An enhanced automated machine learning model for optimizing cycle-to-cycle variation in hydrogen-enriched methanol engines
Applied Energy(2024)
摘要
Renewable methanol and hydrogen have emerged as great potential alternative fuels for internal combustion engines in recent research. Hydrogen exhibits the ability to mitigate cycle-to-cycle variations in methanol engines thanks to its rapid combustion rate. Furthermore, the integration of machine learning with genetic algorithms provides a solution to achieve efficient and clean combustion in hydrogen-enriched methanol engines. Nevertheless, this approach is constrained due to the limited predictive accuracy in small-sample learning. This study aims at introducing an enhanced model based on the automated machine learning framework, and provide a comparative analysis of the enhanced model and computational fluid dynamics simulations in terms of multi-objective optimization performance and computational time. Additionally, this study further considered the effect of engine cycle-to-cycle variations on performance, ultimately attaining optimal operating parameters for stable combustion. The results indicate that datasets actively created by genetic algorithms are more effective than those generated through Latin hypercube sampling. The introduction of a classification model for the identification of misfires has enhanced the efficiency of dataset utilization. Through feature construction, the automated machine learning model demonstrates improvements in R-square and mean-squared error for all parameters on the test dataset. Furthermore, by employing a larger overall population size, the automated machine learning model has not only achieved a higher-quality Pareto fronts compared to the computational fluid dynamics method but has also significantly reduced computation time, particularly upon introducing additional engine cycle-to-cycle variations constraints.
更多查看译文
关键词
Methanol,Machine learning,Genetic algorithm,Cycle-to-cycle variations
AI 理解论文
溯源树
样例
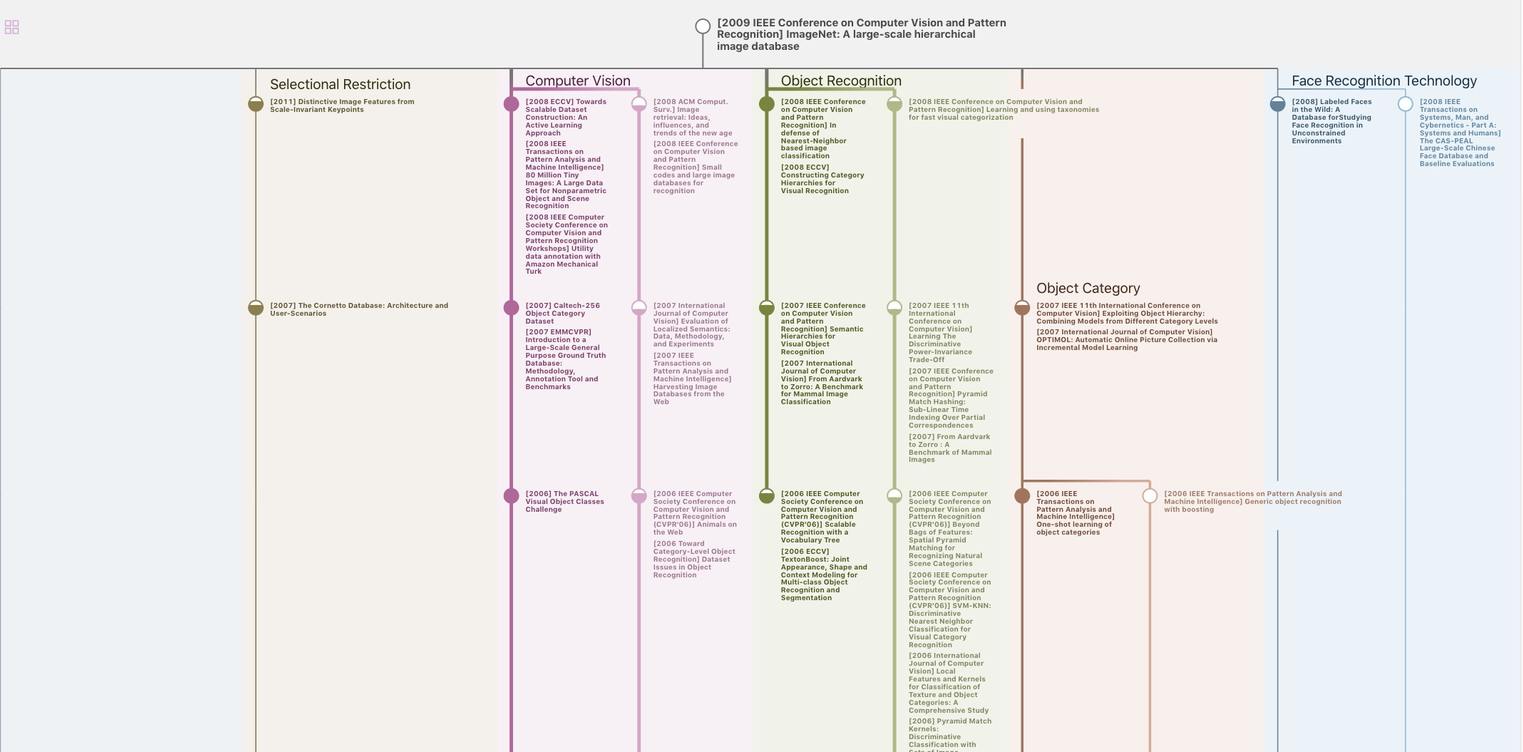
生成溯源树,研究论文发展脉络
Chat Paper
正在生成论文摘要