Intra-video Positive Pairs in Self-Supervised Learning for Ultrasound
CoRR(2024)
摘要
Self-supervised learning (SSL) is one strategy for addressing the paucity of
labelled data in medical imaging by learning representations from unlabelled
images. Contrastive and non-contrastive SSL methods produce learned
representations that are similar for pairs of related images. Such pairs are
commonly constructed by randomly distorting the same image twice. The
videographic nature of ultrasound offers flexibility for defining the
similarity relationship between pairs of images. In this study, we investigated
the effect of utilizing proximal, distinct images from the same B-mode
ultrasound video as pairs for SSL. Additionally, we introduced a sample
weighting scheme that increases the weight of closer image pairs and
demonstrated how it can be integrated into SSL objectives. Named Intra-Video
Positive Pairs (IVPP), the method surpassed previous ultrasound-specific
contrastive learning methods' average test accuracy on COVID-19 classification
with the POCUS dataset by ≥ 1.3%. Detailed investigations of IVPP's
hyperparameters revealed that some combinations of IVPP hyperparameters can
lead to improved or worsened performance, depending on the downstream task.
Guidelines for practitioners were synthesized based on the results, such as the
merit of IVPP with task-specific hyperparameters, and the improved performance
of contrastive methods for ultrasound compared to non-contrastive counterparts.
更多查看译文
关键词
self-supervised learning,ultrasound,contrastive learning,non-contrastive learning,representation learning
AI 理解论文
溯源树
样例
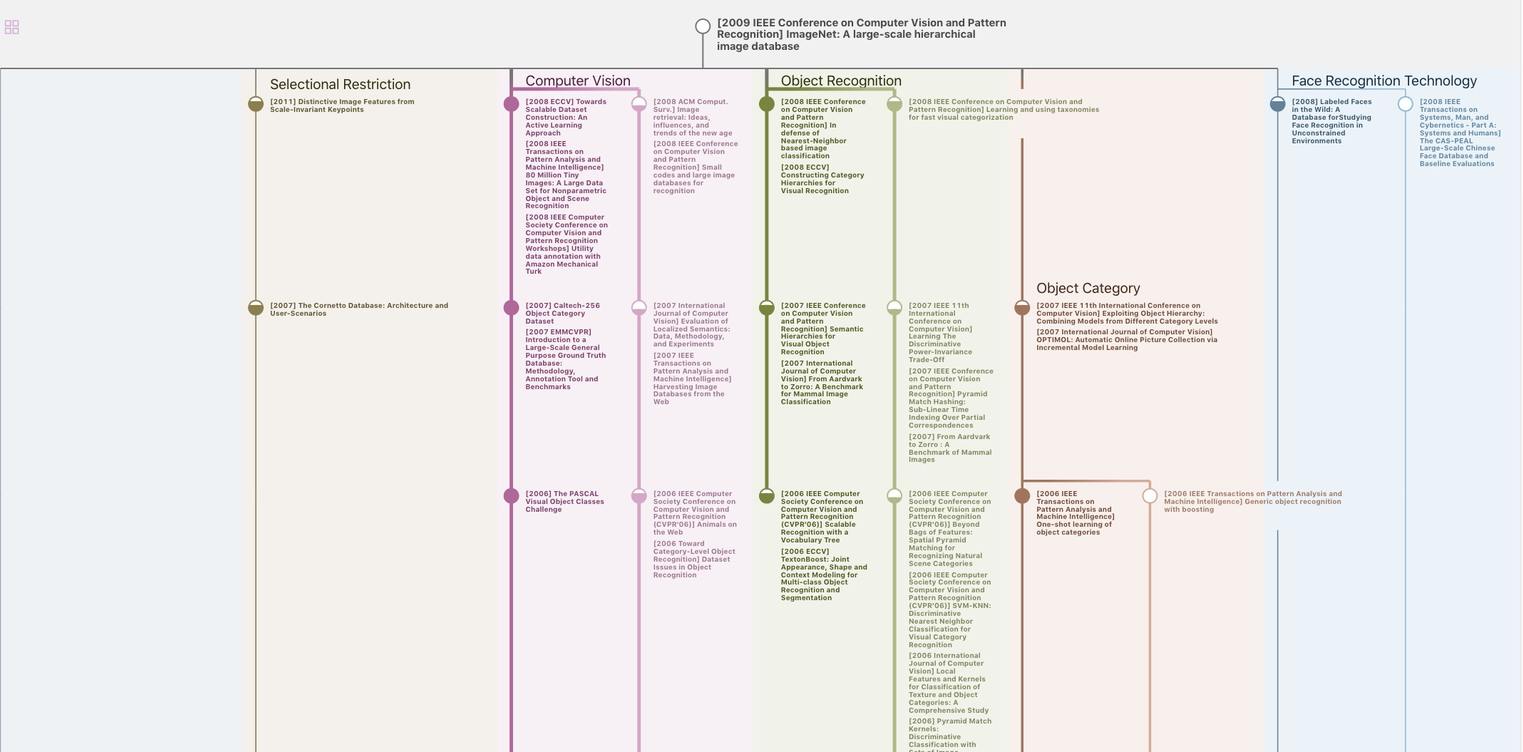
生成溯源树,研究论文发展脉络
Chat Paper
正在生成论文摘要