MSAMS-Net: accurate lung lesion segmentation from COVID-19 CT images
Multimedia Tools and Applications(2024)
摘要
The coronavirus disease 2019 (COVID-19) has emerged as a global pandemic, inflicting significant harm on the health of humans. A crucial objective in combating this disease is the development of a precise and effective technique for automatically segmenting lung lesions, which can help reduce the risk of severe complications. However, lesion segmentation of COVID-19 from CT slices presents numerous challenges. These challenges include the small size of the lesions and the blurred boundaries observed during the early stages of the disease. Additionally, the limited availability of medical image datasets and the considerable variation in symptoms among patients pose significant tests for the generalization ability of the model. In this paper, we develop a novel Multi-path Shuffle Attention module with a master-slave encoder and decoder network (MSAMS-Net) for lesion segmentation of COVID-19, especially those tiny lesions. In our proposed MSAMS-Net, an Efficient Multi-path Shuffle Attention module is presented to compensate for the lack of detail semantics when fusing over long distances. Moreover, we present a parallel attention module to capture both global and local information. To evaluate the performance of our model, we conduct experiments on four datasets which are collected from seven publicly available datasets. The results demonstrate that our proposed model outperforms other models in terms of Dice scores or Hausdorff Distance. Furthermore, the ablation study greatly proves the significance of the multi-path shuffle attention mechanism module. Overall, our proposed model exhibits superior performance compared to other state-of-the-art methods.
更多查看译文
关键词
COVID-19,CT slice,Attention mechanism,Multi-scale feature,Lesion segmentation,Multi-path structure
AI 理解论文
溯源树
样例
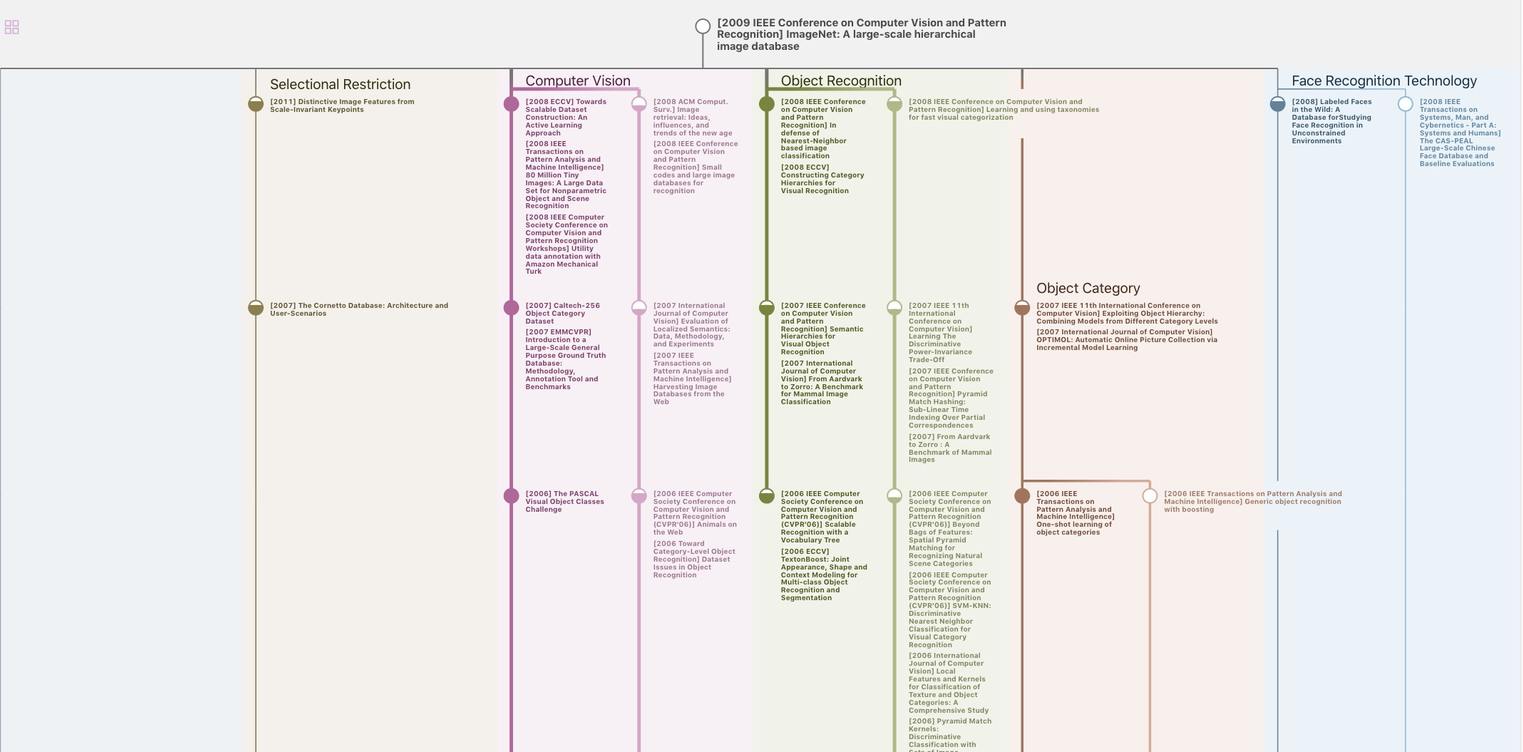
生成溯源树,研究论文发展脉络
Chat Paper
正在生成论文摘要