Machine learning based human mental state classification using wavelet packet decomposition-an EEG study
Multimedia Tools and Applications(2024)
摘要
Stress is a crucial factor that causes various health-related issues. Recent research has focused on stress prediction using Electroencephalography (EEG) signal processing. Early detection of stress among the students helps to avoid suicidal thoughts and illness, also proper counseling is given to improve the learning ability of the students. To improve the performance metrics of the classifier model, EEG features such as relative sub-band energies and EEG band ratios were considered. In this study, two levels of classification such as stress and non-stress states were carried out using machine learning techniques. An experimental work with EEG signal acquired from 25 subjects under two conditions as relax mode (non-stress) and during a mental task (stress) using an 8-channel wireless Enobio device. EEG features extracted using discrete wavelet transform technique, relative sub-band energy such as alpha, theta, and beta energies, and the relative band ratios computed from sub-band energies for two states such as arousal index, heart rate, performance enhancement index, cognitive performance attentional resource index (CPARI), CNS arousal and desynchronization. EEG Features were selected by analyzing statistically significant (p < 0.05) for both states of data by using a non-parametric test as the Wilcoxon signed-rank test, and brain functional connectivity analysis was carried out for subband energies. Then, the extracted features were imported to various machine learning classifier algorithms such as decision tree, linear discriminant analysis, Naïve Bayes, Support Vector Machine (SVM), k-Nearest Neighbor (kNN), ensemble, and neural network. The classifier performance metrics such as classification accuracy, sensitivity, specificity, and precision were compared for the above classifiers. The experimental result shows that the cubic SVM classifier has achieved the highest accuracy of 95.83
更多查看译文
关键词
Electroencephalography (EEG),Performance enhancement index,Cognitive performance attentional resource index (CPARI),SVM,kNN,Neural network
AI 理解论文
溯源树
样例
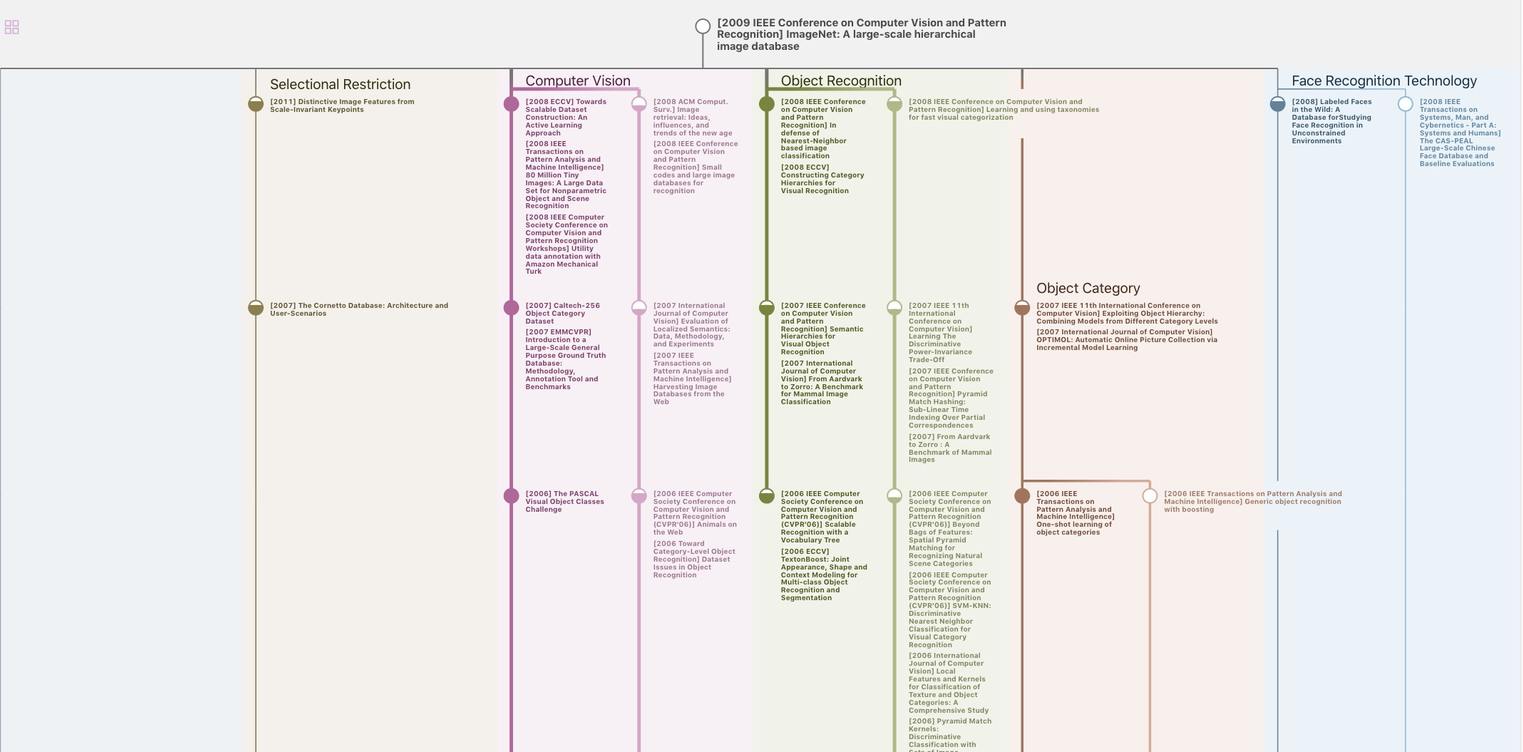
生成溯源树,研究论文发展脉络
Chat Paper
正在生成论文摘要