34.9 A Flash-SRAM-ADC-Fused Plastic Computing-in-Memory Macro for Learning in Neural Networks in a Standard 14nm FinFET Process.
IEEE International Solid-State Circuits Conference(2024)
摘要
AI edge devices are not only required to perform inference tasks with low power and high real-time performance but are also expected to have the capability to learn and adapt to dynamic and unpredictable environments, without heavily relying on cloud-based training. The recent rise of computing-in-memory (CIM) has offered a competent solution by minimizing the power and latency associated with data movement. While many existing CIM designs [1–6] have primarily focused on improving the performance of AI inference, those with learning abilities have, so far, been relatively less studied.
更多查看译文
关键词
Neural Network Learning,14nm FinFET,High Voltage,Sparsity,Learning Ability,Matrix Multiplication,Analog-to-digital Converter,Load Data,Parasitic Capacitance,Non-volatile Memory,Data Retention,Unpredictable Environments,Area Overhead,Inference Performance,Analog Domain,Charge Pump,Memory Window,Fine-tuning Step
AI 理解论文
溯源树
样例
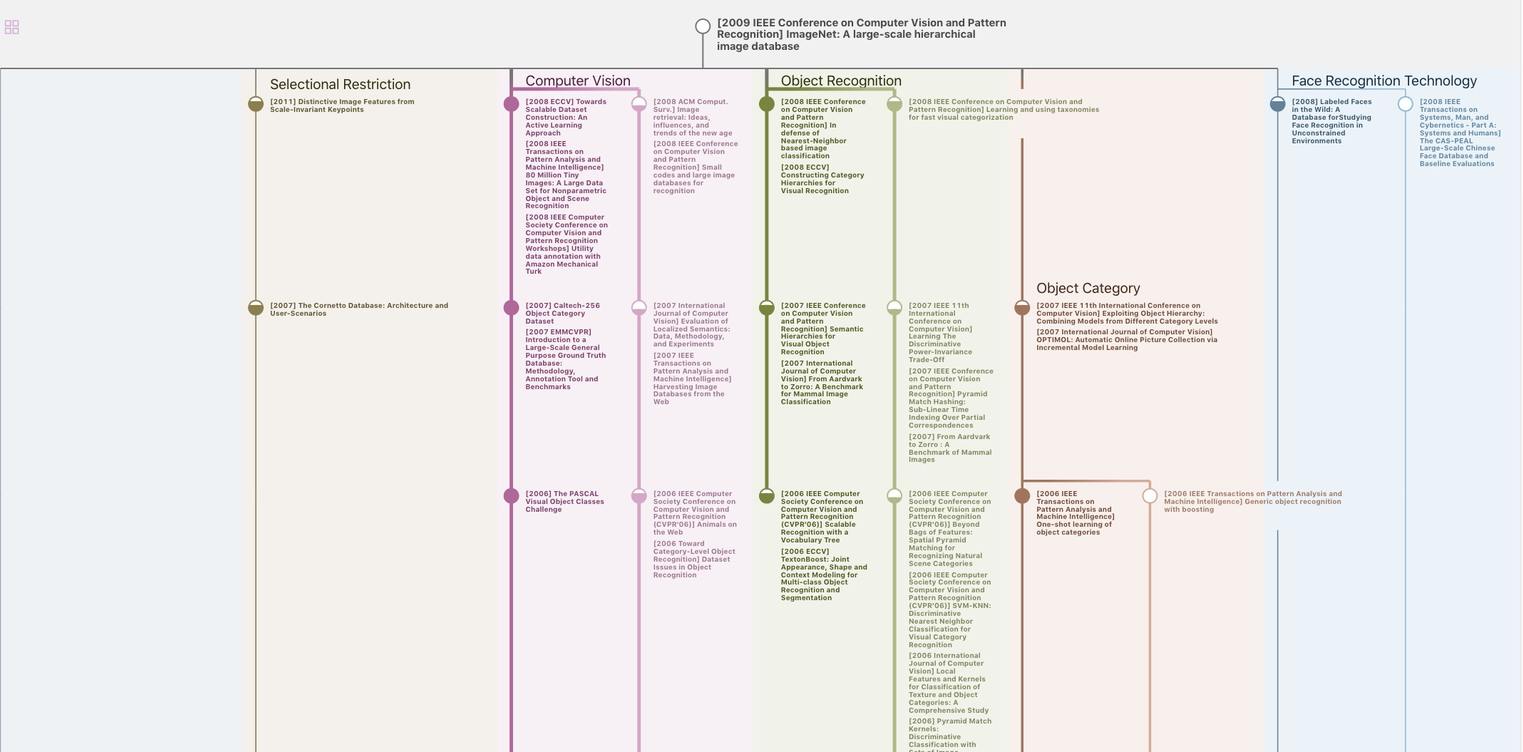
生成溯源树,研究论文发展脉络
Chat Paper
正在生成论文摘要