Prediction of self-consolidating concrete properties using XGBoost machine learning algorithm: Rheological properties
POWDER TECHNOLOGY(2024)
摘要
Rheological properties are critical for assessing self-consolidating concrete (SCC)'s performance and application. However, predicting these properties accurately, specifically plastic viscosity and yield stress, faces challenges due to inconsistent data, small sample sizes, and measurement inaccuracies, with the type of rheometer significantly impacting results. This study meticulously analyzes 348 mixtures from 19 peer-reviewed sources, focusing on experiments that detail rheometer types to understand variability in rheological properties. Twelve variables, including cement content and water-to-powder ratio, were identified as key to SCC's rheology. Utilizing these, an XGBoost model demonstrated exceptional accuracy (R2 of 0.99), markedly better than traditional methods. This advance not only aids in SCC design but also showcases the potential of machine learning in construction materials research, suggesting a new direction for material property prediction and innovation in construction.
更多查看译文
关键词
Machine learning,Self -consolidating concrete,Rheological properties,Plastic viscosity,Yield stress
AI 理解论文
溯源树
样例
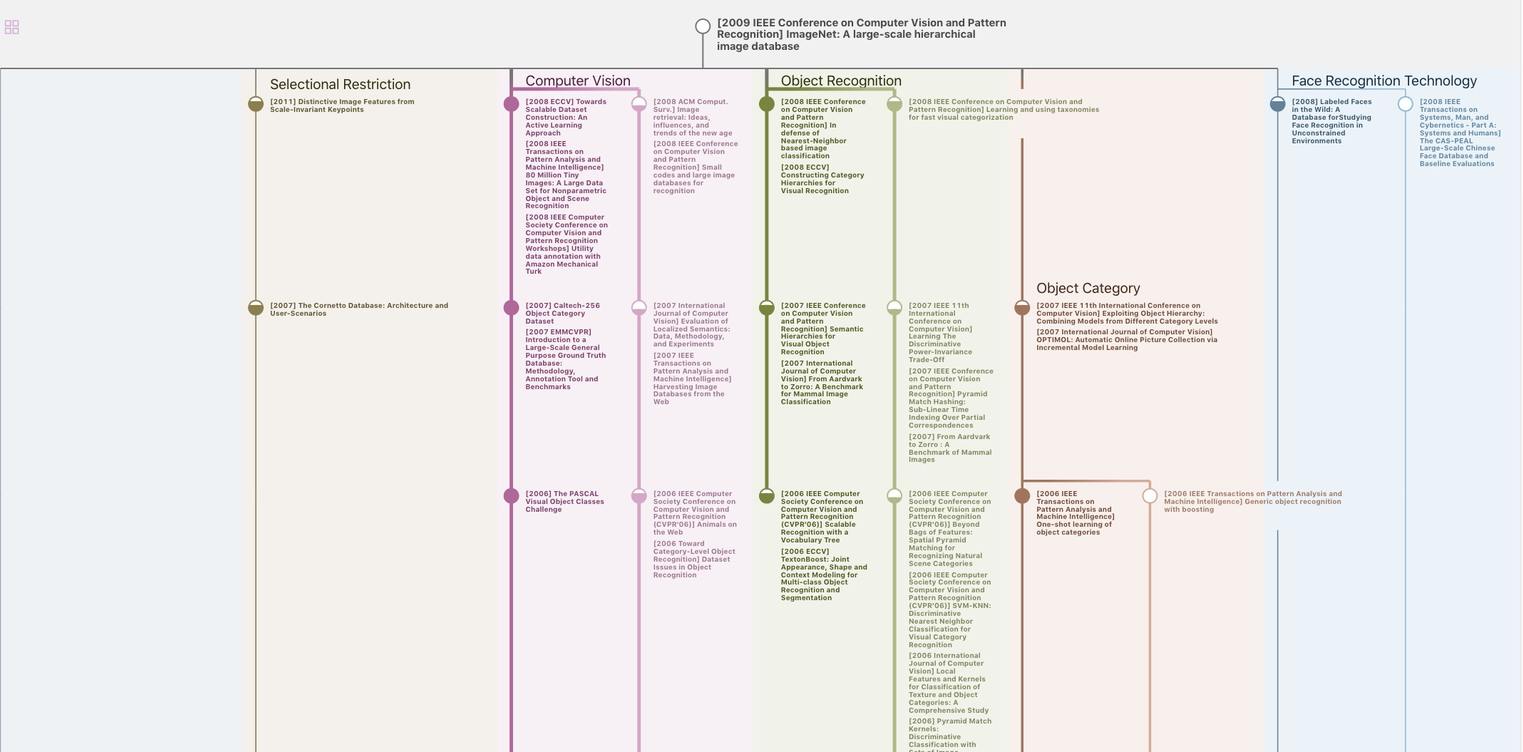
生成溯源树,研究论文发展脉络
Chat Paper
正在生成论文摘要