SASEGAN-TCN: Speech Enhancement Algorithm Based on Self-Attention Generative Adversarial Network and Temporal Convolutional Network
Mathematical Biosciences & Engineering(2024)
关键词
speech enhancement,deep learning,generative adversarial network,autoencoder
AI 理解论文
溯源树
样例
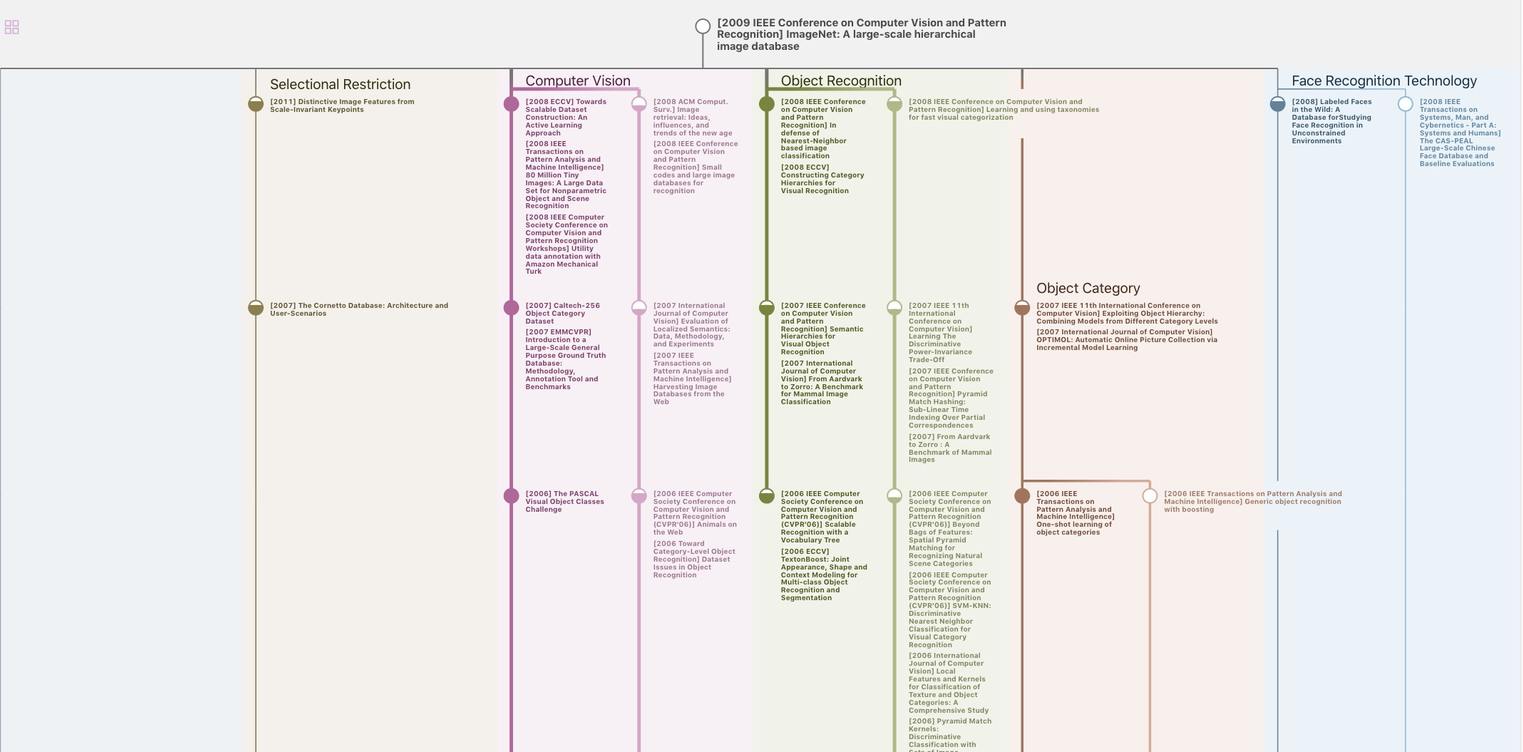
生成溯源树,研究论文发展脉络
Chat Paper
正在生成论文摘要