Few-Shot Learning for Crop Mapping from Satellite Image Time Series
REMOTE SENSING(2024)
摘要
Recently, deep learning methods have achieved promising crop mapping results. Yet, their classification performance is constrained by the scarcity of labeled samples. Therefore, the development of methods capable of exploiting label-rich environments to classify crops in label-scarce environments using only a few labeled samples per class is required. Few-shot learning (FSL) methods have achieved this goal in computer vision for natural images, but they remain largely unexplored in crop mapping from time series data. In order to address this gap, we adapted eight FSL methods to map infrequent crops cultivated in the selected study areas from France and a large diversity of crops from a complex agricultural area situated in Ghana. The FSL methods are commonly evaluated using class-balanced unlabeled sets from the target domain data (query sets), leading to overestimated classification results. This is unrealistic since these sets can have an arbitrary number of samples per class. In our work, we used the Dirichlet distribution to model the class proportions in few-shot query sets as random variables. We demonstrated that transductive information maximization based on alpha-divergence (alpha-TIM) performs better than the competing methods, including dynamic time warping (DTW), which is commonly used to tackle the lack of labeled samples. alpha-TIM achieved, for example, a macro F1-score of 59.6% in Ghana in a 24-way 20-shot setting (i.e., 20 labeled samples from each of the 24 crop types) and a macro F1-score of 75.9% in a seven-way 20-shot setting in France, outperforming the second best-performing methods by 2.7% and 5.7%, respectively. Moreover, alpha-TIM outperformed a baseline deep learning model, highlighting the benefits of effectively integrating the query sets into the learning process.
更多查看译文
关键词
crop mapping,few-shot learning,deep learning,transfer learning,time series
AI 理解论文
溯源树
样例
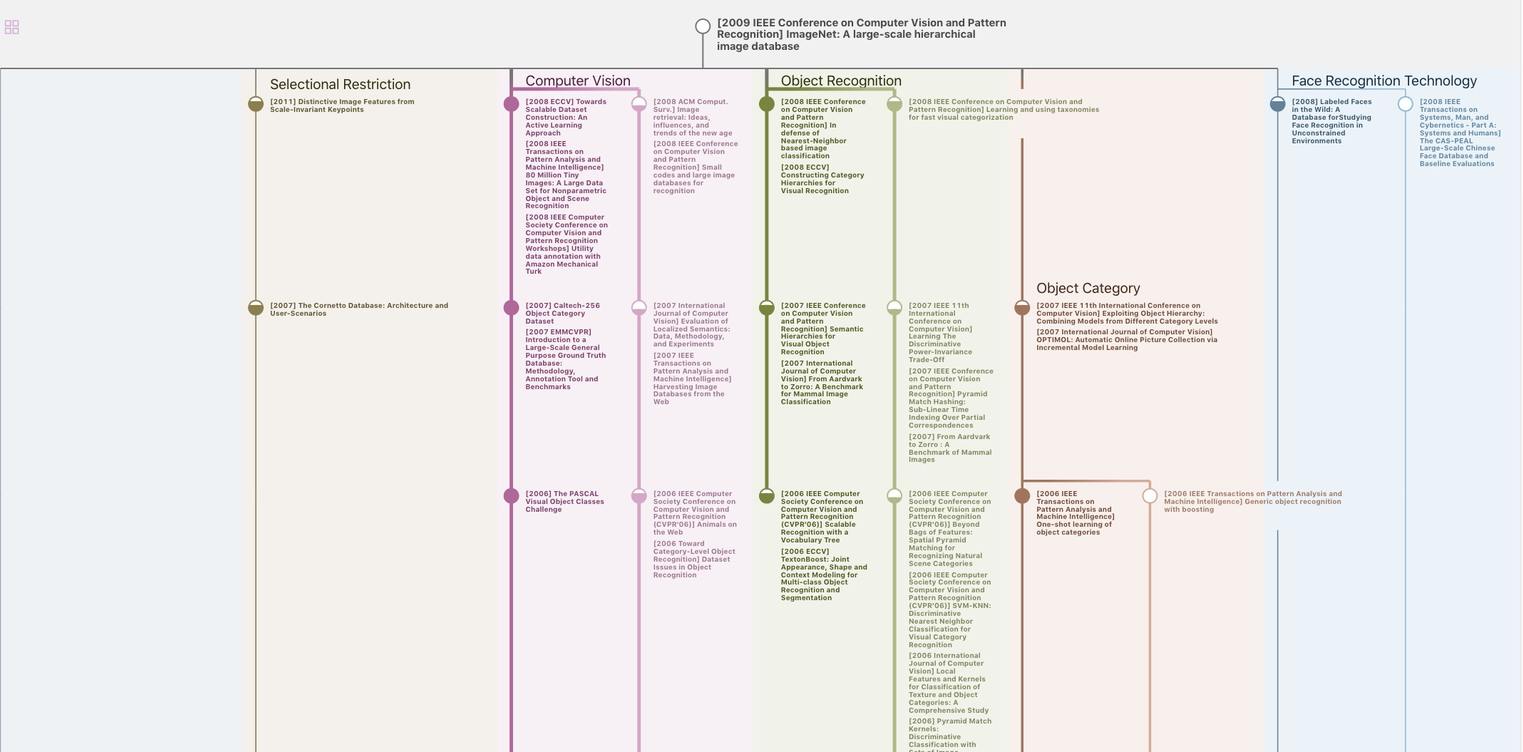
生成溯源树,研究论文发展脉络
Chat Paper
正在生成论文摘要