Harder Tasks Need More Experts: Dynamic Routing in MoE Models
arxiv(2024)
摘要
In this paper, we introduce a novel dynamic expert selection framework for
Mixture of Experts (MoE) models, aiming to enhance computational efficiency and
model performance by adjusting the number of activated experts based on input
difficulty. Unlike traditional MoE approaches that rely on fixed Top-K routing,
which activates a predetermined number of experts regardless of the input's
complexity, our method dynamically selects experts based on the confidence
level in expert selection for each input. This allows for a more efficient
utilization of computational resources, activating more experts for complex
tasks requiring advanced reasoning and fewer for simpler tasks. Through
extensive evaluations, our dynamic routing method demonstrates substantial
improvements over conventional Top-2 routing across various benchmarks,
achieving an average improvement of 0.7
parameters. Further analysis shows our model dispatches more experts to tasks
requiring complex reasoning skills, like BBH, confirming its ability to
dynamically allocate computational resources in alignment with the input's
complexity. Our findings also highlight a variation in the number of experts
needed across different layers of the transformer model, offering insights into
the potential for designing heterogeneous MoE frameworks. The code and models
are available at https://github.com/ZhenweiAn/Dynamic_MoE.
更多查看译文
AI 理解论文
溯源树
样例
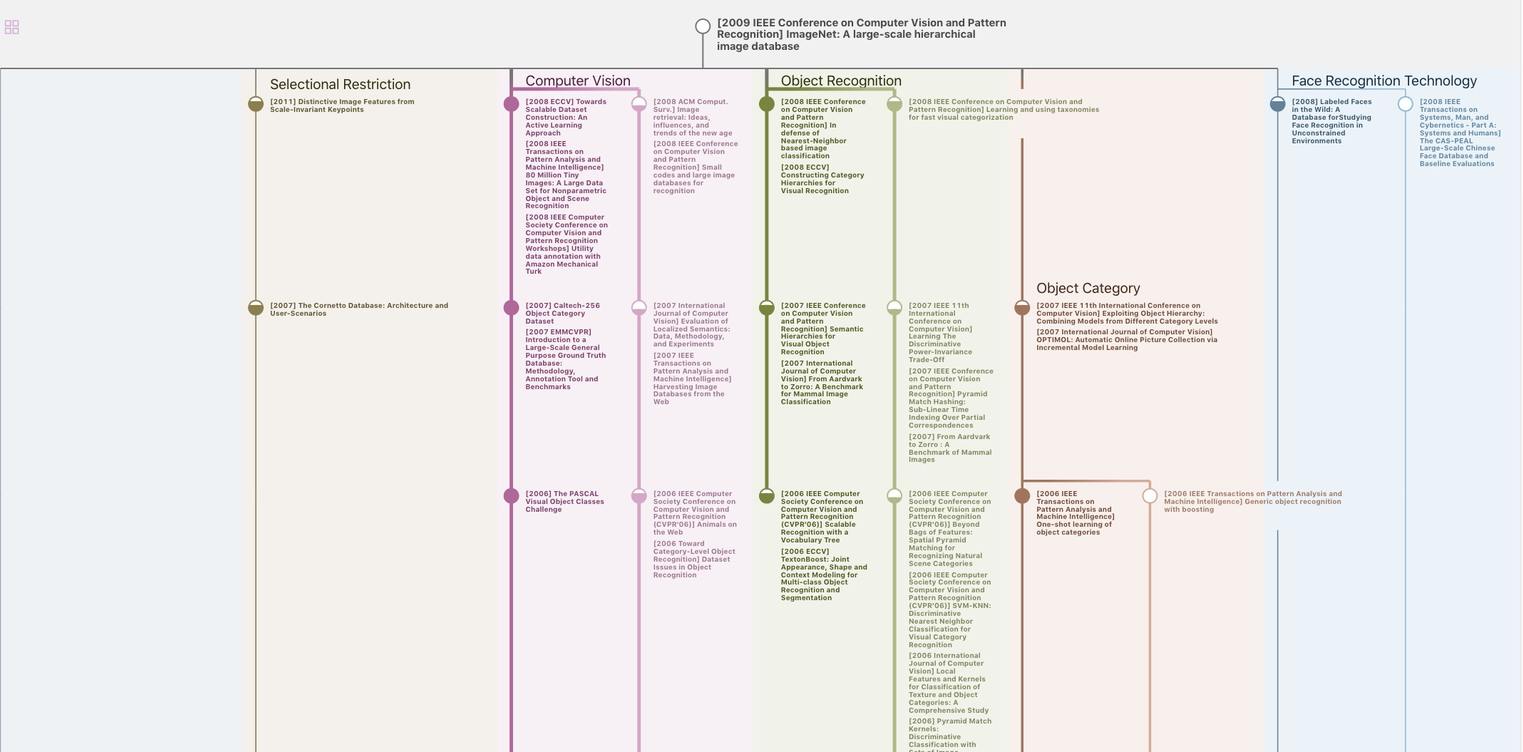
生成溯源树,研究论文发展脉络
Chat Paper
正在生成论文摘要