DPFLA: Defending Private Federated Learning Against Poisoning Attacks
IEEE Transactions on Services Computing(2024)
摘要
Federated learning (FL) is vulnerable to data poisoning attacks when an adversary attempts to upload poison gradients with the intent to corrupt the global model of FL. Various approaches have been proposed to counter these risks. However, it becomes challenging when one tries to preserve the privacy of FL participants and ensure robustness against data poisoning attacks. In this paper, we propose DPFLA, a novel scheme that can detect poisoning attacks without revealing the actual gradients of participants. DPFLA is a lossless aggregation scheme delicately designed for adopting masks to protect private data while extracting poisoned data features. Specifically, we first apply removable masks to the gradients outputted by each participant. Second, we aggregate the masked data and decompose them using Singular Value Decomposition (SVD) to extract specific features as well as achieve dimensionality reduction. Third, we leverage a clustering paradigm to detect poison gradients from the low dimension and eliminate them in the following training rounds. We conducted extensive experiments to demonstrate that DPFLA can detect poison gradients effectively. Additionally, the comparisons of case studies demonstrate that DPFLA outperforms the state-of-the-art methods.
更多查看译文
关键词
Federated learning,label flipping attacks,backdoor attacks,defense of poisoning attacks,singular value decomposition
AI 理解论文
溯源树
样例
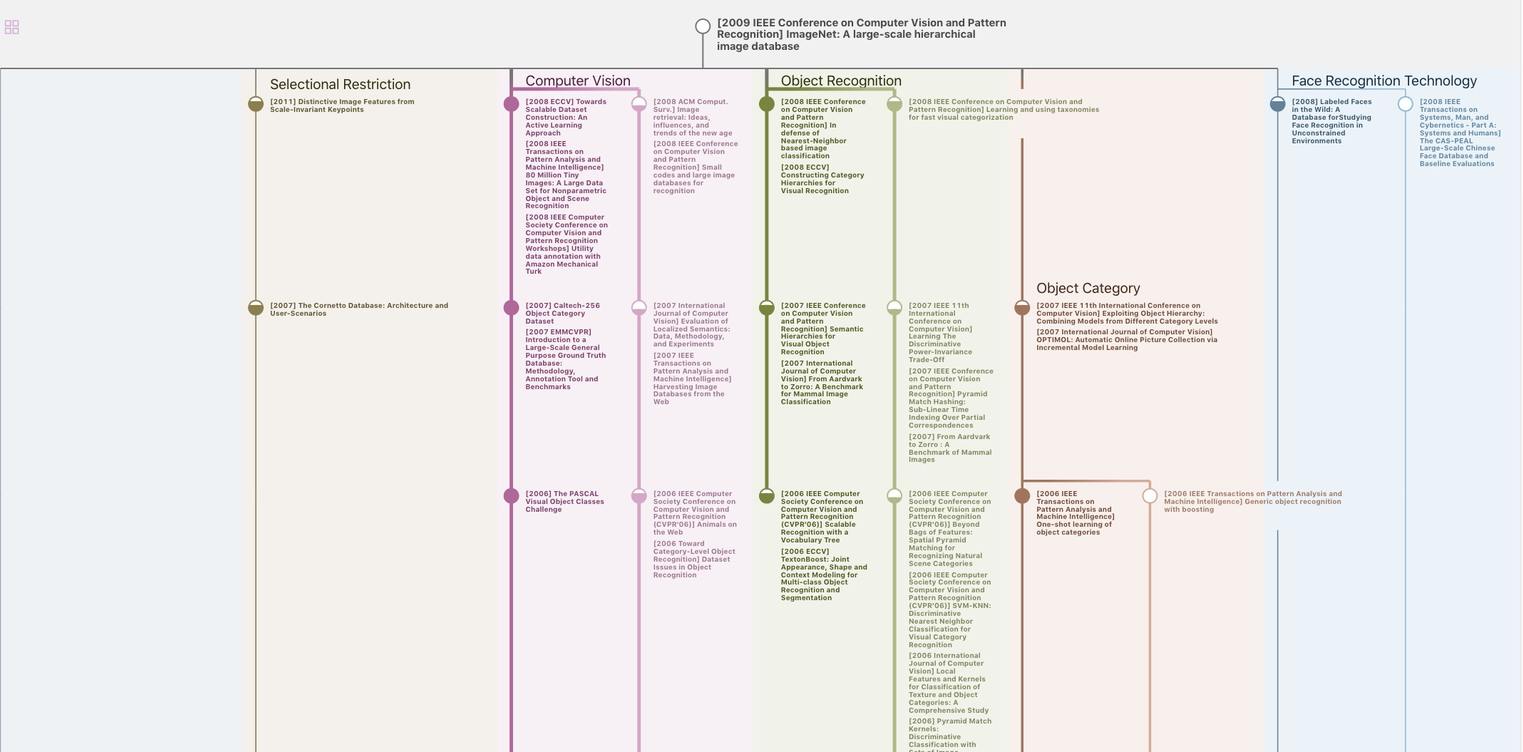
生成溯源树,研究论文发展脉络
Chat Paper
正在生成论文摘要