Developing Machine Learning Algorithms Incorporating Patient Reported Outcomes to Predict Disease Progression in Head and Neck Cancers
International Journal of Radiation Oncology*Biology*Physics(2024)
Abstract
Purpose/Objective(s)
Patient reported outcome (PRO) is gaining traction for implementation into clinical practice within electronic medical record systems. Recent randomized controlled trials support standardized PRO-base monitoring in enhancing health-related quality of life, reducing emergency department use and improving overall survival. Here, we examined whether a validated head and neck cancer (HNC) specific PRO or general PRO can better predict disease progression using machine learning algorithms.
Materials/Methods
This was a retrospective analysis of a prospective observational study through the Comprehensive Translational Research Study of Head and Neck Tumors at our institution where baseline clinical, demographic and PROMs were collected between 2007 and 2018. Patients with a primary HNC, who had at least one MD Anderson Symptom Inventory (MDASI) or Edmonton Symptom Assessment Score (ESAS) questionnaire collected 3 months after curative intents treatment were included. Models were fit to predict disease progression three months following a post-treatment MDASI or ESAS questionnaire. Eight models were generated using supervised machine learning: least absolute shrinkage and selection operator (LASSO) logistic regression and random forest models. Predictive performances were assessed via area under the receiver-operating curve, computed with 10-fold cross-validation. Four of the models were based on first post-treatment PRO, while the others on change over time in PRO scores. Relative variable importance was computed with average decrease in out-of-bag prediction accuracy of each tree.
Results
We included 1302 HNC patients, of whom 527 (40%) were HPV-positive, and 556 (43%) were from oropharynx. Disease progression occurred in 123 (9.5%) of HNC patients. With baseline post-treatment MDASI, our best random forest model demonstrated an area under the curve (AUC) approximating 0.675. The top three variables were pain, speech and dry mouth. When comparing change in MDASI scores over time, random forest models again performed the best, with an AUC of 0.676. The top three variables were pain, choking and fatigue. Models incorporating MDASI were more predictive for disease progression than ESAS.
Conclusion
Machine-learning approaches on head and neck cancer specific patient reported outcomes can identify patients at high risk for disease progression with high sensitivity. Future work will involve evaluating whether implementing predictive models using MDASI with targeted clinical interventions can improve clinical outcomes.
MoreTranslated text
AI Read Science
Must-Reading Tree
Example
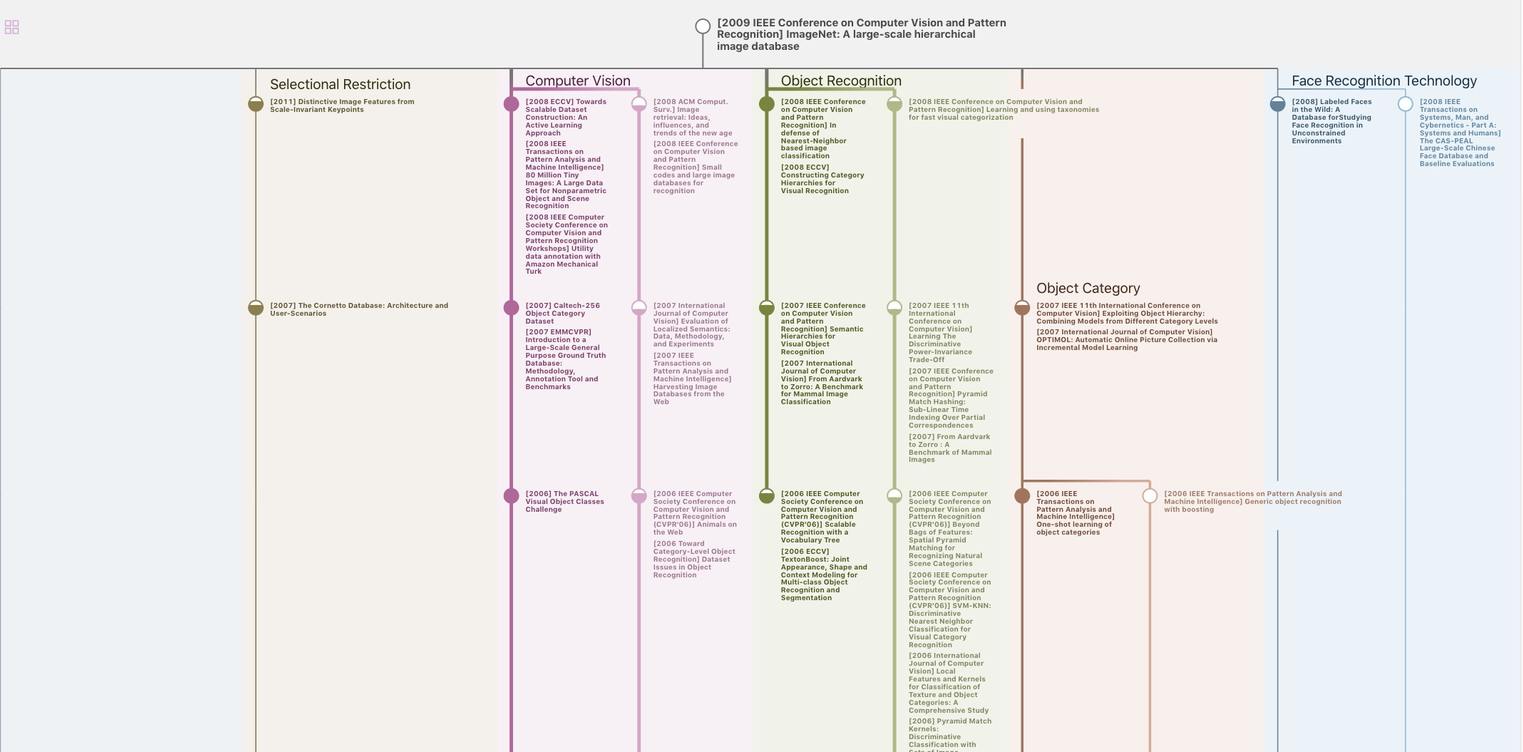
Generate MRT to find the research sequence of this paper
Chat Paper
Summary is being generated by the instructions you defined