An End-to-End Bilateral Network for Multidefect Detection of Solid Propellants.
IEEE Trans. Ind. Informatics(2024)
摘要
Defect detection tasks of solid propellants (SPs), involving size, shape, and surface defects, are essential for ensuring the quality of many industrial products. Developing separate models for three tasks, however, is complicated and inefficient due to the redundant deployment. Multitask learning (MTL), with its potential for knowledge sharing, may greatly reduce the space and power consumption, but still faces the challenges of destructive interference and empirical tradeoffs between tasks. To this end, a novel end-to-end network for multidefect detection of SPs is put forth: 1) a new setting for MTL without any empirical tradeoffs is introduced, in which the knowledge is shared while the models are not visible to each other among diverse tasks; 2) in this setting, a bilateral feature extractor is constructed to extract both low- and high-level features, and a feature fusion module is further exploited to encourage each task to adaptively learn the task-specific knowledge; 3) an end-to-end training manner with a dynamic balance strategy and a gradient stop-flow strategy is designed to ensure that different tasks can benefit from, but do not interfere with, each other; 4) the introduction of semantic knowledge from the size detection branch enables the surface detection branch to learn semantic features beyond only pixel-to-pixel mapping. A smoothness construction loss is further designed to boost the performance of the surface detection task. Experimental results on an image dataset from a real-world manufacturing line show that the setting for MTL has the superiority in terms of the model size, inference speed, and detection accuracy.
更多查看译文
AI 理解论文
溯源树
样例
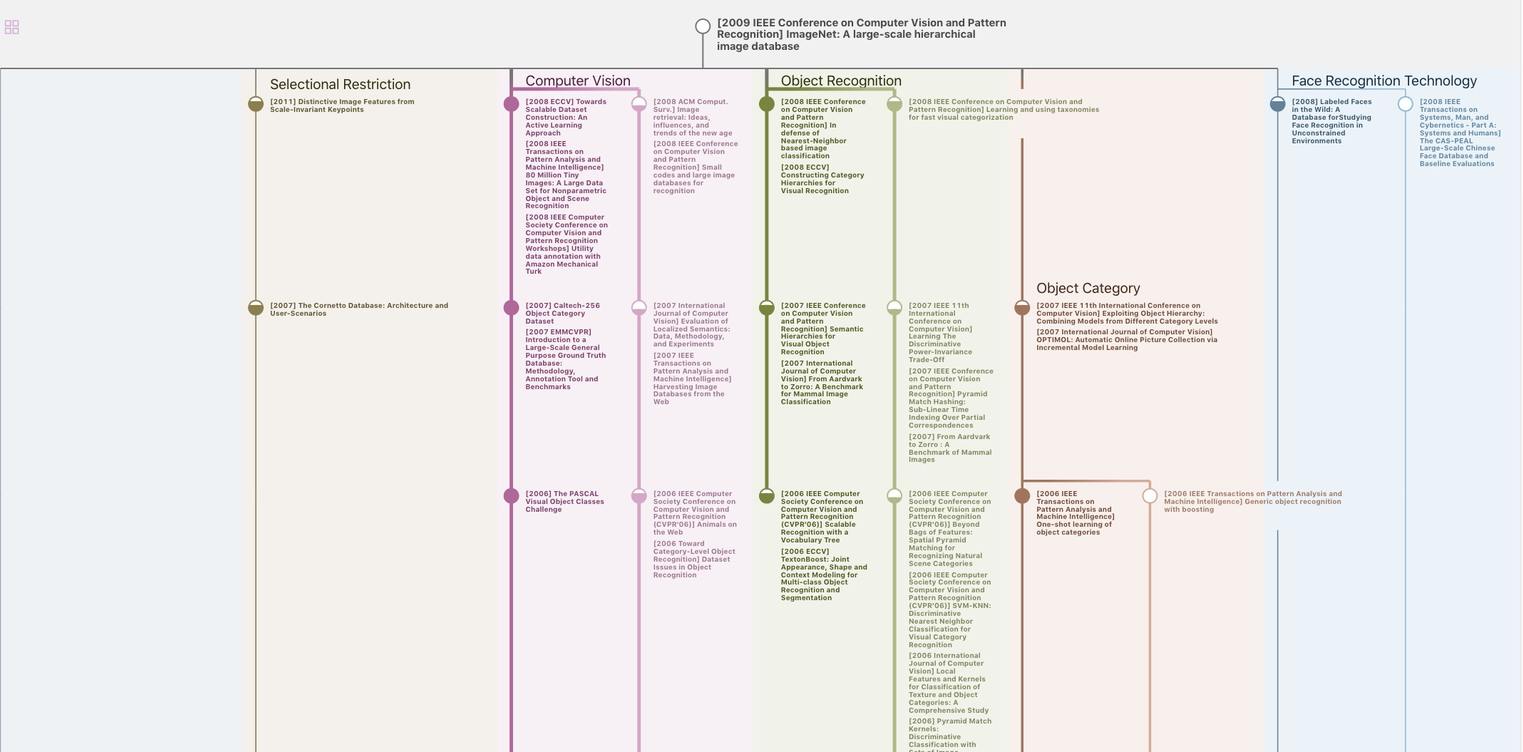
生成溯源树,研究论文发展脉络
Chat Paper
正在生成论文摘要