How good are machine learning clouds? Benchmarking two snapshots over 5 years
The VLDB Journal(2024)
摘要
We conduct an empirical study of machine learning functionalities provided by major cloud service providers, which we call machine learning clouds. Machine learning clouds hold the promise of hiding all the sophistication of running large-scale machine learning: Instead of specifying how to run a machine learning task, users only specify what machine learning task to run and the cloud figures out the rest. Raising the level of abstraction, however, rarely comes free—a performance penalty is possible. How good, then, are current machine learning clouds on real-world machine learning workloads? We study this question by conducting benchmark on the mainstream machine learning clouds. Since these platforms continue to innovate, our benchmark tries to reflect their evolvement. Concretely, this paper consists of two sub-benchmarks—mlbench and automlbench. When we first started this work in 2016, only two cloud platforms provide machine learning services and limited themselves to model training and simple hyper-parameter tuning. We then focus on binary classification problems and present mlbench, a novel benchmark constructed by harvesting datasets from Kaggle competitions. We then compare the performance of the top winning code available from Kaggle with that of running machine learning clouds from both Azure and Amazon on mlbench. In the recent few years, more cloud providers support machine learning and include automatic machine learning (AutoML) techniques in their machine learning clouds. Their AutoML services can ease manual tuning on the whole machine learning pipeline, including but not limited to data preprocessing, feature selection, model selection, hyper-parameter, and model ensemble. To reflect these advancements, we design automlbench to assess the AutoML performance of four machine learning clouds using different kinds of workloads. Our comparative study reveals the strength and weakness of existing machine learning clouds and points out potential future directions for improvement.
更多查看译文
关键词
Machine learning cloud,Automatic machine learning,Benchmark
AI 理解论文
溯源树
样例
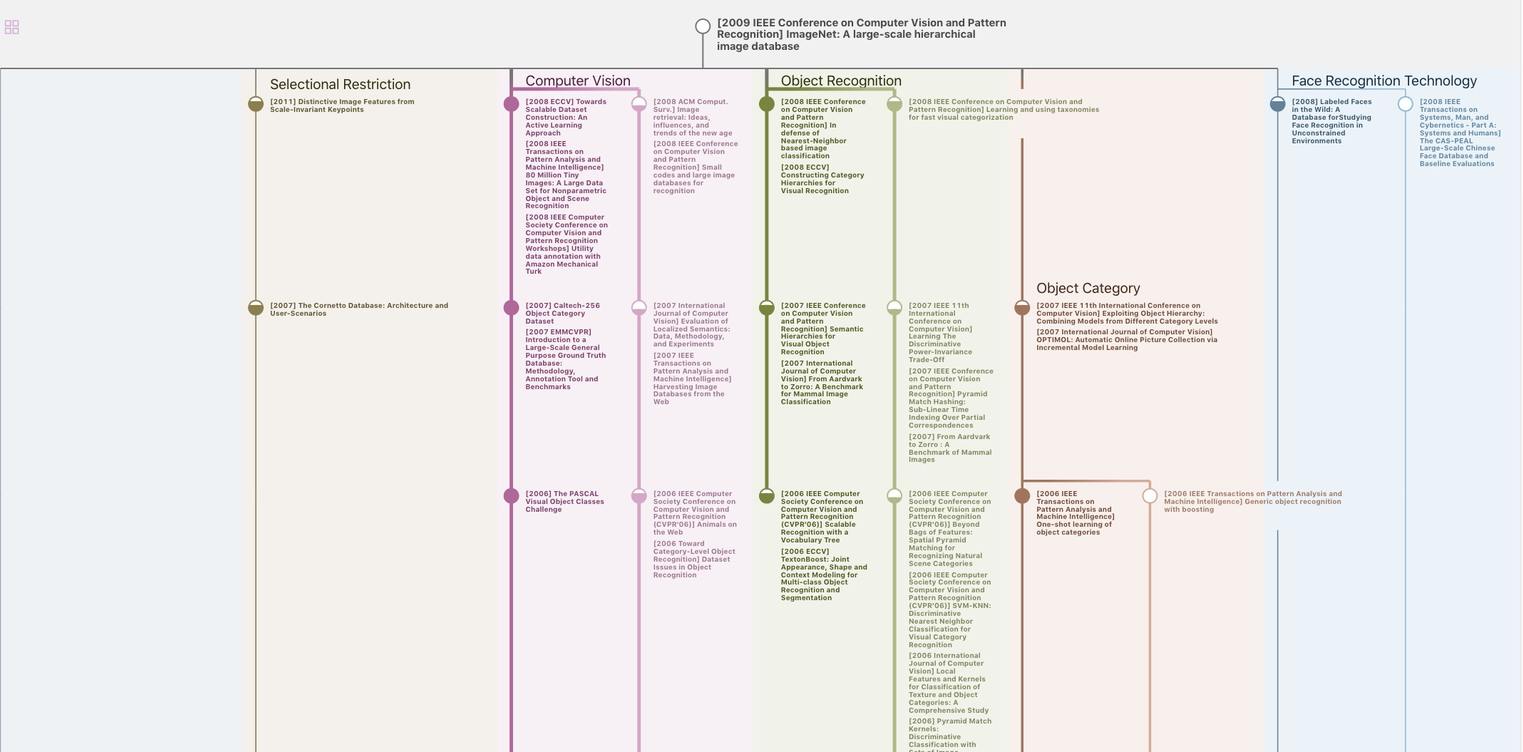
生成溯源树,研究论文发展脉络
Chat Paper
正在生成论文摘要