The model architecture search system for chromosome image classification
Signal, Image and Video Processing(2024)
摘要
The classification of chromosome images holds immense significance in the fields of genetics, clinical diagnostics, and medical research. It plays a pivotal role in the precise identification of genetic abnormalities, allowing for early and accurate diagnosis of various genetic disorders and birth defects. The automation of this process offers significant advantages in terms of time and human resource savings. This study introduces the Model Architecture Search System (MASS), designed to adapt itself to classify chromosome images for karyotyping. The MASS framework aims to construct an optimal model architecture for the specific classification task by leveraging predefined CNN backbones, activation functions, and loss functions. There are 12 pre-trained networks, 5 activation functions, and 2 loss functions in the selection set of the MASS. The proposed framework utilizes the Tree-structured Parzen Estimator (TPE) algorithm based on Bayesian Optimization, eliminating the need for manual model searching processes and finding optimal model architecture. The suitable model structure for the relevant dataset is generated from these groups automatically by using TPE. Experiments conducted on two distinct datasets demonstrate the superior performance achieved by this proposed mechanism.
更多查看译文
关键词
Chromosome images,Deep learning,Classification,Bayesian optimization,Tree-structured Parzen estimator
AI 理解论文
溯源树
样例
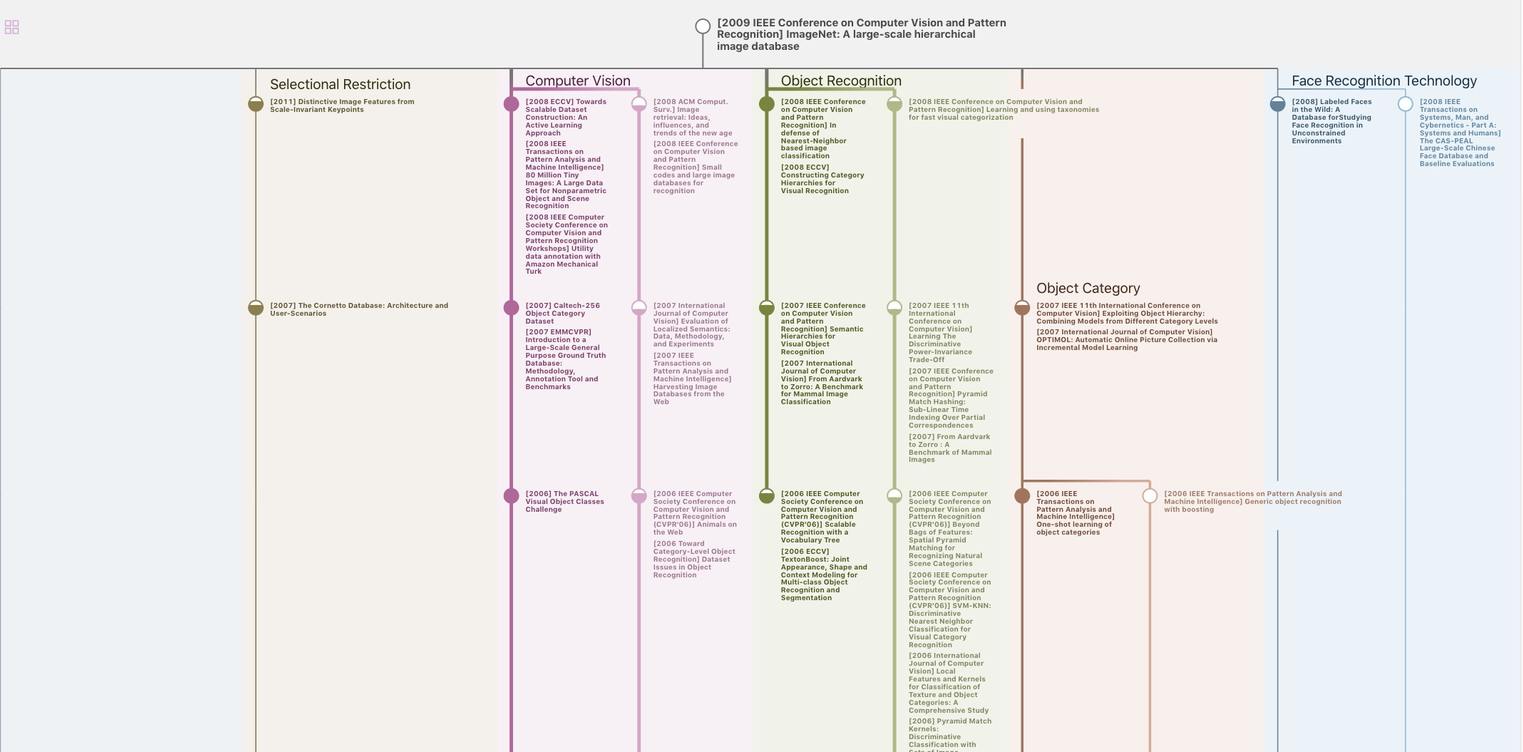
生成溯源树,研究论文发展脉络
Chat Paper
正在生成论文摘要