Iteratively Reweighted Hypergraph Subspace Clustering.
CSAI(2023)
摘要
With the development and application of hypergraphs, many hypergraph-based subspace clustering methods have been proposed by designing unique hypergraph construction based on the specific subspace representation model. However, there is no unified method to introduce hypergraphs into traditional simple graph-based subspace clustering methods to improve their clustering performance. To this end, this paper proposes an iteratively reweighted hypergraph subspace clustering framework (IRHSC), where the hypergraph is constructed unified based on representation coefficients. The hyperedge is generated according to a neighborhood strategy by taking each data point as a centroid vertex and linking to its coefficient-based K-nearest-neighbors adaptively selected via the rule of energy ratio θ. The weight is calculated through an iteratively reweighted method that continuously modifies the weights until a satisfactory weight is obtained. The adjustable ratio θ and continuously revised weights make the proposed framework IRHSC suitable for various subspace representation models and provide improvement effects. Experiments demonstrate the superiority of the framework IRHSC.
更多查看译文
AI 理解论文
溯源树
样例
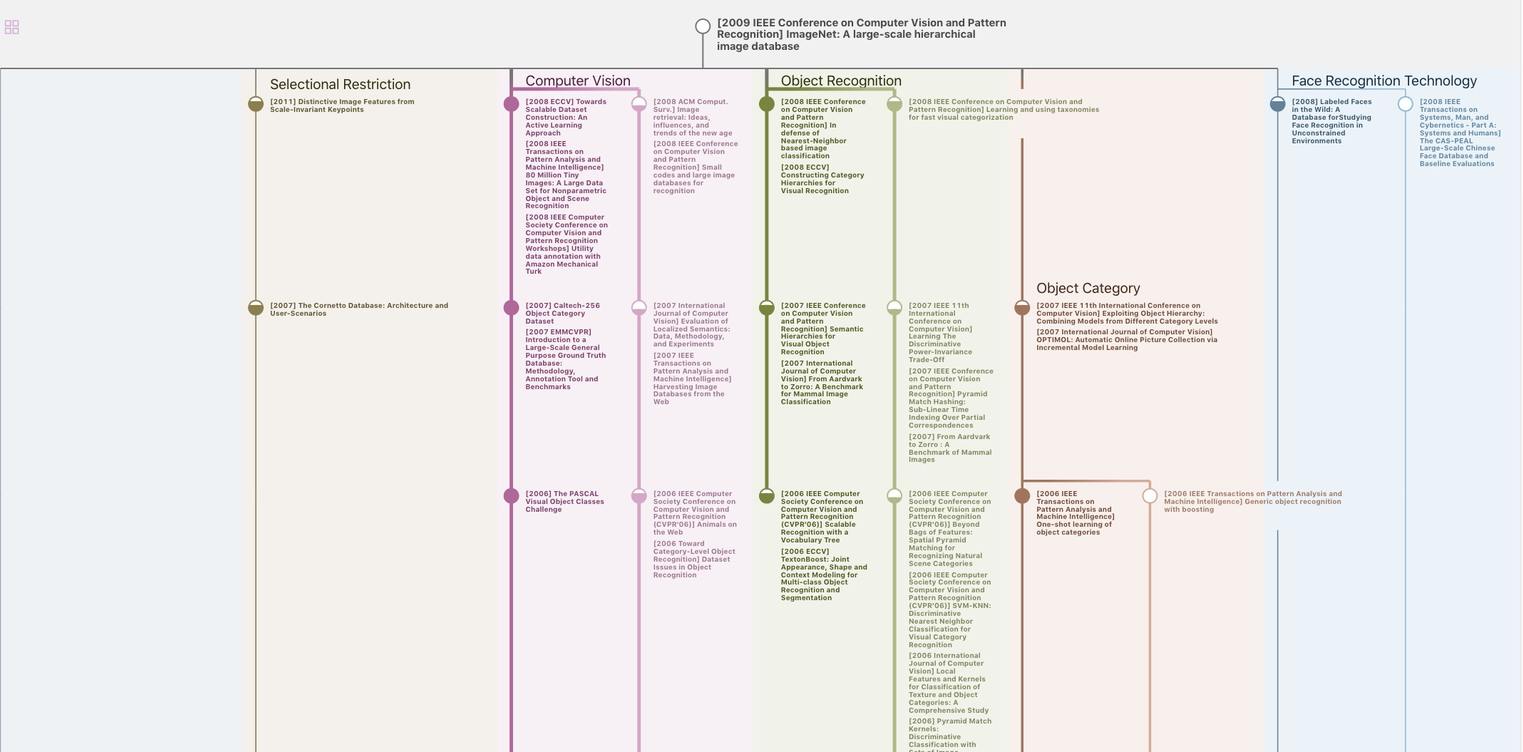
生成溯源树,研究论文发展脉络
Chat Paper
正在生成论文摘要