Decoupled interpretable robust domain generalization networks: A fault diagnosis approach across bearings, working conditions, and artificial-to-real scenarios
Advanced Engineering Informatics(2024)
摘要
Increasing the generalizability of intelligent diagnostic models amidst data distribution shifts is receiving growing attention. Nevertheless, current domain generalization methods primarily enhance fault diagnosis in variable working conditions or machines. Due to the lack of prior knowledge to determine which features are task-unrelated and which features are task-related, existing methods typically learn coupled features. Facing industrial diagnosis scenarios across bearings and artificial-to-real faults, coupled features induce false correlations that limit the model's generalizability. To address this challenge, this paper proposes a decoupled interpretable robust domain generalization network (DIRNet) to enhance model generalizability by interpretably transferring fault-related components. First, this paper constructs a neural basis function decoupling module to disentangle the signal into fault-related and fault-unrelated basis functions. Second, a dynamic Shapley pruning network is proposed to dynamically prune the fault-unrelated neural basis functions, achieving the generalization of fault-related basis functions. Third, we introduce a loss function that relies on interpretable basis function selection to enhance the expressive capability of the basis function decoupling module. Experiments on a self-collected industrial distributed fault bearing case and two laboratory cases are carried out. The results demonstrate that DIRNet can obtain generalizable fault-related components to effectively deal with industrial across bearings and artificial-to-real fault diagnosis scenarios compared to the previous methods.
更多查看译文
关键词
Domain generalization,Bearing fault diagnosis,Basis function decoupling,Shapley explanations
AI 理解论文
溯源树
样例
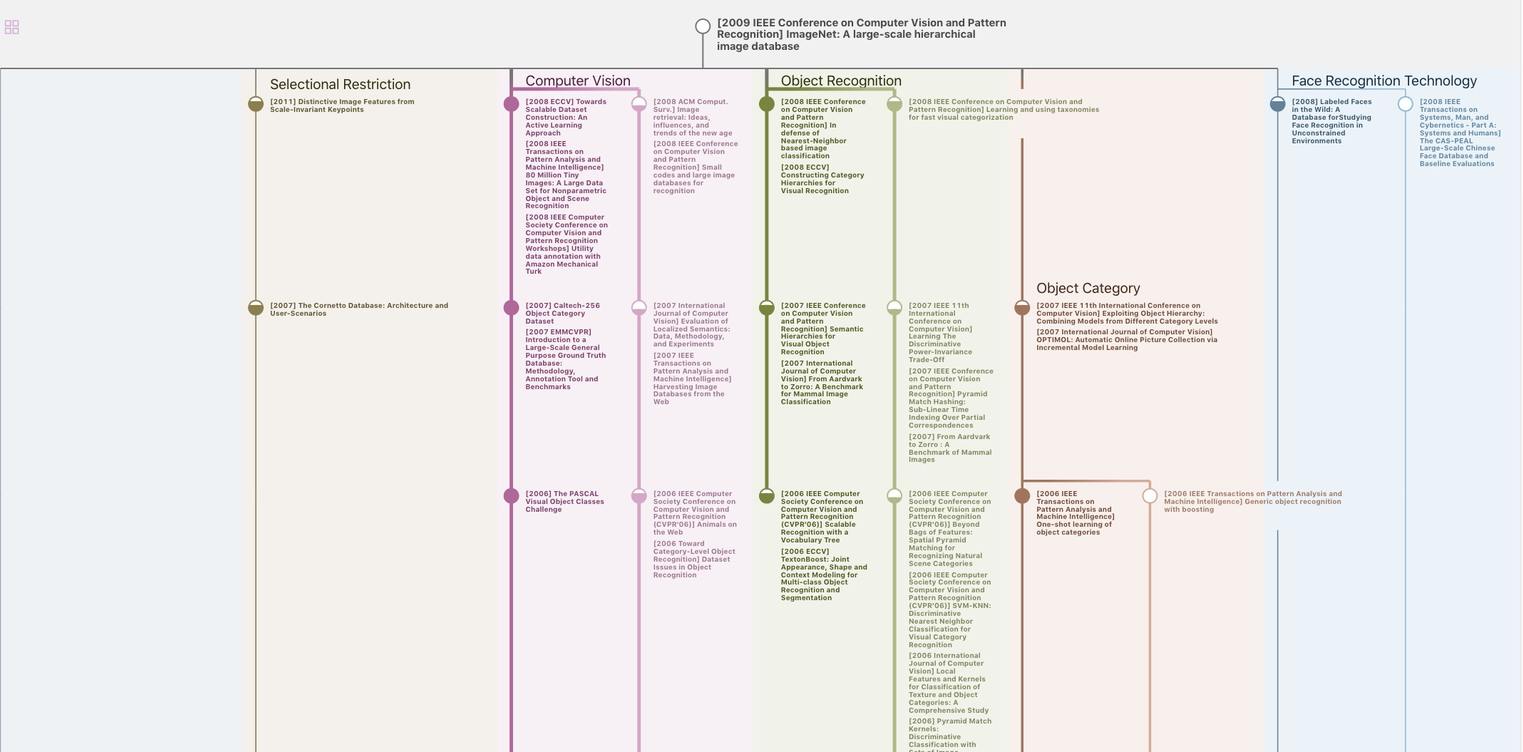
生成溯源树,研究论文发展脉络
Chat Paper
正在生成论文摘要