Adaptive Multiscale Reversible Column Network for SAR Ship Detection
IEEE Journal of Selected Topics in Applied Earth Observations and Remote Sensing(2024)
摘要
Ship detection via synthetic aperture radar (SAR) is widely used in maritime safety and maritime traffic control, etc. Recently, deep learning methods are employed to improve the performance of SAR ship detection to a large extent. However, the presence of clutter in SAR images and the large-scale difference of ships result in the diminished detection performance in complex environments. As such, in this article, a novel adaptive multiscale reversible column network is proposed. First, the idea of disentangled feature learning is applied to construct reversible column networks with a C2f module to alleviate the problem of large-scale differences and the loss of ship information. Second, the multiplexed adaptive spatial pyramid pooling module is proposed to alleviate the impact of complex background clutter through multiplexed pooling operations and adaptive fusion. Finally, a novel feature pyramid network with an adaptive downsampling module is designed to reduce the information loss caused by downsampling while enhancing the multiscale ship detection capability. The effectiveness of the proposed method is validated on two public datasets: 1) SAR ship detection dataset and 2) high-resolution SAR images dataset. The experimental results show that the proposed method is able to achieve better results than current state-of-the-art methods for SAR ship detection in complex environments and large-scale differences of ships.
更多查看译文
关键词
Adaptive downsampling,feature extraction,reversible column network,ship detection,synthetic aperture radar (SAR)
AI 理解论文
溯源树
样例
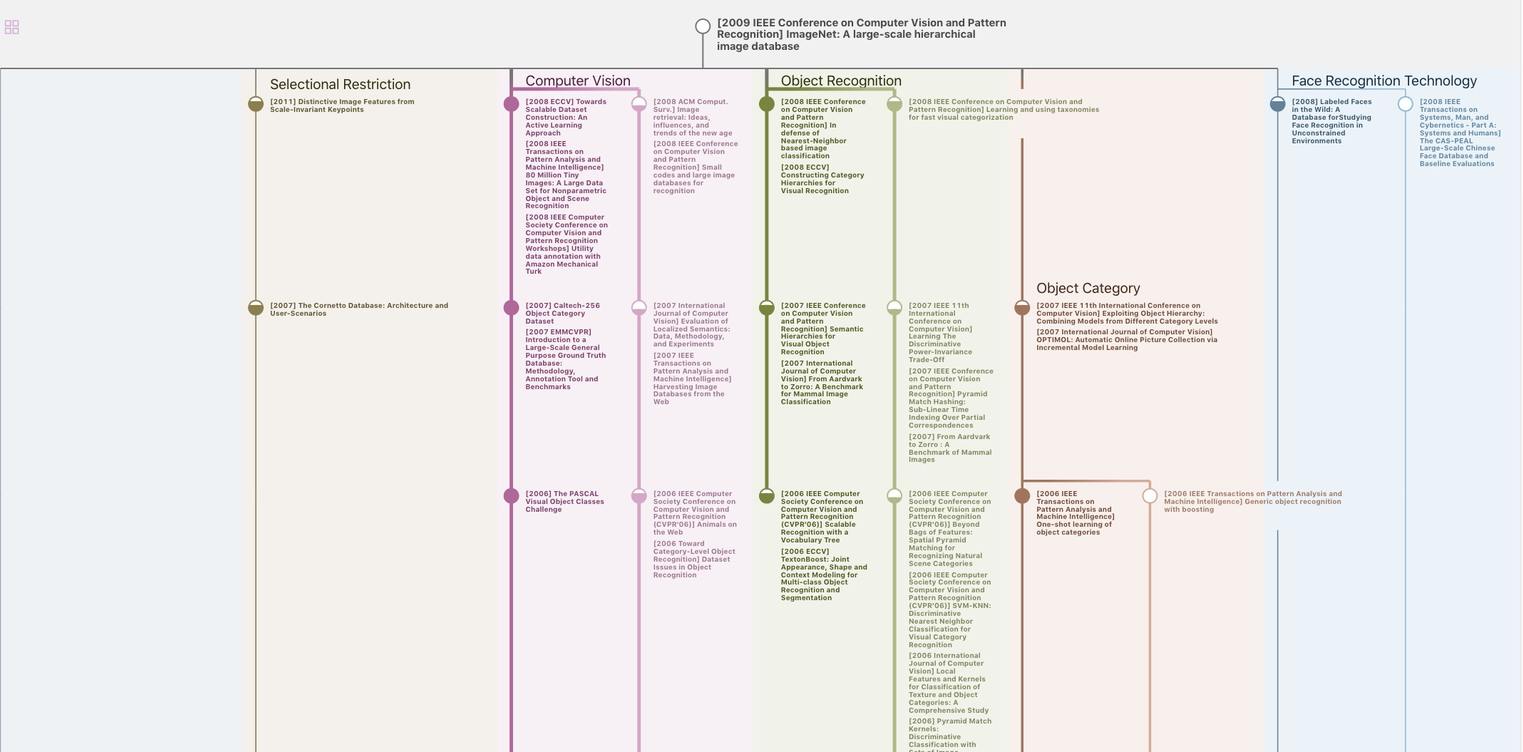
生成溯源树,研究论文发展脉络
Chat Paper
正在生成论文摘要