30.2 A 22nm 0.26nW/Synapse Spike-Driven Spiking Neural Network Processing Unit Using Time-Step-First Dataflow and Sparsity-Adaptive In-Memory Computing.
2024 IEEE International Solid-State Circuits Conference (ISSCC)(2024)
Abstract
Recently, brain-inspired spiking neural networks (SNNs) have demonstrated tremendous improvement in energy efficiency (EE) and low power by exploiting highly sparse spikes and event-driven design [1–2]. (top of Fig. 30.2.1) With the same spike-based information carrier, the combination of an SNN and dynamic vision sensor (DVS) [3] offers a promising solution for edge AI applications. Typically, the greater the number of synapses in an SNN can improve inference accuracy, but induce more significant power consumption and memory size. Therefore, deploying more synapses in an SNN chip while maintaining low power, memory size and high EE requires co-design of algorithm, architecture and circuits. At the algorithm level, SNN training based on conversion from an artificial NN (ANN) [1] achieves the best accuracy in static image classification, but at the cost of worse EE and higher power consumption, as it requires excessive time steps and energy arising from ANN-SNN conversion. At the architecture level, the multiple-time-step forward computation in SNNs introduces redundant data movement and storage, including input-output spikes, weights, partial sums and membrane potentials, restricting the network scale that can be accommodated by limited on-chip memory, i.e., network density (ND) [4–6]. At the circuit level, in-memory computing (IMC) is attractive to minimize data movement. However, traditional frame-based IMC circuits are incompatible with the highly sparse and fine-grained spike-driven nature of SNNs. Recent advancements in neuromorphic IMC [7] employ integrated-fire (IF) converters, which are globally triggered by power-hungry clocks even if there is no spike, resulting in high power per synapse, i.e., power density (PD).
MoreTranslated text
Key words
Computational Memory,Spiking Neural Networks,Time Step,Energy Efficiency,Power Consumption,Dense Network,Power Density,Load Data,Pulse Generator,Application Of Solution,Consumption Cost,Limited Memory,Top Right,Improve Energy Efficiency,Input Range,Partial Sums,Memory Size,High Power Consumption,Improvement In Power,Limited Computational Resources
AI Read Science
Must-Reading Tree
Example
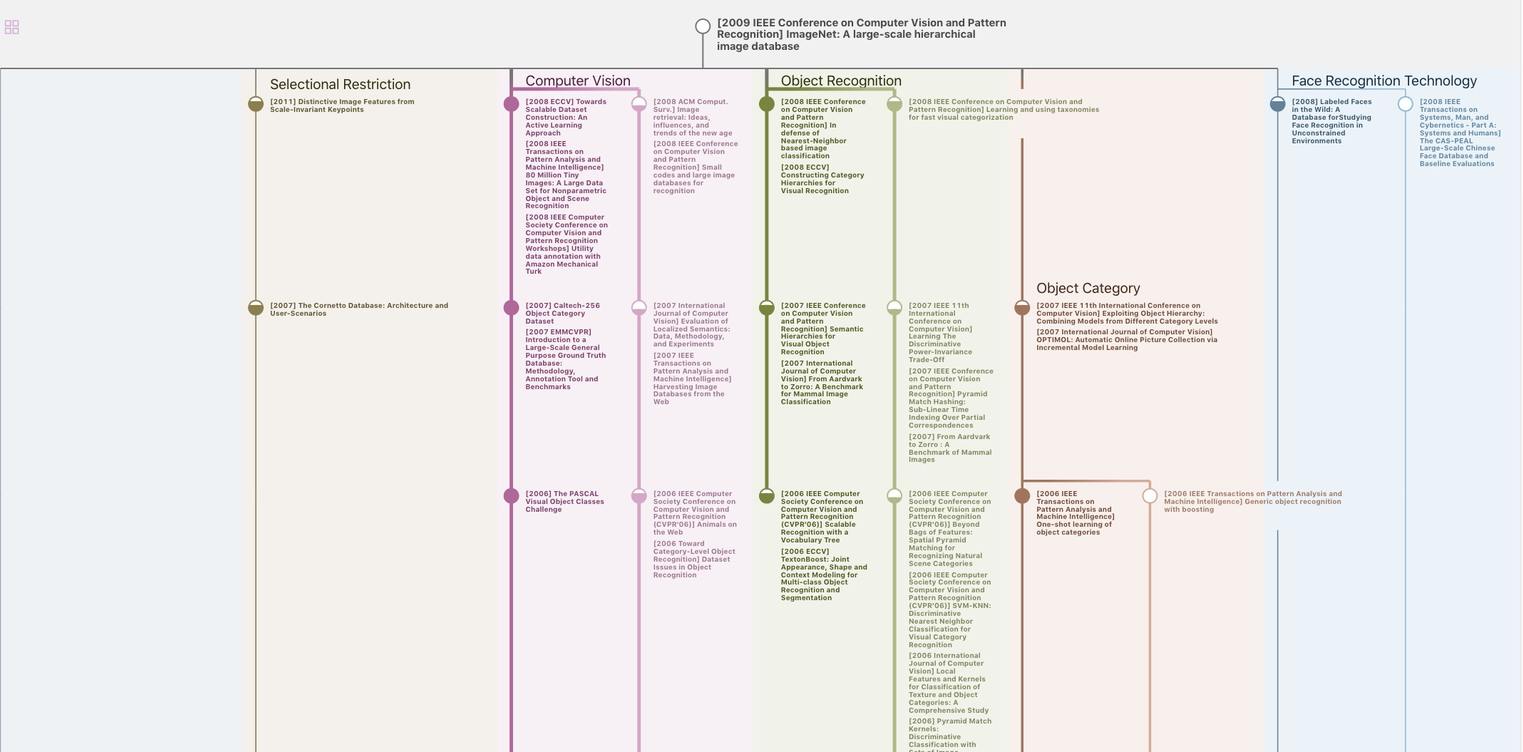
Generate MRT to find the research sequence of this paper
Chat Paper
Summary is being generated by the instructions you defined