Decoding phenotypic screening: A comparative analysis of image representations
COMPUTATIONAL AND STRUCTURAL BIOTECHNOLOGY JOURNAL(2024)
摘要
Biomedical imaging techniques such as high content screening (HCS) are valuable for drug discovery, but high costs limit their use to pharmaceutical companies. To address this issue, The JUMP-CP consortium released a massive open image dataset of chemical and genetic perturbations, providing a valuable resource for deep learning research. In this work, we aim to utilize the JUMP-CP dataset to develop a universal representation model for HCS data, mainly data generated using U2OS cells and CellPainting protocol, using supervised and selfsupervised learning approaches. We propose an evaluation protocol that assesses their performance on mode of action and property prediction tasks using a popular phenotypic screening dataset. Results show that the selfsupervised approach that uses data from multiple consortium partners provides representation that is more robust to batch effects whilst simultaneously achieving performance on par with standard approaches. Together with other conclusions, it provides recommendations on the training strategy of a representation model for HCS images.
更多查看译文
关键词
High Content Screening,Self-supervised learning,Image representation,Deep Learning,Activity prediction
AI 理解论文
溯源树
样例
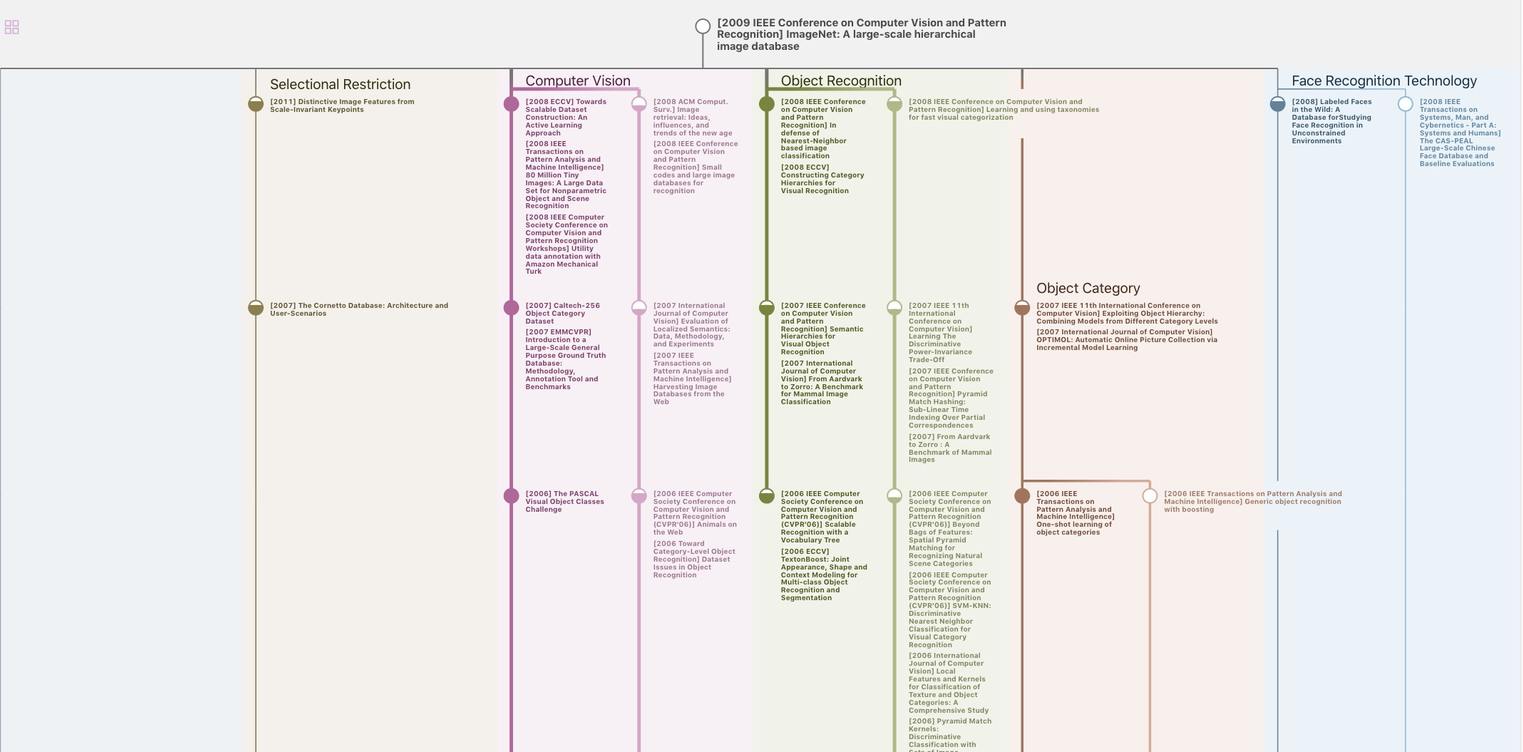
生成溯源树,研究论文发展脉络
Chat Paper
正在生成论文摘要