ASPTF: A computational tool to predict abiotic stress-responsive transcription factors in plants by employing machine learning algorithms
Biochimica et Biophysica Acta (BBA) - General Subjects(2024)
摘要
Background
Abiotic stresses pose serious threat to the growth and yield of crop plants. Several studies suggest that in plants, transcription factors (TFs) are important regulators of gene expression, especially when it comes to coping with abiotic stresses. Therefore, it is crucial to identify TFs associated with abiotic stress response for breeding of abiotic stress tolerant crop cultivars.
Methods
Based on a machine learning frame work, a computational model was envisaged to predict TFs associated with abiotic stress response in plants. To numerically encode TF sequences, four distinct sequence derived features were generated. The prediction was performed using ten shallow learning and four deep learning algorithms. For prediction using more pertinent and informative features, feature selection techniques were also employed.
Results
Using the features chosen by the light-gradient boosting machine-variable importance measure (LGBM-VIM), the LGBM achieved the highest cross-validation performance metrics (accuracy: 86.81%, auROC: 92.98%, and auPRC: 94.03%). Further evaluation of the proposed model (LGBM prediction method + LGBM-VIM selected features) was also done using an independent test dataset, where the accuracy, auROC and auPRC were observed 81.98%, 90.65% and 91.30%, respectively.
Conclusions
To facilitate the adoption of the proposed strategy by users, the technique was implemented and made available as a prediction server called ASPTF, accessible at https://iasri-sg.icar.gov.in/asptf/. The developed approach and the corresponding web application are anticipated to complement experimental methods in the identification of transcription factors (TFs) responsive to abiotic stress in plants.
更多查看译文
关键词
Transcription factor,Machine learning,Abiotic stress,Computational model,Bioinformatics
AI 理解论文
溯源树
样例
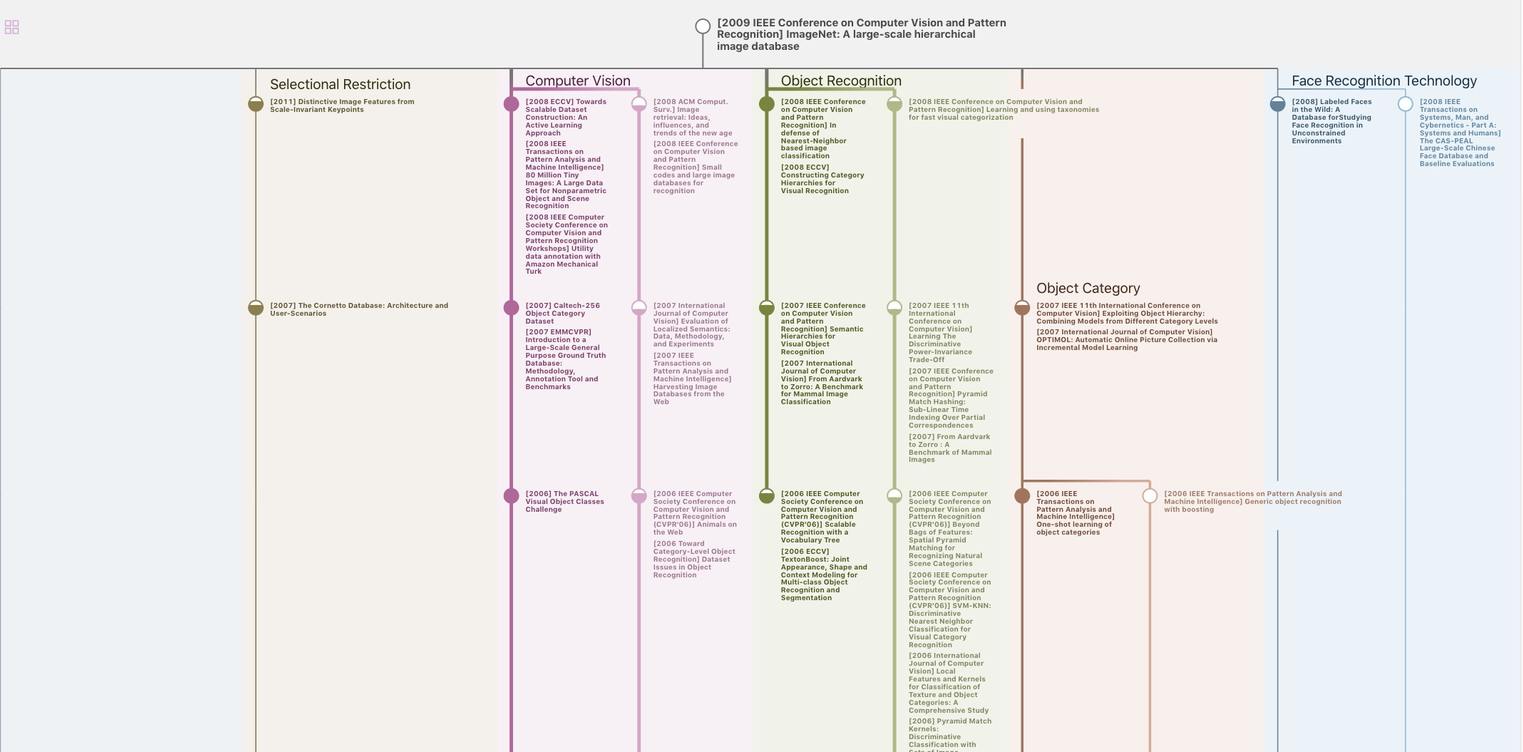
生成溯源树,研究论文发展脉络
Chat Paper
正在生成论文摘要