ML- and LSTM-Based Radiator Predictive Maintenance for Energy Saving in Compressed Air Systems
ENERGIES(2024)
摘要
Air compressors are widely used in industrial fields. Compressed air systems aggregate air flows and then supply them to places of demand. These huge systems consume a significant amount of energy and generate heat internally. Machine components in compressed air systems are vulnerable to heat, and, in particular, a radiator to cool the heat of the overall air compressor is the core component. Dirty radiators increase energy consumption due to anomalous cooling. To reduce the energy consumption of air compressors, this mechanism emphasizes a machine learning-based radiator fault detection, using features such as RPM, motor power, outlet pressure, air flow, water pump power, and outlet temperature with slight true fault labels. Moreover, the proposed system adds an LSTM-based motor power prediction model to point out the initial judgment of radiator fault possibility. Via the rigorous analysis and the comparison among machine learning models, this meticulous approach improves the performance of radiator fault prediction up to 93.0%, and decreases the mean power consumption of the air compressor around 2.24%.
更多查看译文
关键词
predictive maintenance,air compressor,machine learning,fault detection,radiator,energy consumption
AI 理解论文
溯源树
样例
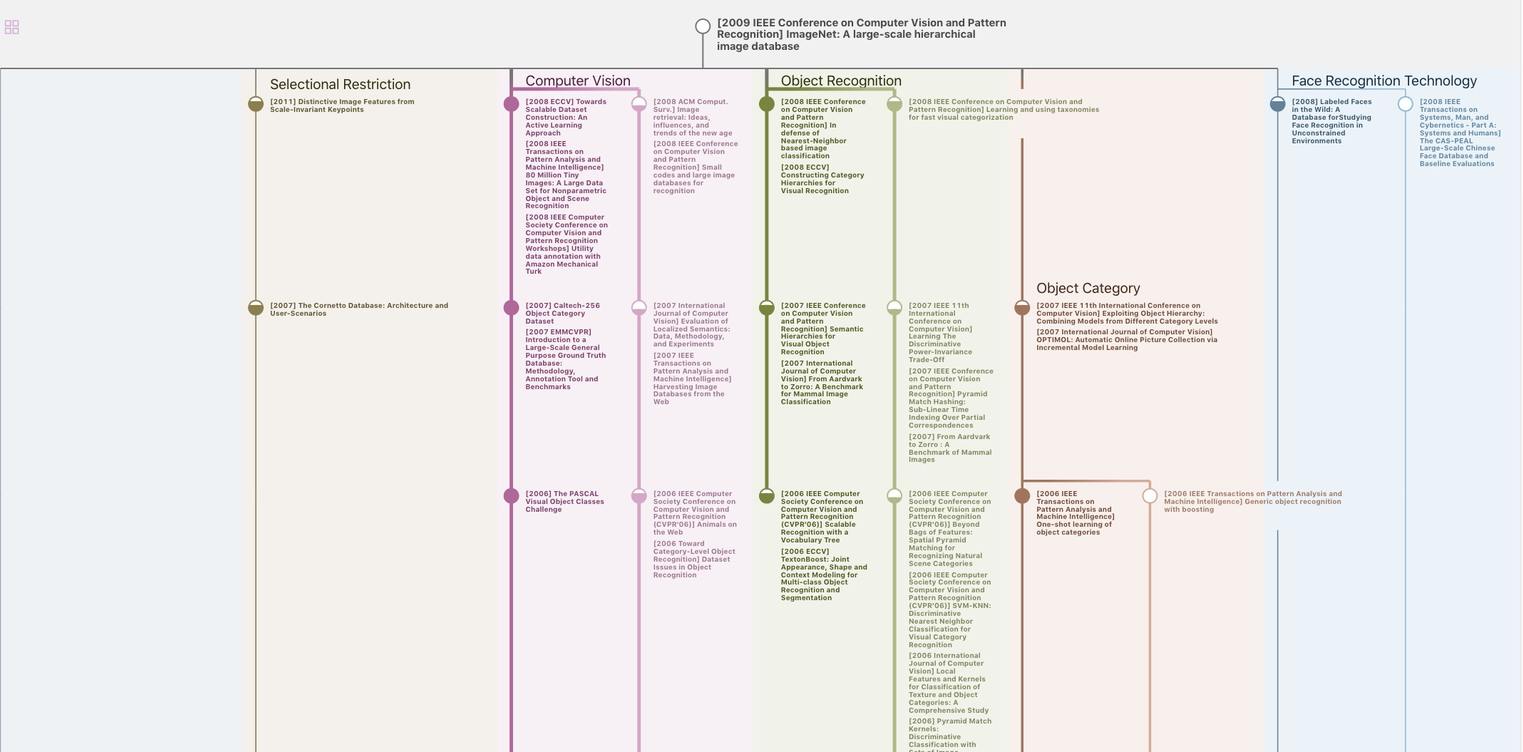
生成溯源树,研究论文发展脉络
Chat Paper
正在生成论文摘要