Fine-tuning Neural Network Quantum States
arxiv(2024)
摘要
Recent progress in the design and optimization of Neural Network Quantum
States (NNQS) have made them an effective method to investigate ground-state
properties of quantum many-body systems. In contrast to the standard approach
of training a separate NNQS from scratch at every point of the phase diagram,
we demonstrate that the optimization at a highly expressive point of the phase
diagram (i.e., close to a phase transition) yields interpretable features that
can be reused to accurately describe a wide region across the transition. We
demonstrate the feasibility of our approach on different systems in one and two
dimensions by initially pretraining a NNQS at a given point of the phase
diagram, followed by fine-tuning only the output layer for all other points.
Notably, the computational cost of the fine-tuning step is very low compared to
the pretraining stage. We argue that the reduced cost of this paradigm has
significant potential to advance the exploration of condensed matter systems
using NNQS, mirroring the success of fine-tuning in machine learning and
natural language processing.
更多查看译文
AI 理解论文
溯源树
样例
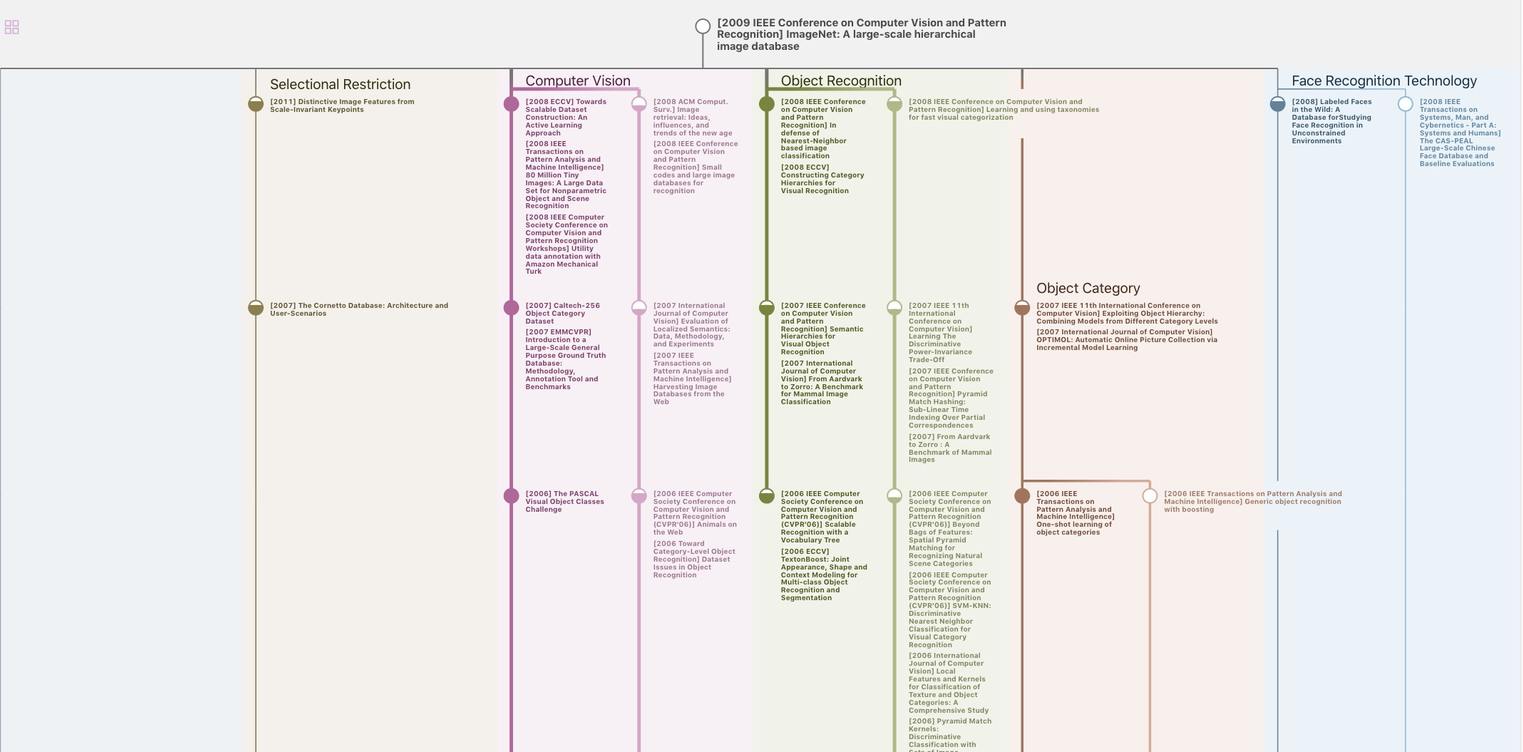
生成溯源树,研究论文发展脉络
Chat Paper
正在生成论文摘要