EquiAV: Leveraging Equivariance for Audio-Visual Contrastive Learning
ICML 2024(2024)
Abstract
Recent advancements in self-supervised audio-visual representation learning have demonstrated its potential to capture rich and comprehensive representations. However, despite the advantages of data augmentation verified in many learning methods, audio-visual learning has struggled to fully harness these benefits, as augmentations can easily disrupt the correspondence between input pairs. To address this limitation, we introduce EquiAV, a novel framework that leverages equivariance for audio-visual contrastive learning. Our approach begins with extending equivariance to audio-visual learning, facilitated by a shared attention-based transformation predictor. It enables the aggregation of features from diverse augmentations into a representative embedding, providing robust supervision. Notably, this is achieved with minimal computational overhead. Extensive ablation studies and qualitative results verify the effectiveness of our method. EquiAV outperforms previous works across various audio-visual benchmarks. The code is available on https://github.com/JongSuk1/EquiAV
MoreTranslated text
AI Read Science
Must-Reading Tree
Example
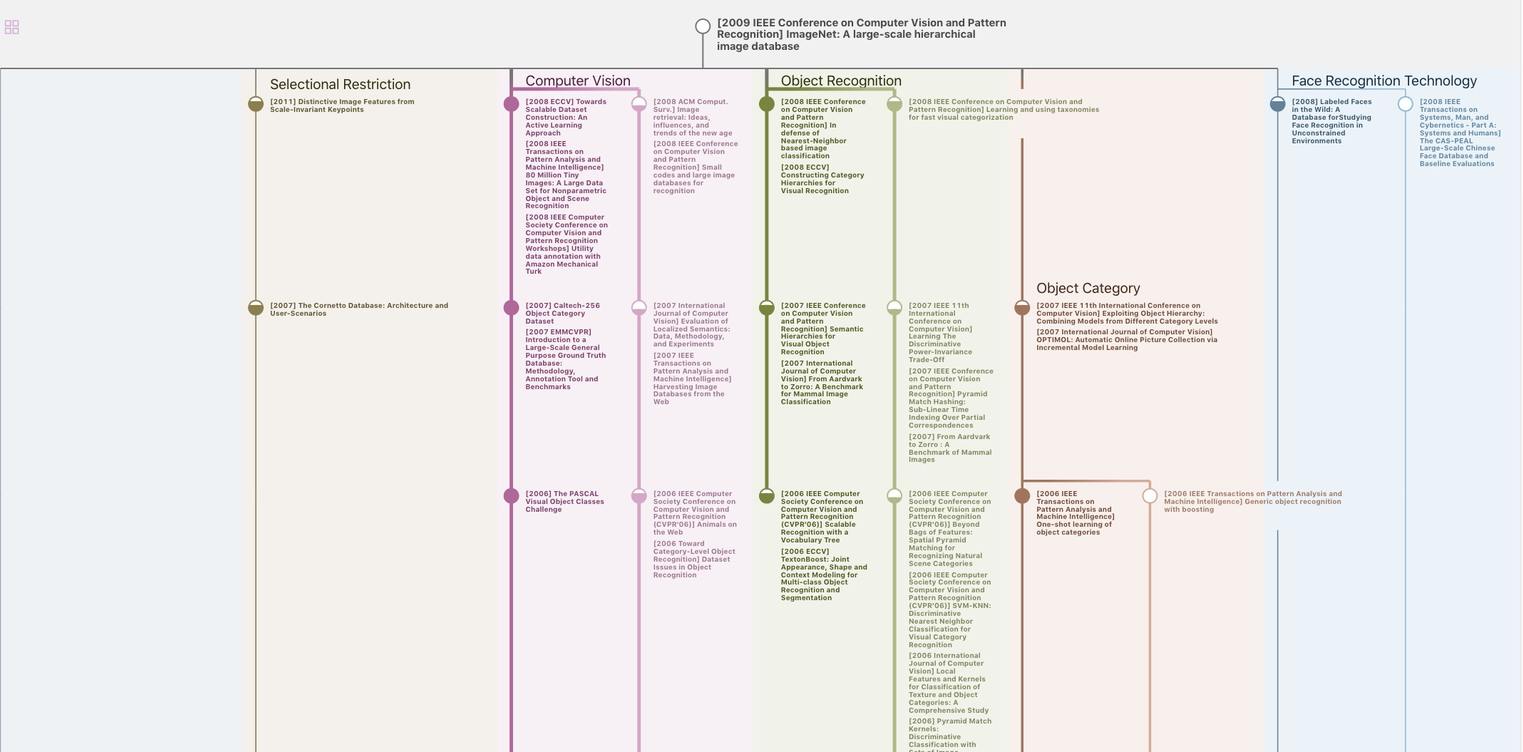
Generate MRT to find the research sequence of this paper
Chat Paper
Summary is being generated by the instructions you defined