FEGR: Feature Enhanced Graph Representation Method for Graph Classification
PROCEEDINGS OF THE 2023 IEEE/ACM INTERNATIONAL CONFERENCE ON ADVANCES IN SOCIAL NETWORKS ANALYSIS AND MINING, ASONAM 2023(2023)
摘要
Graph representation plays a key role in graph analytics to perform a variety of downstream machine-learning tasks. This paper presents a novel method for extracting expressive graph representation based on a combination of statistics captured from a graph and node properties. We use both local and global-level information along with the original node properties to extract a meaningful feature representation of the graph. This allows us to build expressive graph descriptors that can be run with limited training data and computational resources and achieve competitive results. We discuss the merits of the proposed approach in terms of sensitivity, running times, and stability. Our evaluation of various graph classification benchmark datasets shows that the proposed method either outperforms or provides similar results to state-of-the-art methods. We further outline the potential future directions in graph machine learning research.
更多查看译文
关键词
Graph representation,concatenated features,Non-parametric approach,Graph descriptor
AI 理解论文
溯源树
样例
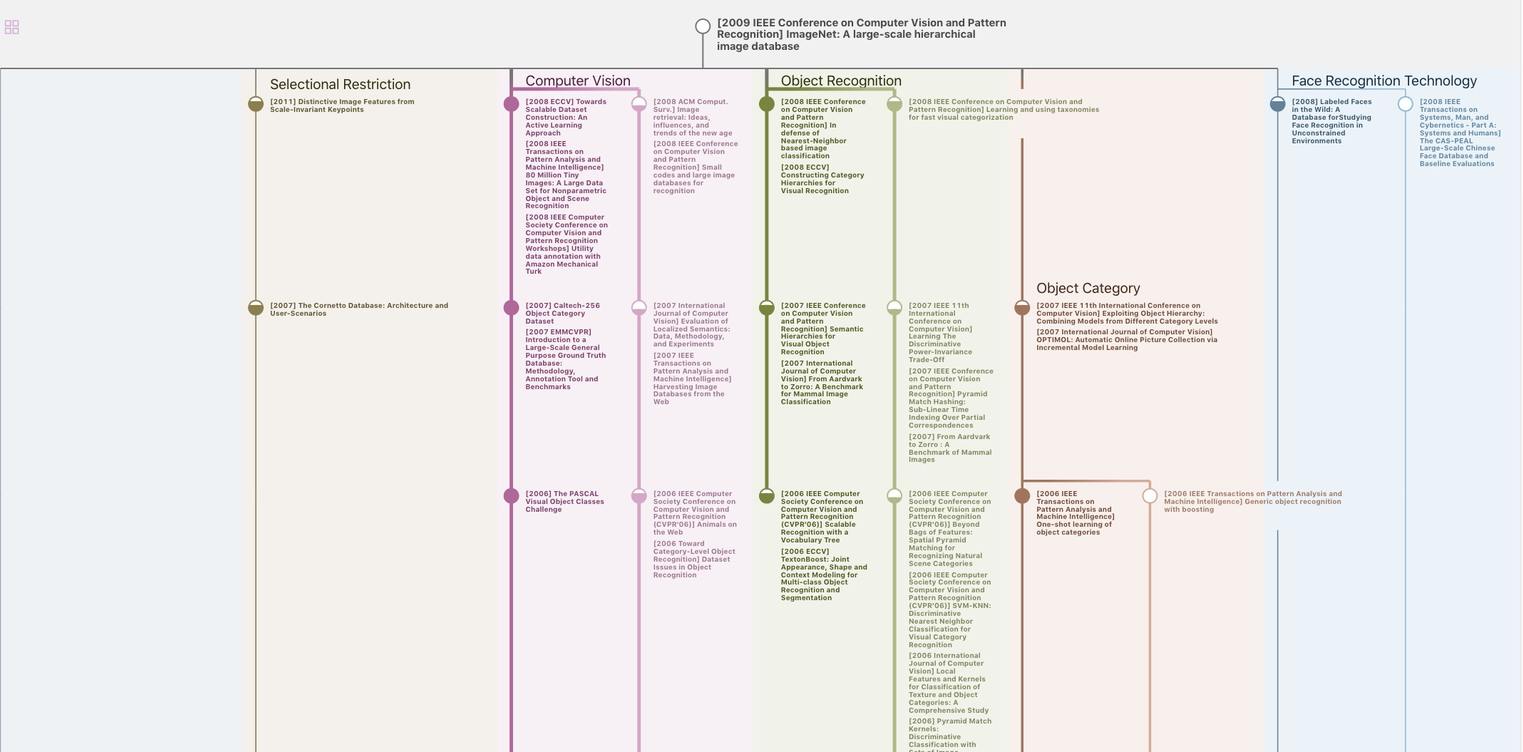
生成溯源树,研究论文发展脉络
Chat Paper
正在生成论文摘要