Simulation Learning and Optimization: Methodology and Applications
ASIA-PACIFIC JOURNAL OF OPERATIONAL RESEARCH(2024)
摘要
Due to the rapid advancement of computational power and the Internet of Things (IoT) together with recent developments in digital-twin and cyber-physical systems, the integration of big data analytics techniques (BDAT) (e.g., data mining, machine learning (ML), deep learning, data farming, etc.) into traditional simulation methodologies, along with the enhancement of past simulation optimization techniques, has given rise to an emerging field known as simulation learning and optimization (SLO). Unlike simulation optimization, SLO is a data-driven fusion approach that integrates conventional simulation modeling methodologies, simulation optimization techniques, and learning-based big data analytics (BDA) to enhance the ability to address stochastic and dynamic decision-making problems in the real-world complex system and quickly find the best outcomes for the decision support in both real-time and nonreal-time analyses to achieve the data-driven digital twin capability. Although some literature in the past has mentioned similar applications and concepts, no paper provides a structural explanation and comprehensive review of relevant literature on SLO from both methodological and applied perspectives. Consequently, in the first part of this paper, we conduct a literature review on a novel SLO methodology emerging from the fusion of traditional simulation methodology, simulation optimization algorithms, and BDAT. In the second part of this paper, we review the applications of SLO in various contexts, with detailed discussions on manufacturing, maintenance, redundancy allocation, hybrid energy systems, humanitarian logistics, and healthcare systems. Lastly, we investigate potential research directions for SLO in this new era of big data and artificial intelligence (AI).
更多查看译文
关键词
Simulation optimization,simulation learning,big data analytics,data-driven,machine learning,deep learning
AI 理解论文
溯源树
样例
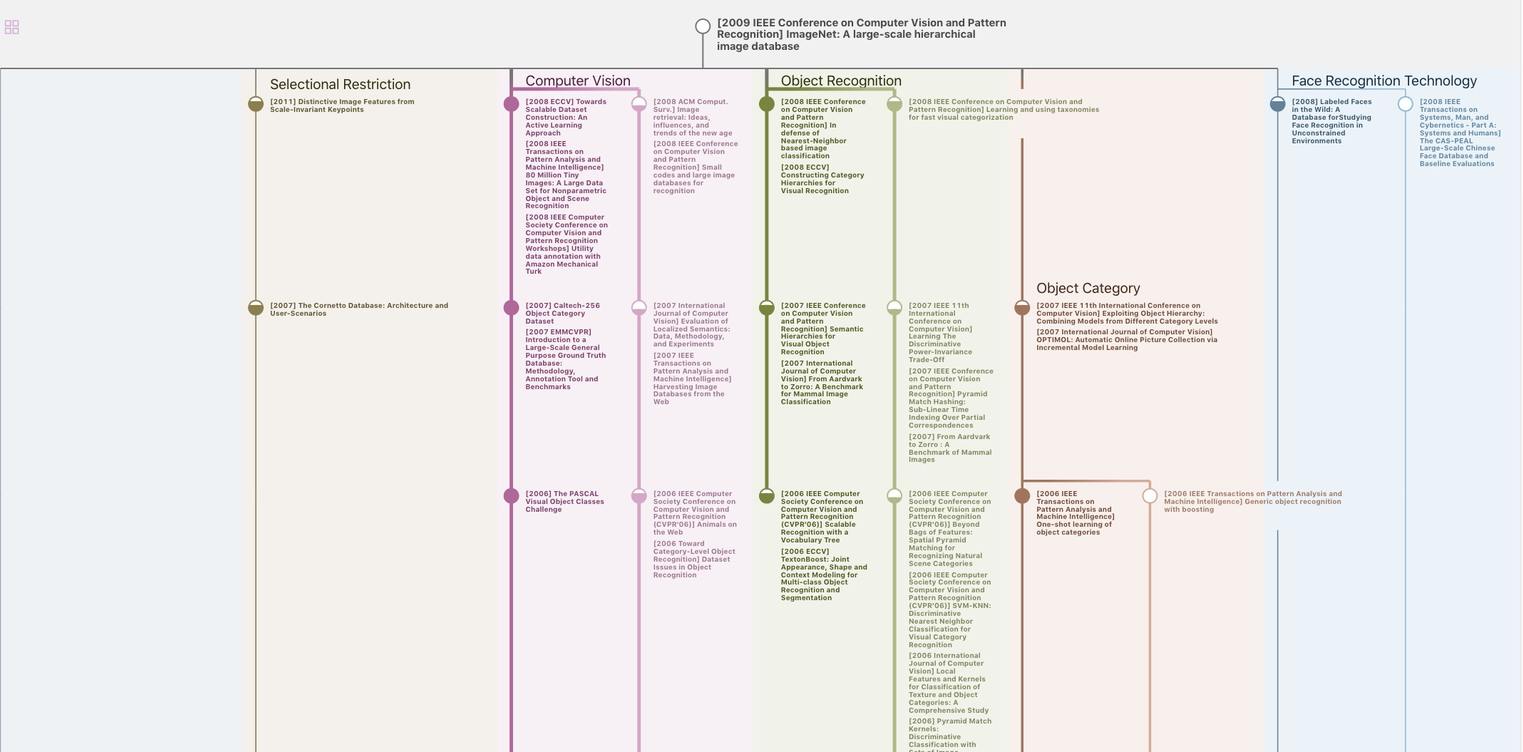
生成溯源树,研究论文发展脉络
Chat Paper
正在生成论文摘要