224 Artificial Intelligence-based Decision Support Predicts Requirement for Neurosurgical Intervention in Acute Traumatic Brain Injury: Automated Surgical Intervention Support Tool (ASIST-TBI) Development, Validation and Simulated Prospective Deployment
Neurosurgery(2024)
AI 理解论文
溯源树
样例
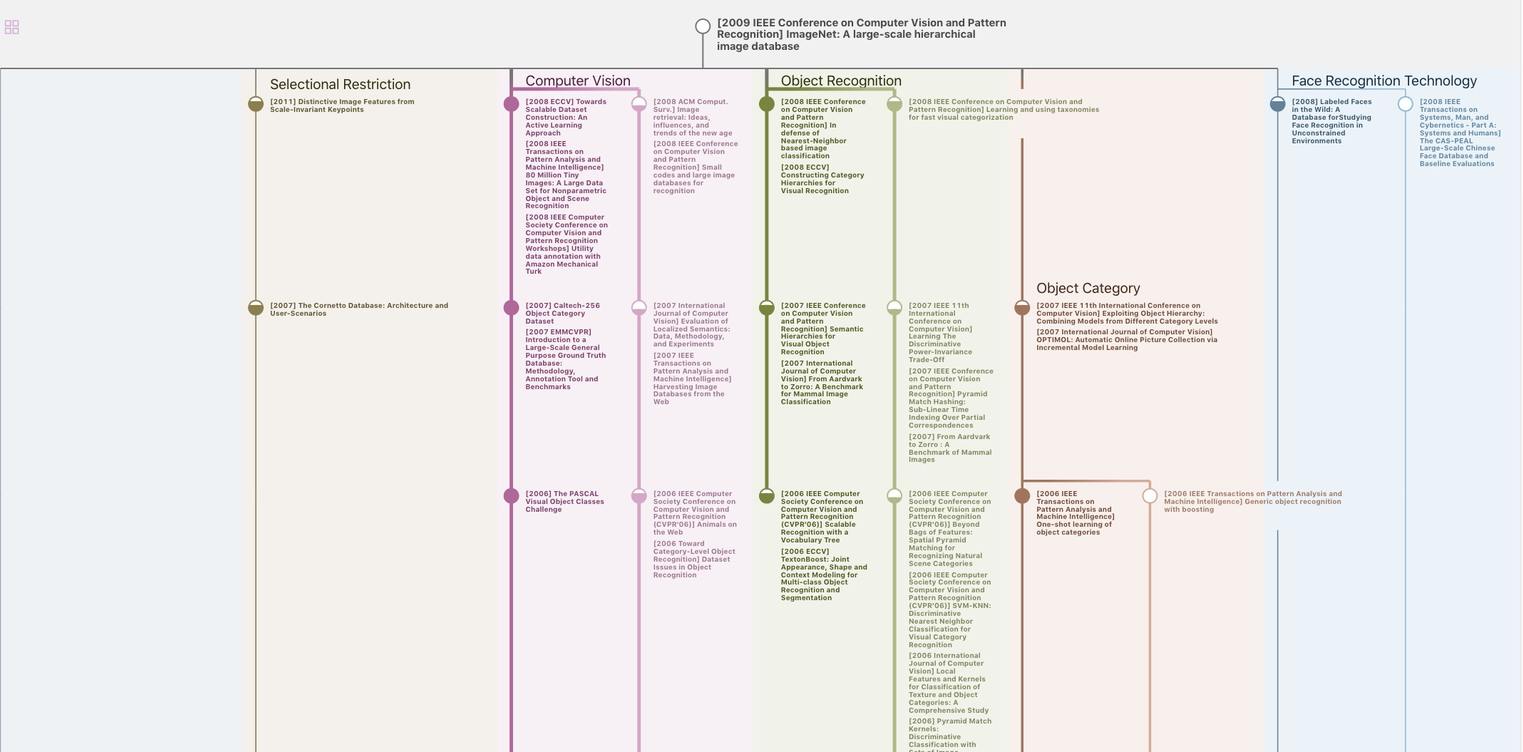
生成溯源树,研究论文发展脉络
Chat Paper
正在生成论文摘要