Enhancing Qualitative Microwave Imaging Indicators by Autoencoder Based Deep Learning Algorithm
crossref(2024)
摘要
Abstract Enhancing microwave inverse imaging methods has garnered significant attention in the field of deep learning and microwave imaging, owing to their immense potential in tackling non-linear problems. Microwave imaging techniques commonly encounter the adverse effects of noise in measurements, leading to suboptimal results. In this research, we propose a novel deep neural network-based model that effectively mitigates the noise effects on qualitative microwave indicators, resulting in high-quality reconstructions. We consider various configurations of dielectric objects in free space to address the microwave imaging problem. The scattered field is computed using the two-dimensional method of moments, employing an antenna array comprising 36 infinitely long line sources. Subsequently, the linear sampling method is utilized to generate multi-frequency qualitative indicators, which are then employed as input to a deep learning network along with corresponding binary ground-truth images. The proposed deep learning model comprises 11 layers, including convolutional and deconvolutional layers, without any dropout or pooling layers to prevent information loss. The results of the network are compared with Jaccard index to see how similar they are. The proposed method achieves higher accuracy and Jaccard score, demonstrating the efficacy of the proposed deep learning approach. Notably, the simplicity of the model construction contributes to its appeal, as it operates in conjunction with the results obtained from the linear sampling method. The presented results underscore the remarkable capabilities of the proposed deep learning method in enhancing microwave inverse imaging.
更多查看译文
AI 理解论文
溯源树
样例
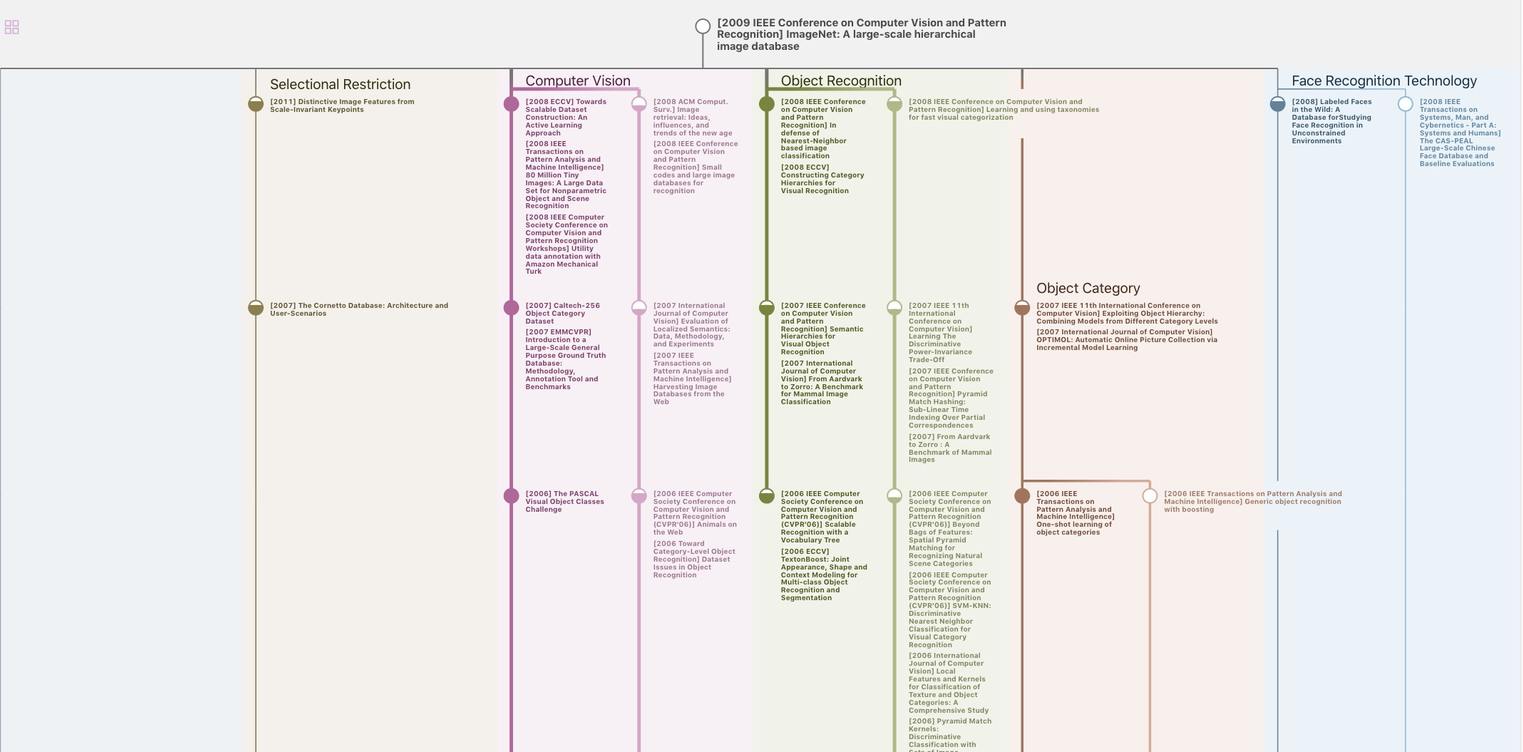
生成溯源树,研究论文发展脉络
Chat Paper
正在生成论文摘要