Improving performance prediction of metal-organic framework membranes for reverse osmosis via genetic algorithm optimized artificial neural networks
MATERIALS TODAY SUSTAINABILITY(2024)
摘要
The pollution of water resources and the rapid development of society are leading to the decrease of available fresh water resources in the world, which intensifies the research on seawater desalination. Metal-organic frameworks (MOFs), as promising membrane materials with diversified sizes, highly porous structures, and tunable chemical functionalities, have been extensively studied for desalination in recent years. In this paper, genetic algorithm (GA) optimized back propagation (BP) artificial neural network was first constructed, which made the prediction model of MOF membranes performances more accurate. Before the GA optimization, the structure of BP model was tuned through cross-validation and hyperparameter adjustment to achieve a better prediction performance and robustness. Then the GA was introduced attaining an enhanced R2 of 0.98 and 0.99 compared to the BP model of 0.90 and 0.97 for water permeability and salt rejection, respectively, which was also superior to the random forest, support vector machine, and multiple linear regression models. In addition, the grey relational analysis was used to screen the feature variables in order to clarify the most influential factors that affect the membrane performances. It is found that the MOF size and the thickness of polyamide layer were the key influential factors. This work will provide insights for the design of high-performance MOF based membranes and further render implications for other membrane developments.
更多查看译文
关键词
Metal-organic framework,Membrane,Permeability,Salt rejection,Machine learning,Grey relational analysis
AI 理解论文
溯源树
样例
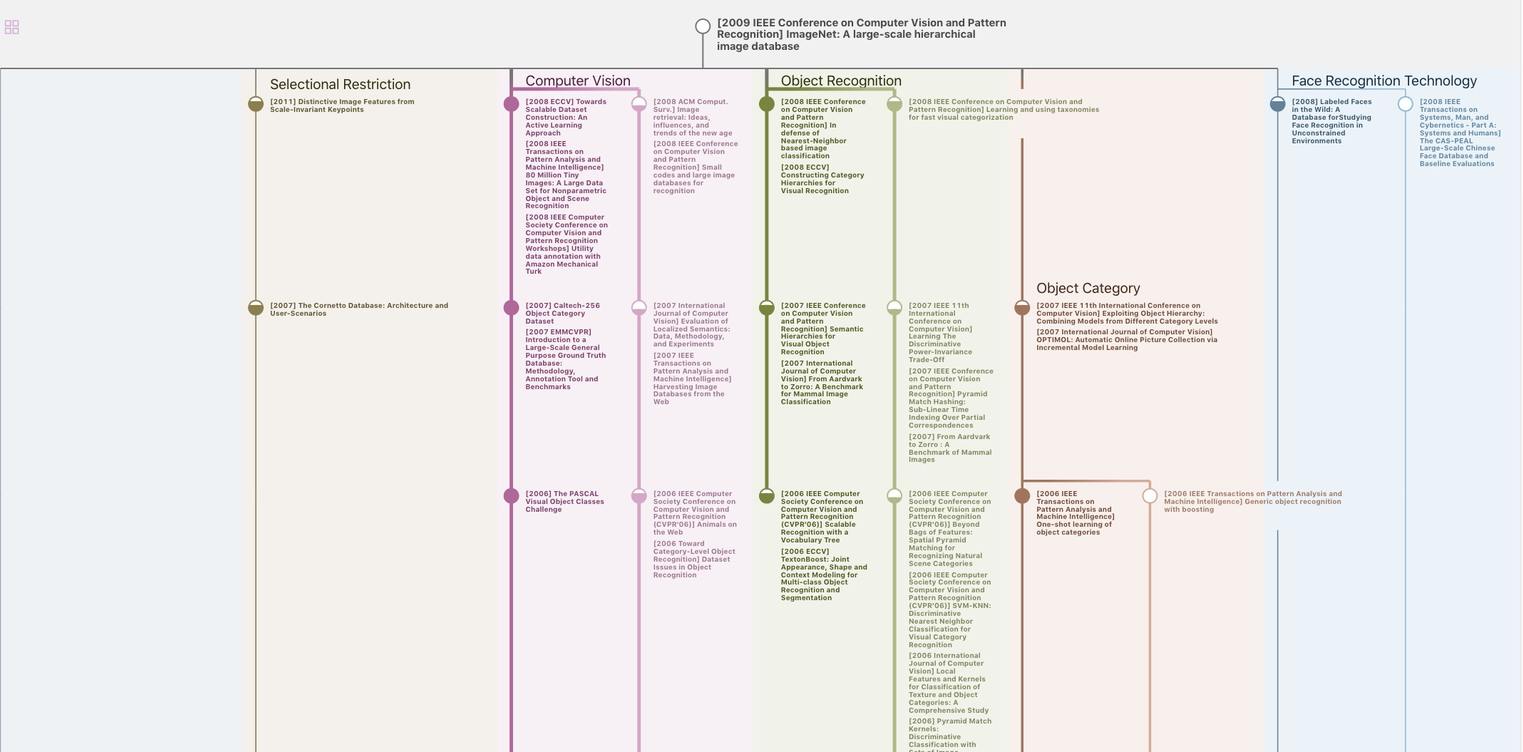
生成溯源树,研究论文发展脉络
Chat Paper
正在生成论文摘要