Score Dynamics: Scaling Molecular Dynamics with Picoseconds Time Steps via Conditional Diffusion Model
JOURNAL OF CHEMICAL THEORY AND COMPUTATION(2024)
摘要
We propose score dynamics (SD), a general framework for learning accelerated evolution operators with large timesteps from molecular dynamics (MD) simulations. SD is centered around scores or derivatives of the transition log-probability with respect to the dynamical degrees of freedom. The latter play the same role as force fields in MD but are used in denoising diffusion probability models to generate discrete transitions of the dynamical variables in an SD time step, which can be orders of magnitude larger than a typical MD time step. In this work, we construct graph neural network-based SD models of realistic molecular systems that are evolved with 10 ps timesteps. We demonstrate the efficacy of SD with case studies of the alanine dipeptide and short alkanes in aqueous solution. Both equilibrium predictions derived from the stationary distributions of the conditional probability and kinetic predictions for the transition rates and transition paths are in good agreement with MD. Our current SD implementation is about 2 orders of magnitude faster than the MD counterpart for the systems studied in this work. Open challenges and possible future remedies to improve SD are also discussed.
更多查看译文
AI 理解论文
溯源树
样例
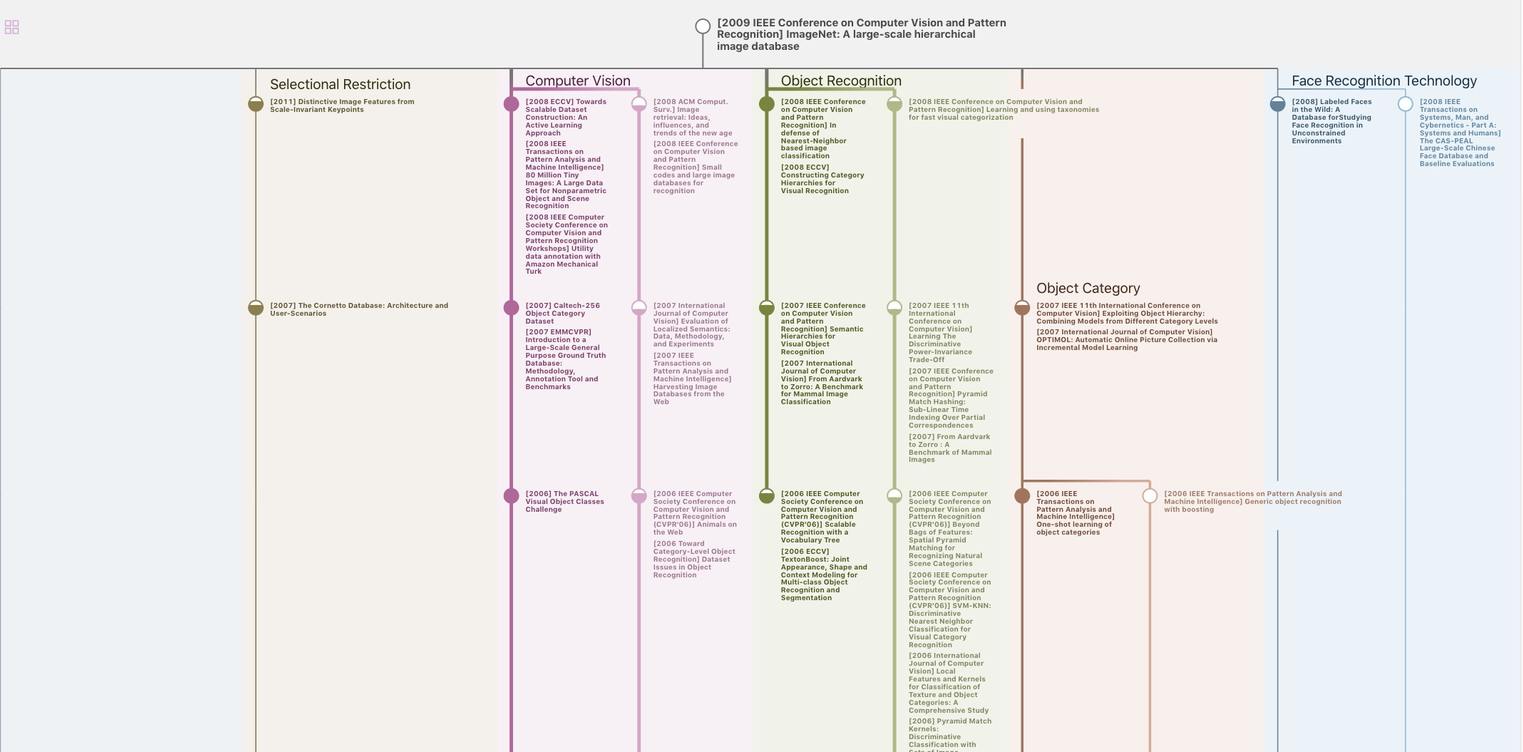
生成溯源树,研究论文发展脉络
Chat Paper
正在生成论文摘要