On autoregressive and neural methods for massive-MIMO channel de-noising
ITU Journal on Future and Evolving Technologies(2024)
Abstract
In modern wireless communication systems, the Multiple-Input Multiple-Output (MIMO) technology allows to greatly increase power efficiency, the serving area, and the overall cell throughput through the use of the antenna array beamforming. Nevertheless, the MIMO systems require accurate channel state knowledge to apply correct precoding. In 5G Time Division Duplex (TDD) systems, the Channel State Information (CSI) is obtained via Sounding Reference Signals (SRS) transmitted by the User Equipment (UE). UEs have limited power capabilities and thus cannot achieve high Uplink (UL) Signal-to-Noise Ratio (SNR) on gNodeB (gNB) in large bandwidth. There are multiple techniques that can be applied to improve the accuracy of Channel Estimation (CE) in noisy conditions. In this paper, we describe a classical method, namely the Vector Autoregression (VAR) with adaptive model order estimation, as well as a modern Deep Neural Network (DNN) approach for the massive-MIMO channel estimation de-noising problem. The developed methods and signal pre and postprocessing steps are described, followed by their performance evaluation in a set of realistic simulations. The designed algorithms provide results outperforming the baseline spatio-temporal windowing approaches by approximately equal to 2dB effective Downlink (DL) Signal-to-Interference-plus-Noise Ratio (SINR) metric in single and multi-user MIMO scenarios. Extensive simulation results demonstrate the robustness of the developed methods to the dynamic channel conditions.
MoreTranslated text
AI Read Science
Must-Reading Tree
Example
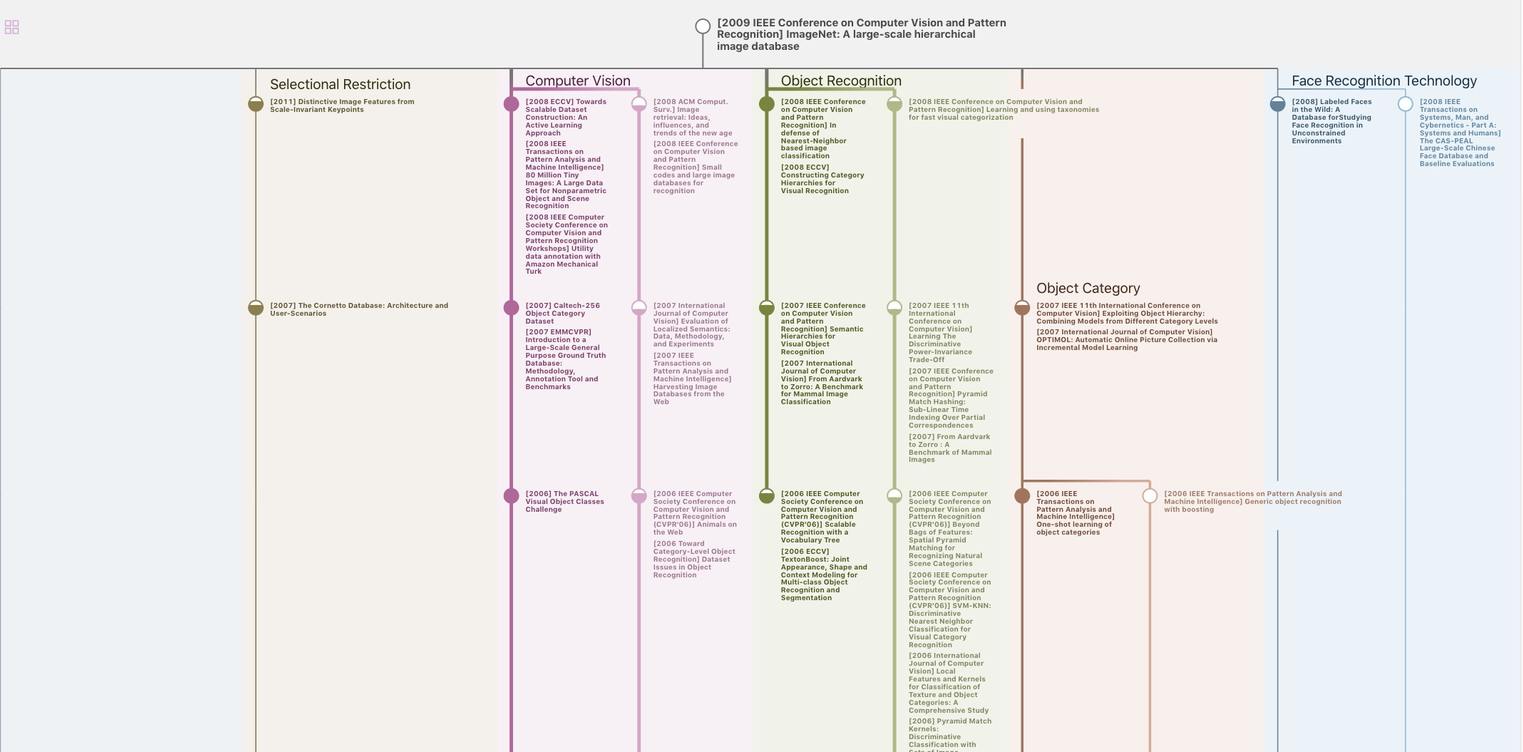
Generate MRT to find the research sequence of this paper
Chat Paper
Summary is being generated by the instructions you defined