Dual teachers for self-knowledge distillation
Pattern Recognition(2024)
摘要
We introduce an efficient self-knowledge distillation framework, Dual Teachers for Self-Knowledge Distillation (DTSKD), where the student receives self-supervisions by dual teachers from two substantially different fields, i.e., the past learning history and the current network structure. Specifically, DTSKD trains a considerably lightweight multi-branch network and acquires predictions from each, which are simultaneously supervised by a historical teacher from the previous epoch and a structural teacher under the current iteration. To our best knowledge, it is the first attempt to jointly conduct historical and structural self-knowledge distillation in a unified framework where they demonstrate complementary and mutual benefits. The Mixed Fusion Module (MFM) is further developed to bridge the semantic gap between deep stages and shallow branches by iteratively fusing multi-stage features based on the top-down topology. Extensive experiments prove the effectiveness of our proposed method, showing superior performance over related state-of-the-art self-distillation works on three datasets: CIFAR-100, ImageNet-2012, and PASCAL VOC.
更多查看译文
关键词
Model compression,Image classification,Self-knowledge distillation,Dual teachers
AI 理解论文
溯源树
样例
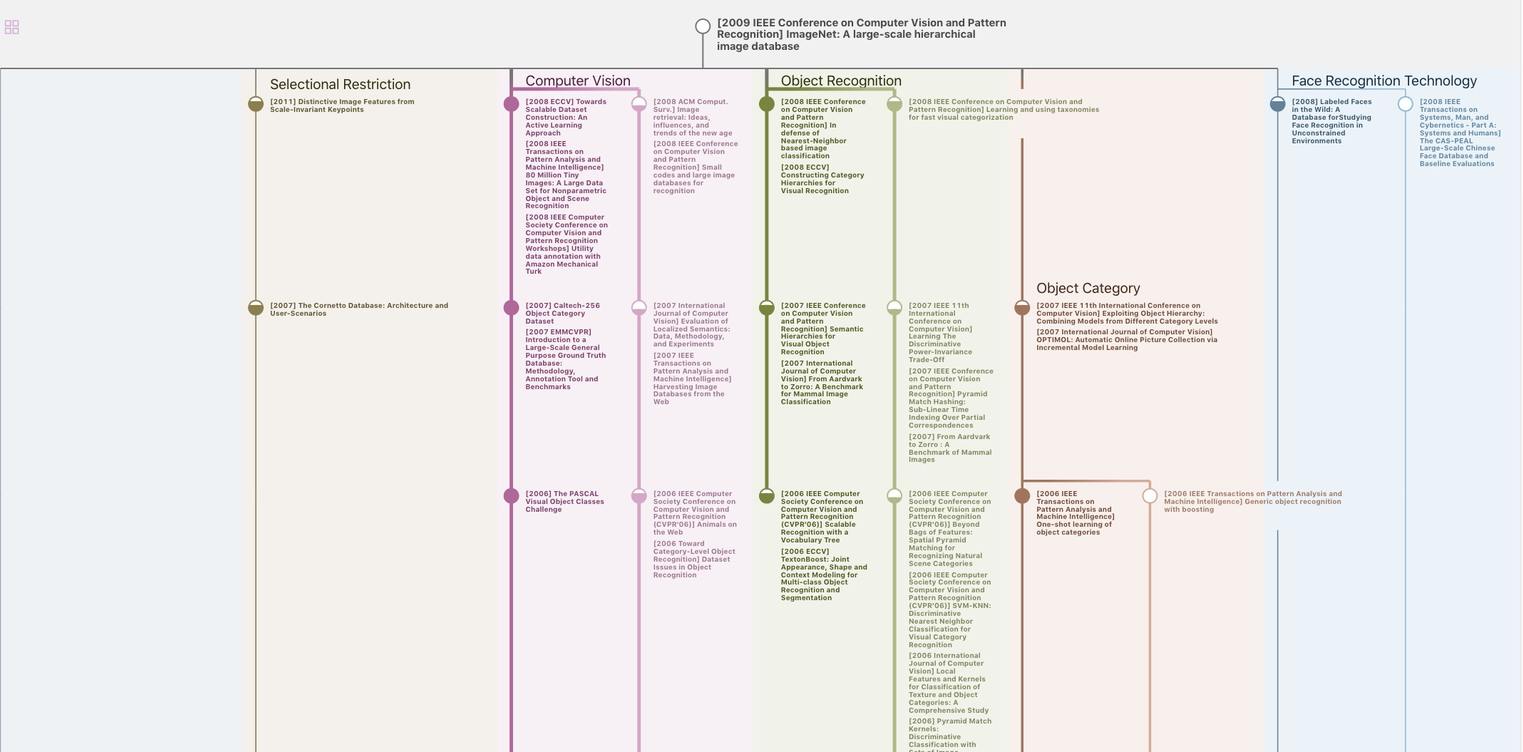
生成溯源树,研究论文发展脉络
Chat Paper
正在生成论文摘要