Deep Learning Insights into ASD: Classifying and Unveiling Behavioural Patterns through RoBERTa and Topic Modeling on QCHAT Data.
crossref(2024)
摘要
Abstract This study leverages advanced Natural Language Processing (NLP) models, including Bidirectional Encoder Representations from Transformers (BERT), A Robustly Optimized BERT Pretraining Approach (RoBERTa), and Topic Modeling, to analyze behavioral patterns in Autism Spectrum Disorder (ASD). Using the Quantitative Checklist for Autism in Toddlers (QCHAT) dataset enhanced with ASD-related behavioral terms, we demonstrate the potential of these models to improve ASD vs. Typically Developing (TD) classification accuracy and uncover key behavioral themes indicative of ASD. Our findings highlight the value of enriching clinical datasets with domain-specific knowledge and showcase the power of adapting deep learning techniques for ASD research. This work contributes to developing more accurate and informative ASD diagnostic tools.
更多查看译文
AI 理解论文
溯源树
样例
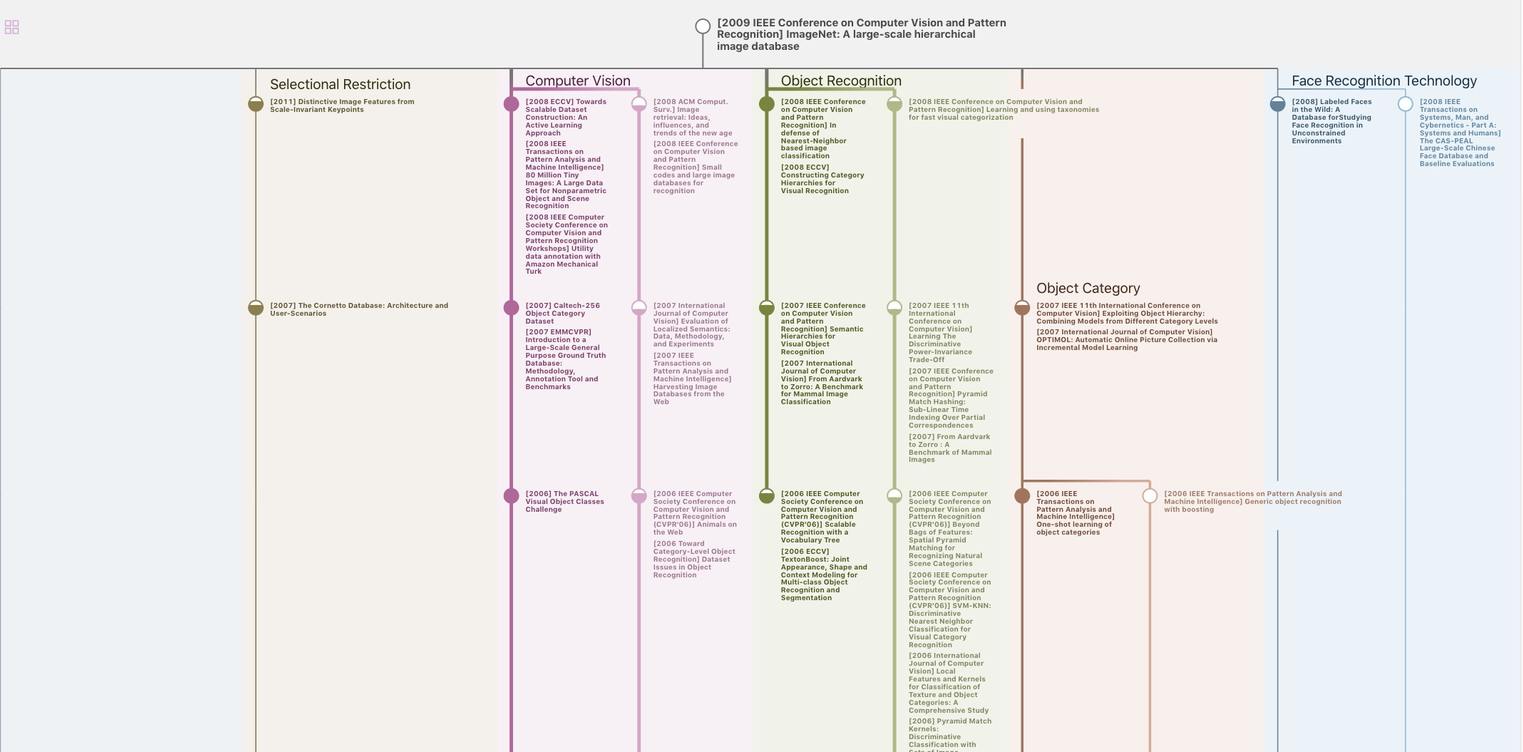
生成溯源树,研究论文发展脉络
Chat Paper
正在生成论文摘要