 days after RT. Acute care costs were obtained via institutional cost accounting. Physician and intervention costs were estimated via Medicare and Medicaid data. Negative binomial regression was used to estimate cost outcomes after adjustment for patient and disease factors.
RESULTS:A total of 311 high-risk RT courses among 305 patients were randomized to the standard (n=157) or the intervention (n=154) group. Unadjusted mean intervention group supplemental visit costs were $155 per course (95% confidence interval, $142 to $168). The intervention group had fewer acute care visits per course (standard, 0.47; intervention, 0.31; P=0.04). Total mean adjusted costs were $3110 per course for the standard group and $1494 for the intervention group (difference in means, $1616 [95% confidence interval, $1450 to $1783]; P=0.03).
CONCLUSIONS:In this economic analysis of a randomized controlled, health care ML study, mandatory supplemental evaluations for ML-identified high-risk patients were associated with both reduced total medical costs and improved clinical outcomes. Further study is needed to determine whether economic results are generalizable. (Funded in part by The Duke Endowment, The Conquer Cancer Foundation, the Duke Department of Radiation Oncology, and the National Cancer Institute of the National Institutes of Health [R01CA277782]; ClinicalTrials.gov number, NCT04277650.).
更多查看译文
AI 理解论文
溯源树
样例
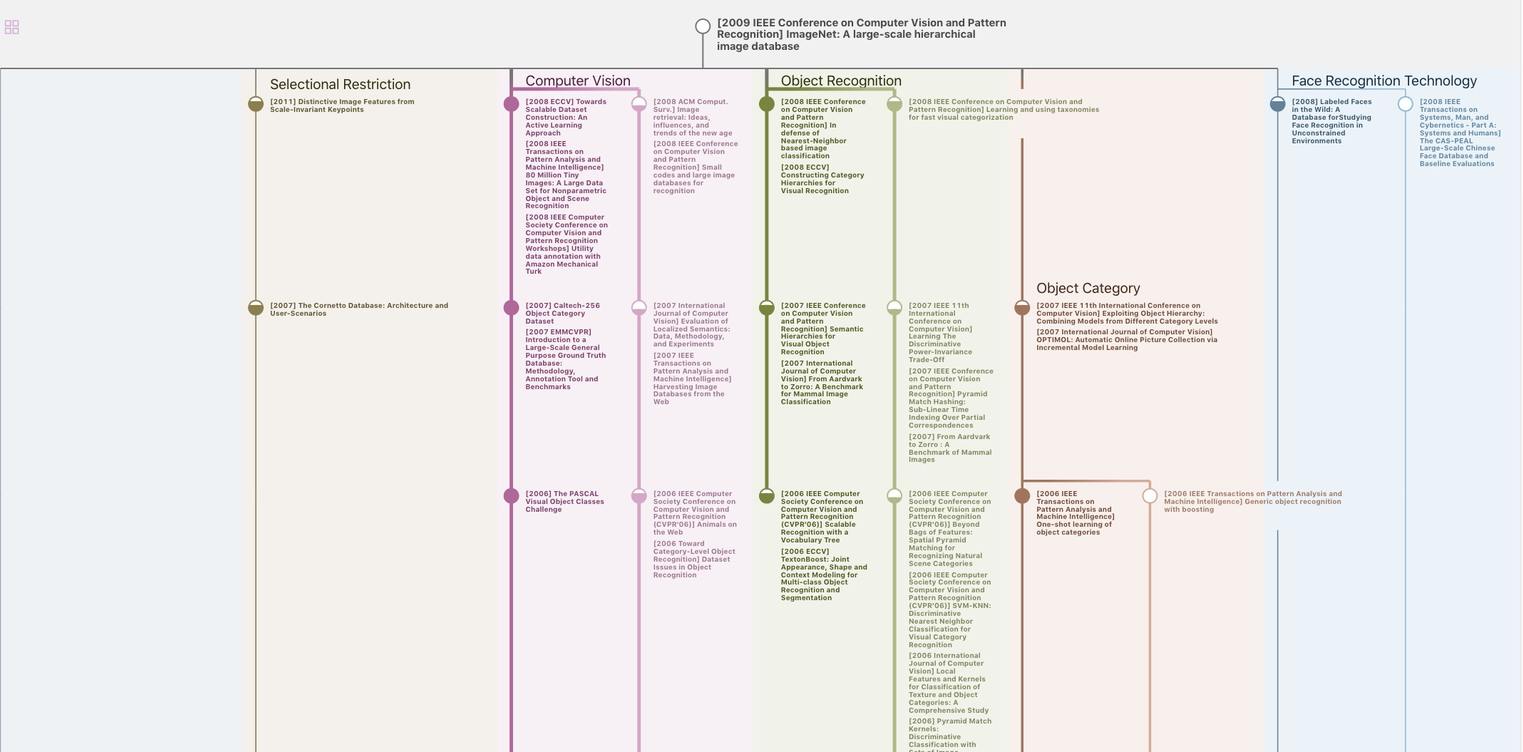
生成溯源树,研究论文发展脉络
Chat Paper
正在生成论文摘要