Low-carbon embodied alkali-activated materials for sustainable construction: A comparative study of single and ensemble learners
REVIEWS ON ADVANCED MATERIALS SCIENCE(2024)
摘要
Popular and eco-friendly alkali-activated materials (AAMs) replace Portland cement concrete. Due to the considerable compositional variability of AAMs and the inability of established materials science methods to understand composition-performance relationships, accurate property forecasts have proved impossible. This study set out to develop AAM compressive strength (CS) evaluation machine learning (ML) models using techniques including extreme gradient boosting (XGB), bagging regressor (BR), and multi-layer perceptron neural network (MLPNN). Ten input variables were used with a large dataset of 676 points. Statistical and K-fold studies were also used to evaluate the developed models' correctness. XGB predicted the CS of AAM the best, followed by BR and MLPNN. The MLPNN and BR models had R-2 values of 0.80 and 0.90, respectively, whereas the XGB model had 0.94. Results from statistical analyses and k-fold cross-validation of the used ML models further attest to their validity. The built models can potentially compute the CS of AAMs for a variety of input parameter values, reducing the requirement for costly and time-consuming laboratory testing. Researchers and businesses may find this study useful in determining the necessary quantities of AAMs' raw components.
更多查看译文
关键词
alkali-activated materials,machine learning,compressive strength
AI 理解论文
溯源树
样例
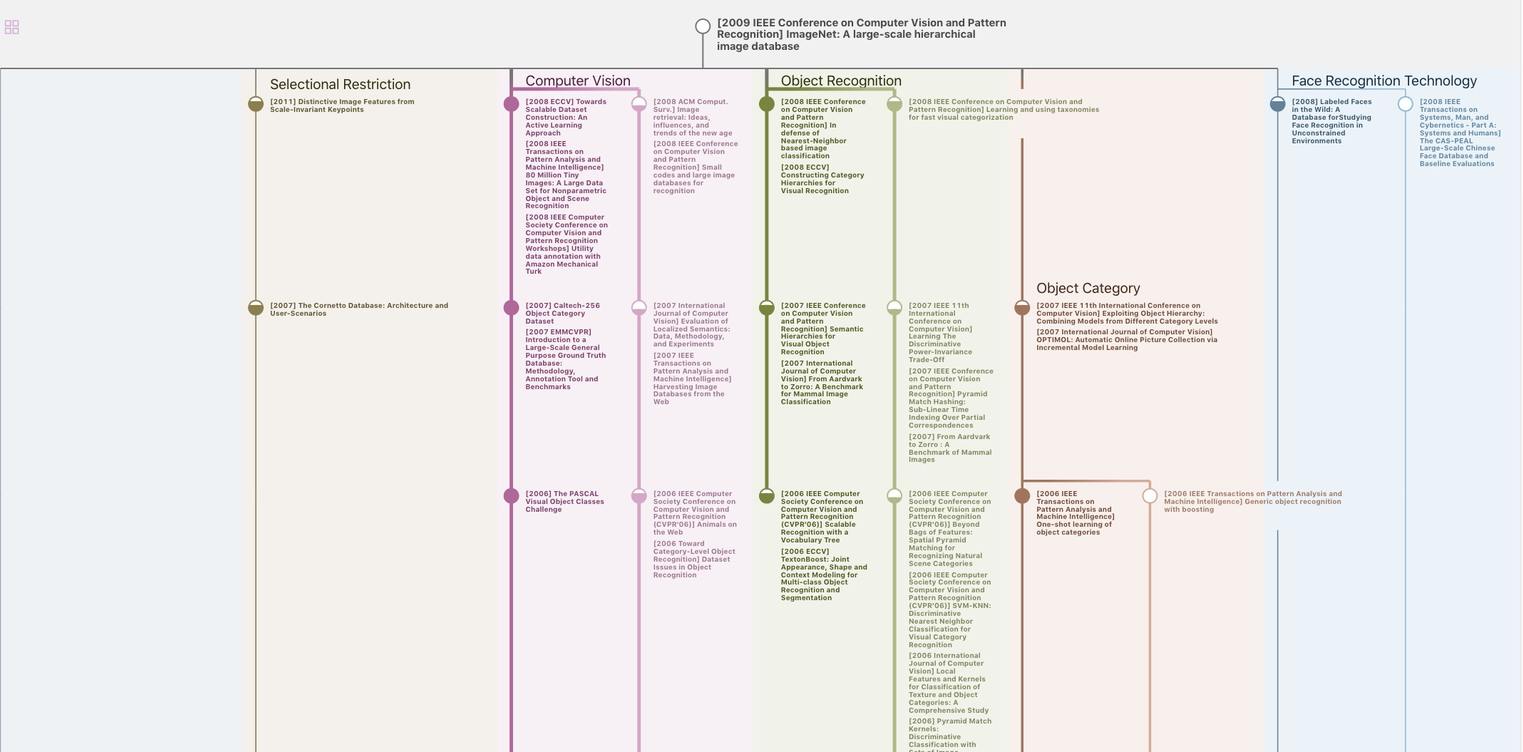
生成溯源树,研究论文发展脉络
Chat Paper
正在生成论文摘要