Virtual metrology for chemical mechanical planarization of semiconductor wafers
Journal of Intelligent Manufacturing(2024)
摘要
Chemical mechanical planarization (CMP) is an important operation for surface modification of wafers in semiconductor manufacturing. Productivity and quality of wafers depends strongly on the efficiency of CMP and virtual metrology (VM) is a promising tool not only to facilitate wafer-to-wafer control but also to reduce cycle time. Development of VM tools for CMP is still not a reality due to the complexity of CMP and unavailability of critical process measurements such as slurry temperature and abrasive particle size distribution in real-time. To overcome these challenges, a novel hybrid modeling framework is proposed for creating a VM solution for CMP. Physics-based models are utilized for estimating slurry temperature and mean abrasive particle size (MAPS) from sensor data. They supplement other sensor data for developing soft sensors to predict slurry temperature, MAPS, and the material removal rate (MRR). This hybrid framework is tested with about 3000 sets of published industrial sensor data. Exploratory analysis indicated two distinct regimes of operation, low and high MRR, and a strong relationship of MRR with slurry temperature and MAPS. Several machine learning (ML) algorithms such as random forest, Lasso regression and support vector machine are explored and XGBoost is found to be the best amongst them. The optimum operating conditions are determined through model-based optimization using the hybrid modeling framework and particle swarm optimization. These results suggested CMP to be carried out at the smallest MAPS to maximize MRR. This framework would be useful for building a digital twin system of CMP.
更多查看译文
关键词
Virtual metrology,Optimization,Soft sensors,Semiconductors,Machine learning
AI 理解论文
溯源树
样例
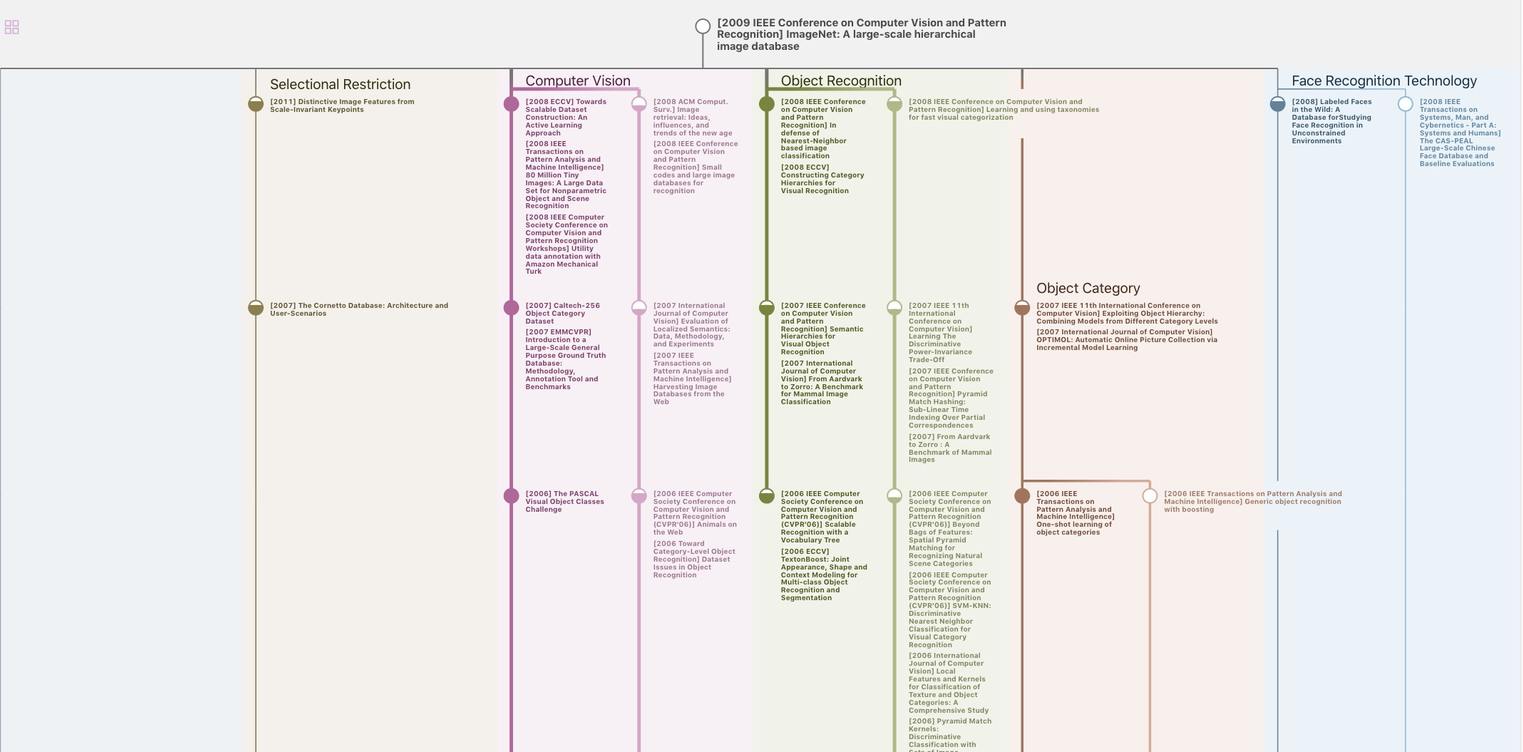
生成溯源树,研究论文发展脉络
Chat Paper
正在生成论文摘要