Is Data All That Matters? The Role of Control Frequency for Learning-Based Sampled-Data Control of Uncertain Systems
CoRR(2024)
摘要
Learning models or control policies from data has become a powerful tool to
improve the performance of uncertain systems. While a strong focus has been
placed on increasing the amount and quality of data to improve performance,
data can never fully eliminate uncertainty, making feedback necessary to ensure
stability and performance. We show that the control frequency at which the
input is recalculated is a crucial design parameter, yet it has hardly been
considered before. We address this gap by combining probabilistic model
learning and sampled-data control. We use Gaussian processes (GPs) to learn a
continuous-time model and compute a corresponding discrete-time controller. The
result is an uncertain sampled-data control system, for which we derive robust
stability conditions. We formulate semidefinite programs to compute the minimum
control frequency required for stability and to optimize performance. As a
result, our approach enables us to study the effect of both control frequency
and data on stability and closed-loop performance. We show in numerical
simulations of a quadrotor that performance can be improved by increasing
either the amount of data or the control frequency, and that we can trade off
one for the other. For example, by increasing the control frequency by 33
can reduce the number of data points by half while still achieving similar
performance.
更多查看译文
AI 理解论文
溯源树
样例
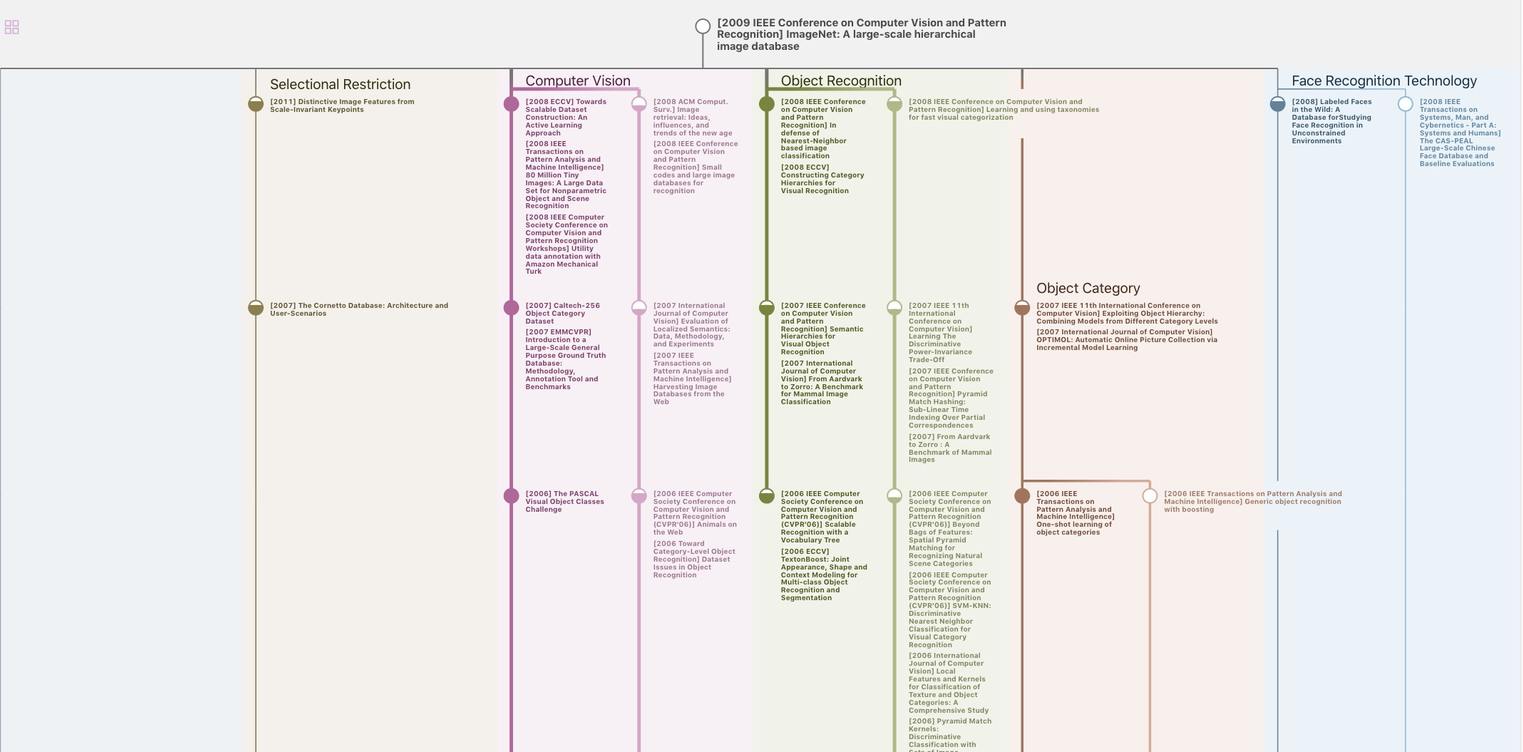
生成溯源树,研究论文发展脉络
Chat Paper
正在生成论文摘要