Learning-Based Energy Minimization Optimization for IRS-Assisted Master-Auxiliary-UAV-Enabled Wireless-Powered IoT Networks
IEEE Internet of Things Journal(2024)
摘要
This paper investigates master-auxiliary unmanned aerial vehicles (UAVs)-enabled wireless-powered Internet-of-Things (WPIoT) networks, which overcome the inflexibility and site selection issues caused by traditional fixed-point intelligent reflecting surface (IRS). Specifically, multiple rechargeable Master UAVs (MUAVs) and IRS-integrated Auxiliary UAVs (AUAVs) are applied in pairs to cooperatively charge and collect data from IoT devices clustered in different subareas under cloud scheduling. Given the constraints of limited onboard battery capacity and complete data collection, we formulate a system energy minimization problem, which is then divided into three sub-problems. We first utilize a pair of U-nets with quantization layers trained by deep unsupervised learning (DUL) to output discrete downlink (DL) and uplink (UL) IRS phases separately. Gradient functions with specific features are also proposed to solve non-differentiable issue during training. Given the optimized IRS phase policies, off-policy deep reinforcement learning (DRL) is exploited to optimize intra-subarea data collection policy and inter-subarea multi-UAV scheduling scheme. Two assistive techniques, positive transition initialization (PTI) and action mask, are proposed to guide the learning of the agent and alleviate the burden of optimization. Numerical results indicate that our proposed approach combining DUL with DRL can improve performance by more than 90% compared to the pure DRL method. Furthermore, our proposed Master-Auxiliary-UAV collaborative data collection scheme (MACS) can achieve better energy performance than homogeneous MUAV data collection scheme (HMCS) in cases with low onboard battery capacity while halving task completion time.
更多查看译文
关键词
WPIoT,rechargeable UAVs,IRS,DUL,DRL
AI 理解论文
溯源树
样例
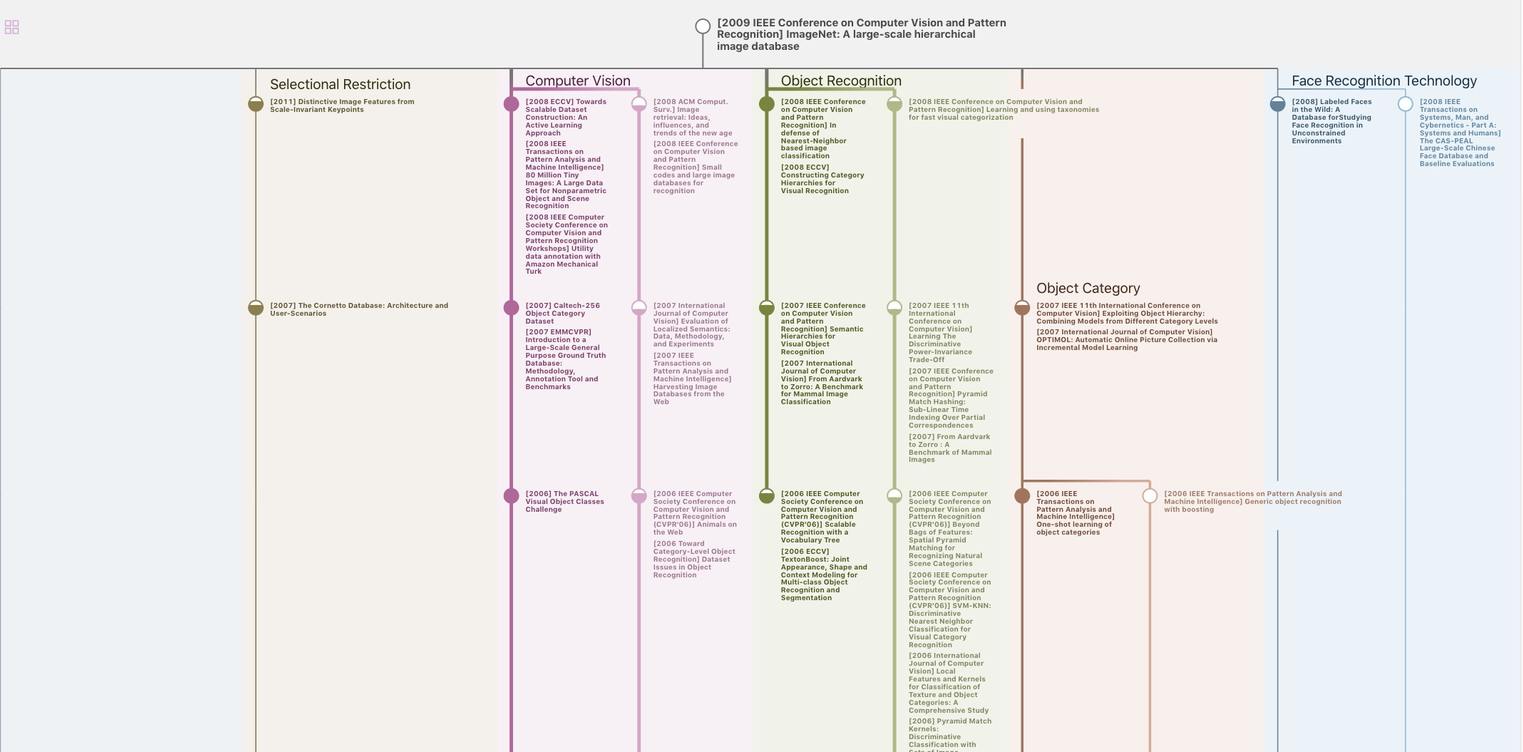
生成溯源树,研究论文发展脉络
Chat Paper
正在生成论文摘要