A Novel Quality Monitoring Approach Based on Multigranularity Spatiotemporal Attentive Representation Learning During Climbing GTAW
IEEE TRANSACTIONS ON INDUSTRIAL INFORMATICS(2024)
摘要
Reliable welding quality monitoring (WQM) is a long-standing challenge for climbing gas tungsten arc welding (GTAW) due to the inherent instability and complexity of the weld pool during upward welding, especially for the fabrication of large-scale structural components with medium-thick and thick aluminum plates. This article presents a novel WQM approach based on multigranularity spatiotemporal attentive representation learning, aiming to accurately characterize molten pool state and detect welding defects in real time. A passive vision sensing system is constructed to monitor the climbing GTAW process. A long-term dynamic information-enhanced multigranularity spatiotemporal attentive representation learning network is proposed. The network adopts a feature-level image fusion strategy and multigranularity attention mechanism to simultaneously aggregate discriminative information at different semantic levels on the temporal and spatial dimensions from a global view, while utilizing a bilateral branch structure to alleviate class imbalance in the data. Moreover, long-term dynamic information is mined from the molten pool time series images through motion edge history images. Experimental results show that the proposed approach has a remarkable classification performance and robustness compared with the typical comparison models even with class imbalance and noisy training data. This approach offers a promising new solution for WQM and is expected to be utilized to provide real-time feedback in a closed-loop quality control system.
更多查看译文
关键词
Climbing gas tungsten arc welding (GTAW),deep learning,multigranularity (MG) spatiotemporal attentive representation,vision sensing,welding quality monitoring (WQM)
AI 理解论文
溯源树
样例
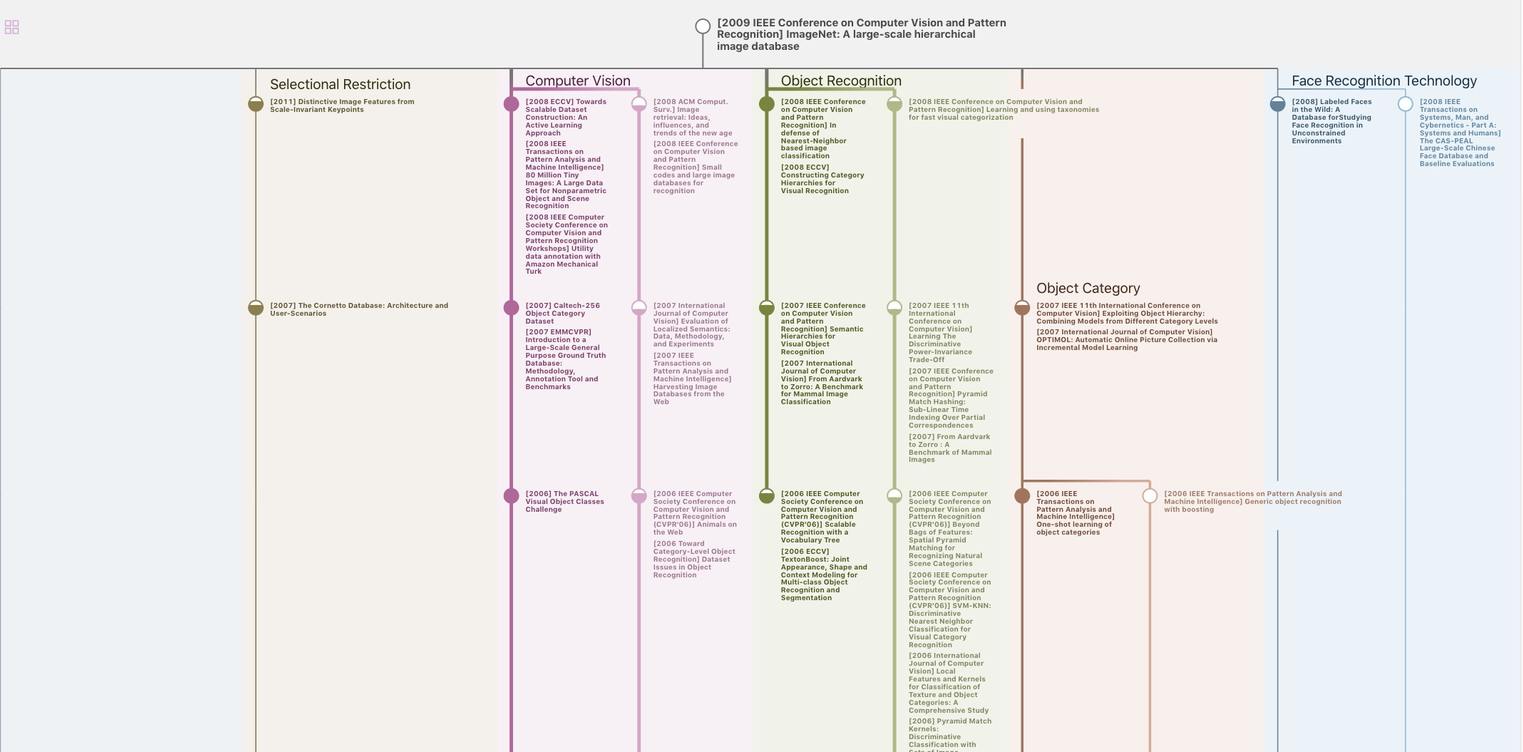
生成溯源树,研究论文发展脉络
Chat Paper
正在生成论文摘要